INK: Injecting kNN Knowledge in Nearest Neighbor Machine Translation
conf_acl(2023)
摘要
Neural machine translation has achieved promising results on many translation tasks. However, previous studies have shown that neural models induce a non-smooth representation space, which harms its generalization results. Recently, kNN-MT has provided an effective paradigm to smooth the prediction based on neighbor representations during inference. Despite promising results, kNN-MT usually requires large inference overhead. We propose an effective training framework INK to directly smooth the representation space via adjusting representations of kNN neighbors with a small number of new parameters. The new parameters are then used to refresh the whole representation datastore to get new kNN knowledge asynchronously. This loop keeps running until convergence. Experiments on four benchmark datasets show that \method achieves average gains of 1.99 COMET and 1.0 BLEU, outperforming the state-of-the-art kNN-MT system with 0.02x memory space and 1.9x inference speedup.
更多查看译文
关键词
nearest neighbor machine translation,knowledge
AI 理解论文
溯源树
样例
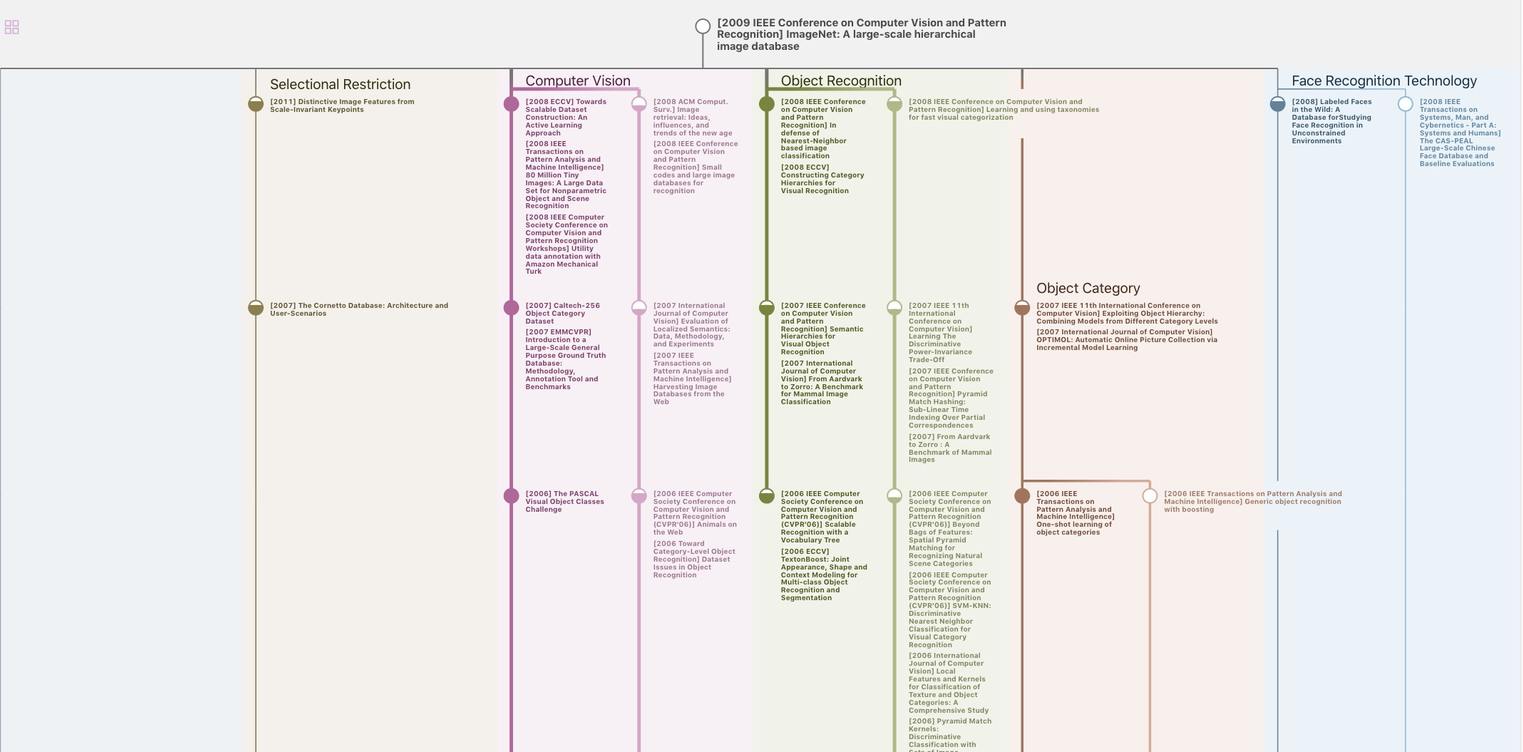
生成溯源树,研究论文发展脉络
Chat Paper
正在生成论文摘要