KeyState: Improving Image-based Reinforcement Learning with Keypoint for Robot Control
2023 IEEE International Conference on Industrial Technology (ICIT)(2023)
摘要
Learning from high-dimensional images is essential for reinforcement learning (RL) to train autonomous agents that can interact directly with the environment using visual observations. A general strategy is to extract task-relevant information in the images to learn a representation that characterizes the system states. Although existing works can find good representations for static states efficiently, they still take a long time to learn system dynamics, which is critical for applications such as robot control. Since the most important system dynamics in robot control is the motions of the robot itself, image-based RL can be more efficient if the state of the robot is known. In this paper, we propose to extract the keypoints of the robot as auxiliary information to improve the data-efficiency of RL from image pixels. The proposed method, called KeyState, is evaluated on DeepMind Control Suite, a common benchmark for evaluating data-efficiency and performance of RL agents. The experimental results show that the performance of KeyState is, on average, 1.65 times better than the prior pixel-based methods.
更多查看译文
关键词
Reinforcement Learning,Robot Control,Key-point,Representation Learning,Sample Efficiency
AI 理解论文
溯源树
样例
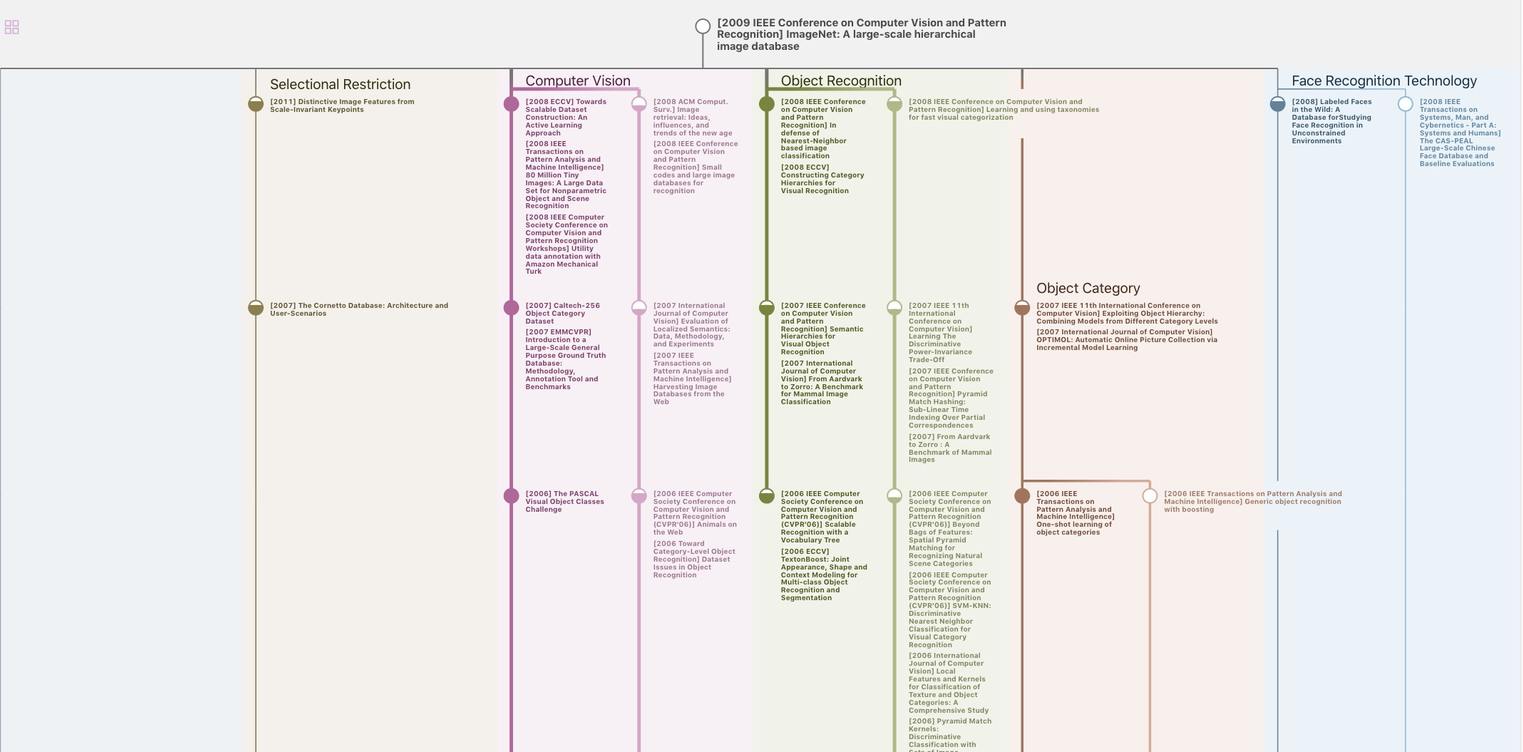
生成溯源树,研究论文发展脉络
Chat Paper
正在生成论文摘要