Locally Private Streaming Data Release with Shuffling and Subsampling
2023 IEEE 39TH INTERNATIONAL CONFERENCE ON DATA ENGINEERING WORKSHOPS, ICDEW(2023)
摘要
Longitudinal data collection is an important task for real-time data analysts in this Big Data Era. However, the continual observation of raw data may leak user's sensitive information. Local differential privacy is a rigorous privacy-preserving technique for statistical data release without a trusted server, but at the cost of low utility. The recently proposed shuffle model of differential privacy has the potential to preserve local differential privacy with high utility by its privacy amplification effect; however, even under the shuffle model, the utility may not be satisfactory when data are collected continuously because the privacy budget needs to be allocated to every time points. In this paper, we make three contributions to address this problem. First, we propose a simple yet effective subsampling scheme to enhance the utility of the shuffle model for private streaming data release. Intuitively, only a portion of users will be sampled to participate in the data analysis at each time point; hence, we can obtain sufficient utility even under continual data collection. Second, we prove that our algorithm with shuffling and subsampling enjoys double privacy amplification, which means a better privacy-utility trade-off than the vanilla shuffle model. Third, we observe an interesting relationship between the number of sampled users and utility: as the sample rate increase, utility first increases and then decreases. Inspired by this, we provided theoretical analysis on choosing the optimal sample rate and verified its effectiveness in our preliminary experiments.
更多查看译文
AI 理解论文
溯源树
样例
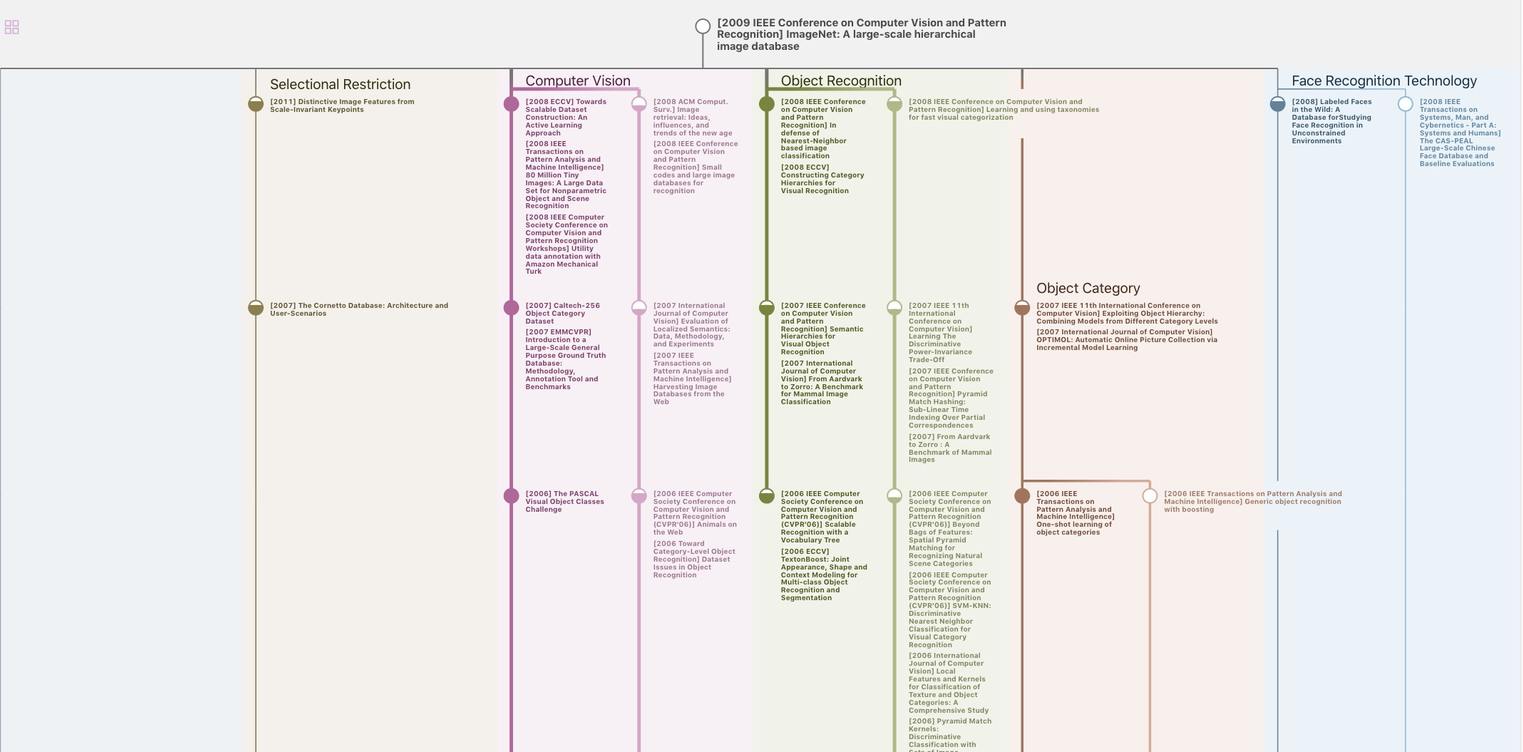
生成溯源树,研究论文发展脉络
Chat Paper
正在生成论文摘要