Theoretical Foundations of Adversarially Robust Learning
CoRR(2023)
摘要
Despite extraordinary progress, current machine learning systems have been shown to be brittle against adversarial examples: seemingly innocuous but carefully crafted perturbations of test examples that cause machine learning predictors to misclassify. Can we learn predictors robust to adversarial examples? and how? There has been much empirical interest in this contemporary challenge in machine learning, and in this thesis, we address it from a theoretical perspective. In this thesis, we explore what robustness properties can we hope to guarantee against adversarial examples and develop an understanding of how to algorithmically guarantee them. We illustrate the need to go beyond traditional approaches and principles such as empirical risk minimization and uniform convergence, and make contributions that can be categorized as follows: (1) introducing problem formulations capturing aspects of emerging practical challenges in robust learning, (2) designing new learning algorithms with provable robustness guarantees, and (3) characterizing the complexity of robust learning and fundamental limitations on the performance of any algorithm.
更多查看译文
关键词
adversarially robust learning,theoretical foundations
AI 理解论文
溯源树
样例
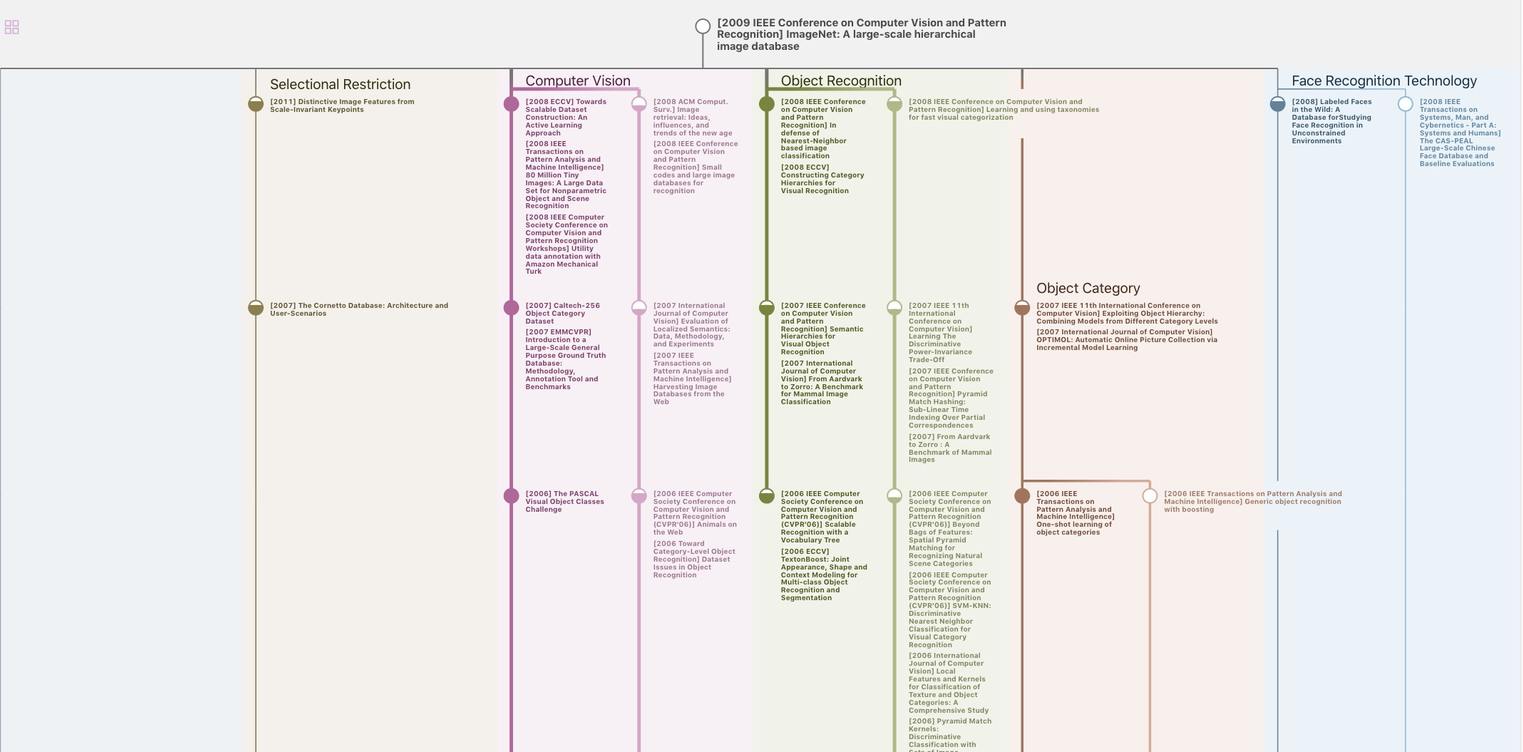
生成溯源树,研究论文发展脉络
Chat Paper
正在生成论文摘要