Interpretable MOOC Dropout Prediction using Different Ensemble Methods and XAI
2023 2nd International Conference on Applied Artificial Intelligence and Computing (ICAAIC)(2023)
摘要
Massive open online course (MOOC) has been around for several years, which started to gain traction in 2012 when Coursera was established. MOOCs use pre-recorded lectures and scheduled weekly tests to provide content and access to students over the internet. The dataset used in this study is taken from the KDDCUP 2015 dataset, a publicly available dataset. Here, 12 features are considered, they are browser access, navigate, average chapter delays, etc to comprehend the possibility of dropout. This research study intends to predict the dropout of a learner so that it can be prevented through manual interaction. Additionally, XAI is implemented to inter-pret the models to suggest MOOC platforms, which feature impact dropout the most. Further, different ensemble learning techniques, namely voting classifier and stacking are used. The proposed voting classifier uses five of the best-performing machine learning models. Then, the performance of the model is evaluated by using multiple metrics such as precision, recall, and F1-score. Finally, a recall of 97.636 % has been obtained with stacking and f1-score of 91.603 % has been obtained with the hard voting classifier.
更多查看译文
关键词
Machine Learning,Deep Learning,Massive Open Online Courses,Dropout Prediction,Ensemble Learning,Explainable Artificial Intelligence
AI 理解论文
溯源树
样例
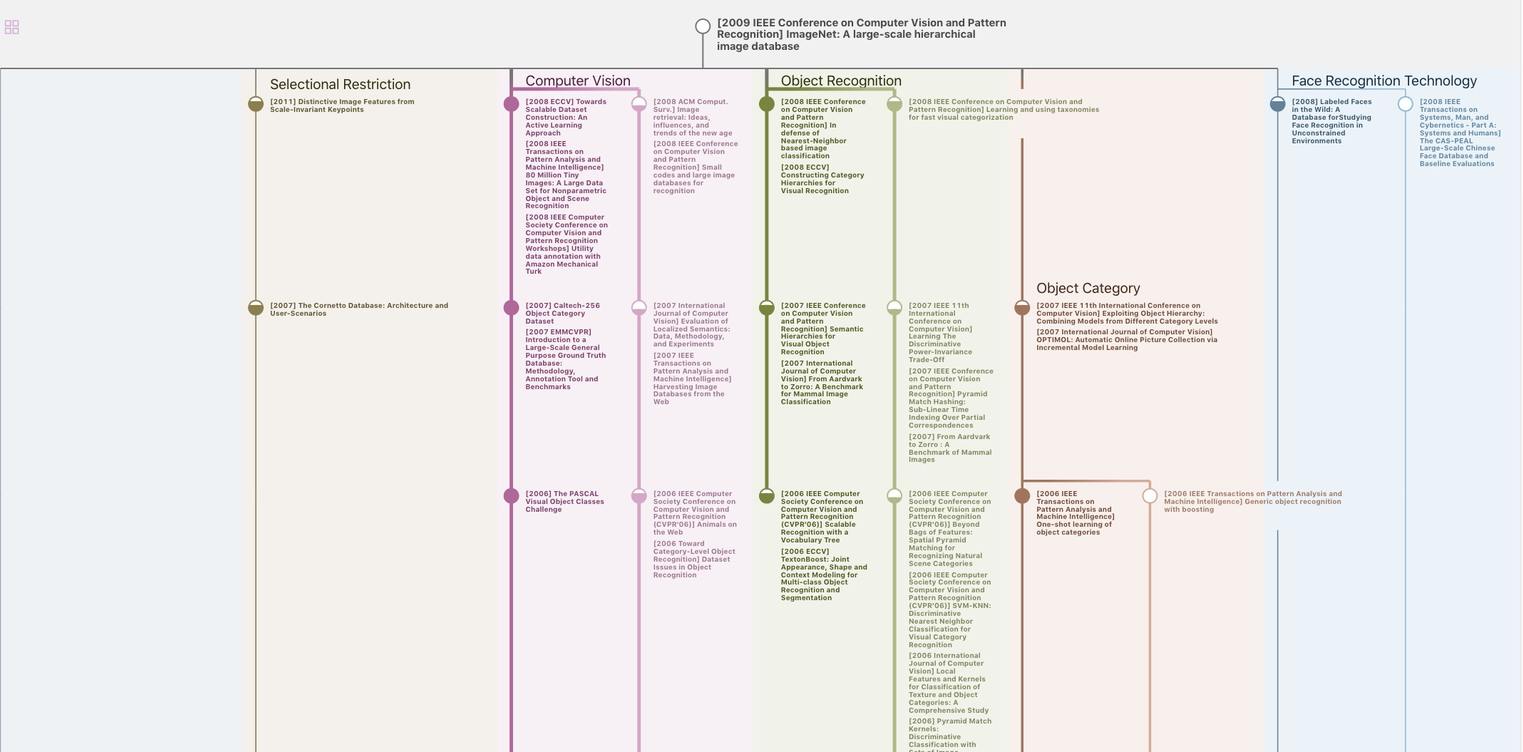
生成溯源树,研究论文发展脉络
Chat Paper
正在生成论文摘要