User-defined Event Sampling and Uncertainty Quantification in Diffusion Models for Physical Dynamical Systems
CoRR(2023)
摘要
Diffusion models are a class of probabilistic generative models that have been widely used as a prior for image processing tasks like text conditional generation and inpainting. We demonstrate that these models can be adapted to make predictions and provide uncertainty quantification for chaotic dynamical systems. In these applications, diffusion models can implicitly represent knowledge about outliers and extreme events; however, querying that knowledge through conditional sampling or measuring probabilities is surprisingly difficult. Existing methods for conditional sampling at inference time seek mainly to enforce the constraints, which is insufficient to match the statistics of the distribution or compute the probability of the chosen events. To achieve these ends, optimally one would use the conditional score function, but its computation is typically intractable. In this work, we develop a probabilistic approximation scheme for the conditional score function which provably converges to the true distribution as the noise level decreases. With this scheme we are able to sample conditionally on nonlinear userdefined events at inference time, and matches data statistics even when sampling from the tails of the distribution.
更多查看译文
关键词
event sampling,uncertainty quantification,diffusion
AI 理解论文
溯源树
样例
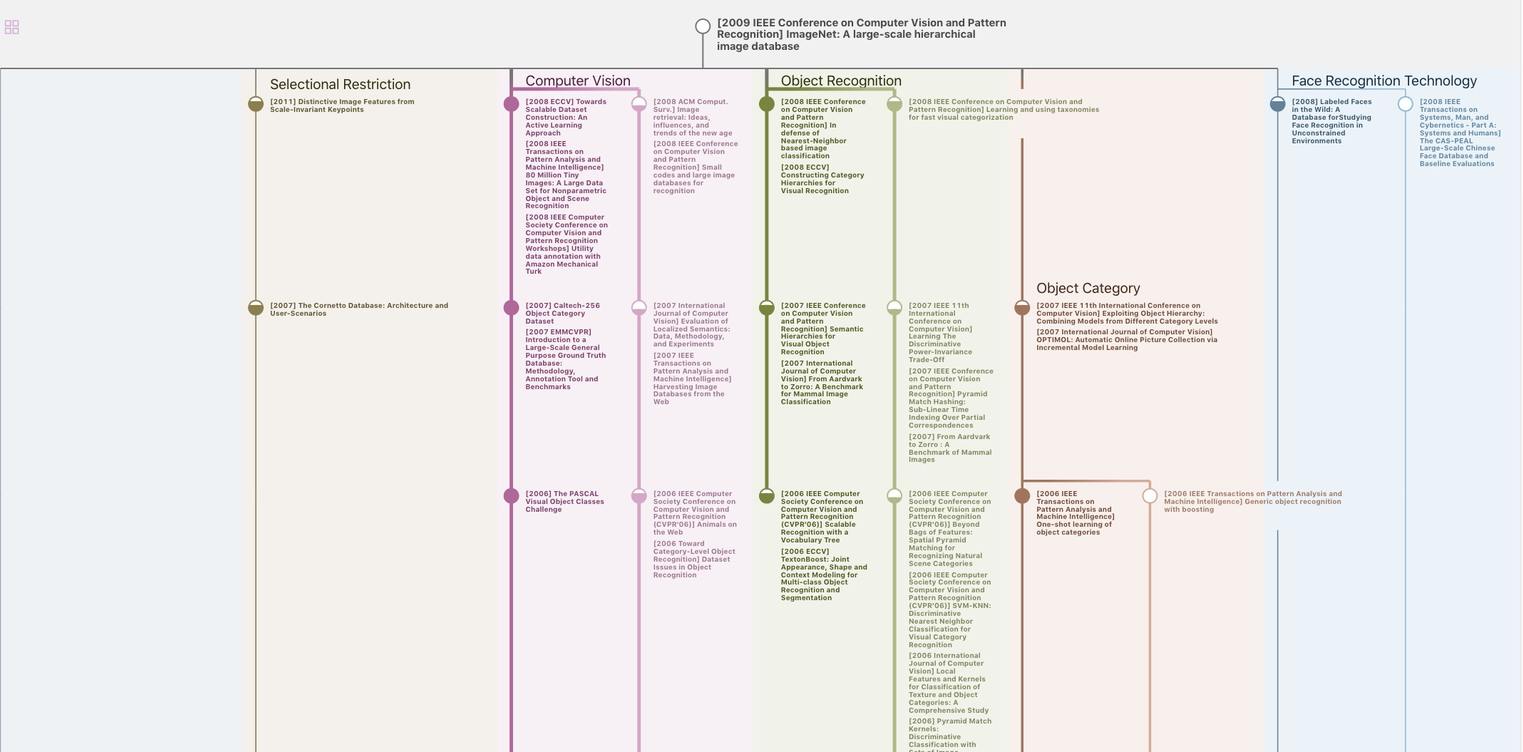
生成溯源树,研究论文发展脉络
Chat Paper
正在生成论文摘要