Rethinking Translation Memory Augmented Neural Machine Translation
conf_acl(2023)
摘要
This paper rethinks translation memory augmented neural machine translation (TM-augmented NMT) from two perspectives, i.e., a probabilistic view of retrieval and the variance-bias decomposition principle. The finding demonstrates that TM-augmented NMT is good at the ability of fitting data (i.e., lower bias) but is more sensitive to the fluctuations in the training data (i.e., higher variance), which provides an explanation to a recently reported contradictory phenomenon on the same translation task: TM-augmented NMT substantially advances vanilla NMT under the high-resource scenario whereas it fails under the low-resource scenario. Then we propose a simple yet effective TM-augmented NMT model to promote the variance and address the contradictory phenomenon. Extensive experiments show that the proposed TM-augmented NMT achieves consistent gains over both conventional NMT and existing TM-augmented NMT under two variance-preferable (low-resource and plug-and-play) scenarios as well as the high-resource scenario.
更多查看译文
关键词
neural machine translation,memory
AI 理解论文
溯源树
样例
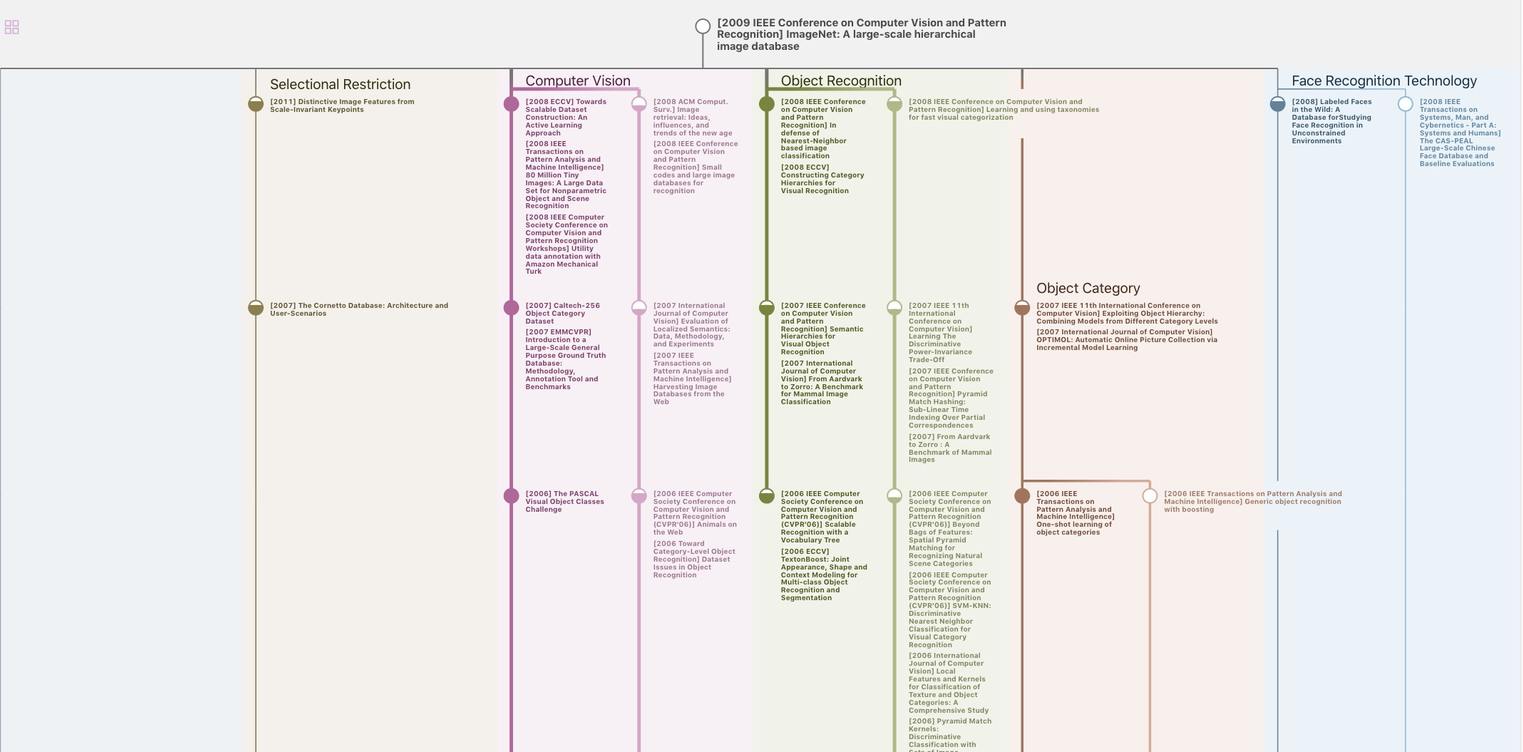
生成溯源树,研究论文发展脉络
Chat Paper
正在生成论文摘要