Split: A Hash-Based Memory Optimization Method for Zero-Knowledge Succinct Non-Interactive Argument of Knowledge (zk-SNARK)
IEEE Transactions on Computers(2023)
摘要
Zero-Knowledge Succinct Non-Interactive Argument of Knowledge (zk-SNARK) is a practical zero-knowledge proof system for Rank-1 Constraint Satisfaction (R1CS), enabling privacy preservation and addressing the previous scalability concerns on zero-knowledge proofs. Existing constructions of zk-SNARKs require huge memory overhead to generate proofs in that the size of the zk-SNARK circuit can be large even for a very simple use case, which limits the applications for regular resource-constrained users. To reduce the memory utilization of zk-SNARKs, this paper presents a hash-based method “Split”. Concretely, Split intends to partition the zk-SNARK circuits so that components can be processed sequentially while ensuring strong security properties leveraging hash circuits. As a zk-SNARK circuit is partitioned, obsolete variables are no longer preserved in the memory. We further propose an enhanced Split as
$n$
-Split, which leads to better optimization by properly choosing multiple splits. Our experimental results validate the effectiveness and efficiency of Split in conserving memory usage for resource-constrained provers as long as the circuit can be partitioned to a Good Split, indicating that via Split zk-SNARKs can be brought one step closer to practical applications.
更多查看译文
关键词
memory optimization method,hash-based,zero-knowledge,non-interactive,zk-snark
AI 理解论文
溯源树
样例
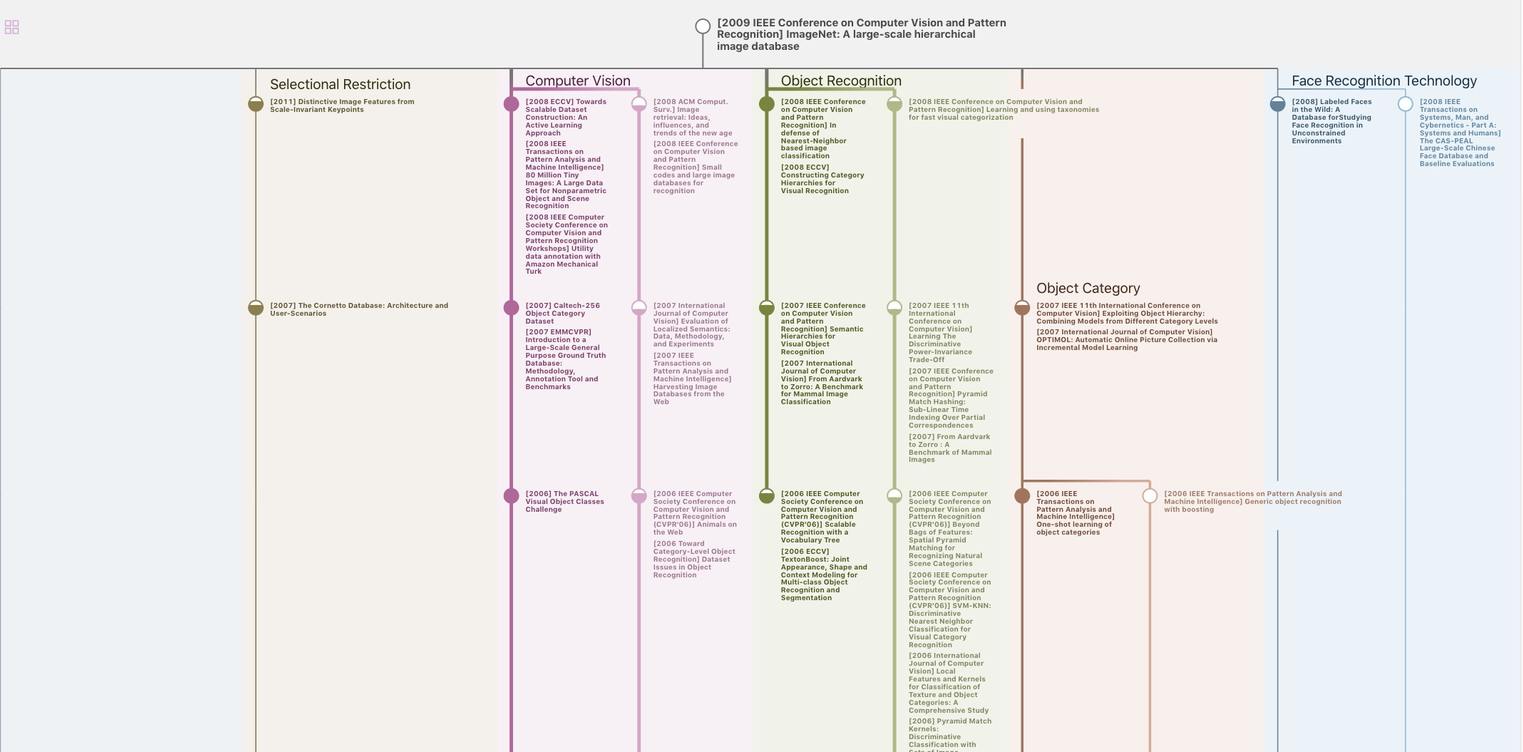
生成溯源树,研究论文发展脉络
Chat Paper
正在生成论文摘要