Budgeted Multi-Armed Bandits with Asymmetric Confidence Intervals
CoRR(2023)
摘要
We study the stochastic Budgeted Multi-Armed Bandit (MAB) problem, where a player chooses from $K$ arms with unknown expected rewards and costs. The goal is to maximize the total reward under a budget constraint. A player thus seeks to choose the arm with the highest reward-cost ratio as often as possible. Current state-of-the-art policies for this problem have several issues, which we illustrate. To overcome them, we propose a new upper confidence bound (UCB) sampling policy, $\omega$-UCB, that uses asymmetric confidence intervals. These intervals scale with the distance between the sample mean and the bounds of a random variable, yielding a more accurate and tight estimation of the reward-cost ratio compared to our competitors. We show that our approach has logarithmic regret and consistently outperforms existing policies in synthetic and real settings.
更多查看译文
关键词
asymmetric confidence intervals,confidence intervals,multi-armed
AI 理解论文
溯源树
样例
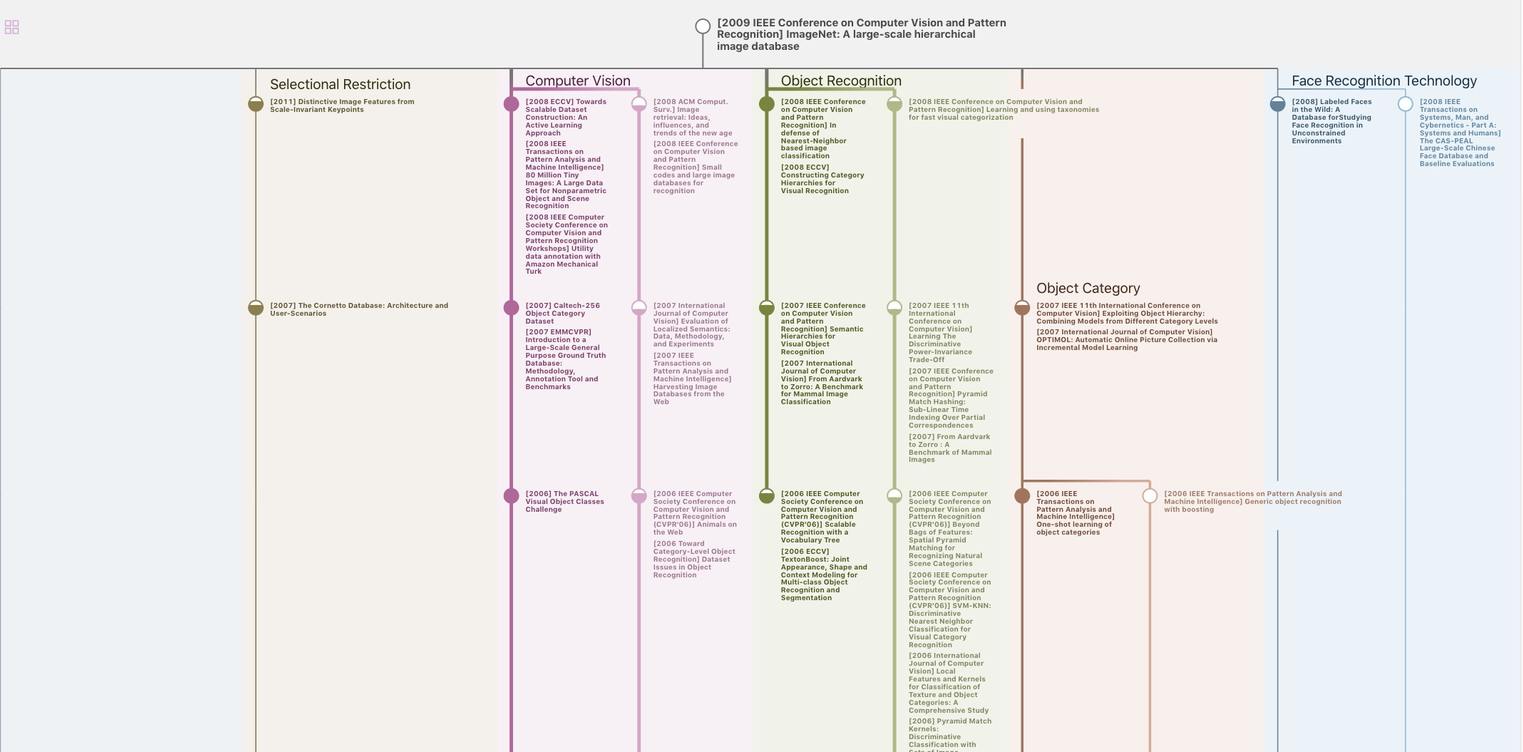
生成溯源树,研究论文发展脉络
Chat Paper
正在生成论文摘要