A Hierarchical Fusion SAR Image Change-Detection Method Based on HF-CRF Model
Remote Sensing(2023)
摘要
The mainstream methods for change detection in synthetic-aperture radar (SAR) images use difference images to define the initial change regions. However, methods can suffer from semantic collapse, which makes it difficult to determine semantic information about the changes. In this paper, we proposed a hierarchical fusion SAR image change-detection model based on hierarchical fusion conditional random field (HF-CRF). This model introduces multimodal difference images and constructs the fusion energy potential function using dynamic convolutional neural networks and sliding window entropy information. By using an iterative convergence process, the proposed method was able to accurately detect the change-detection regions. We designed a dynamic region convolutional semantic segmentation network with a two-branch structure (D-DRUNet) to accomplish feature fusion and the segmentation of multimodal difference images. The proposed network adopts a dual encoder-single decoder structure where the baseline is the UNet network that utilizes dynamic convolution kernels. D-DRUNet extracts multimodal difference features and completes semantic-level fusion. The Sobel operator is introduced to strengthen the multimodal difference-image boundary information and construct the dynamic fusion pairwise potential function, based on local boundary entropy. Finally, the final change result is stabilized by iterative convergence of the CRF energy potential function. Experimental results demonstrate that the proposed method outperforms existing methods in terms of the overall number of detection errors, and reduces the occurrence of false positives.
更多查看译文
关键词
change detection,hierarchical fusion,CRF,D-DRUNet,local boundary entropy
AI 理解论文
溯源树
样例
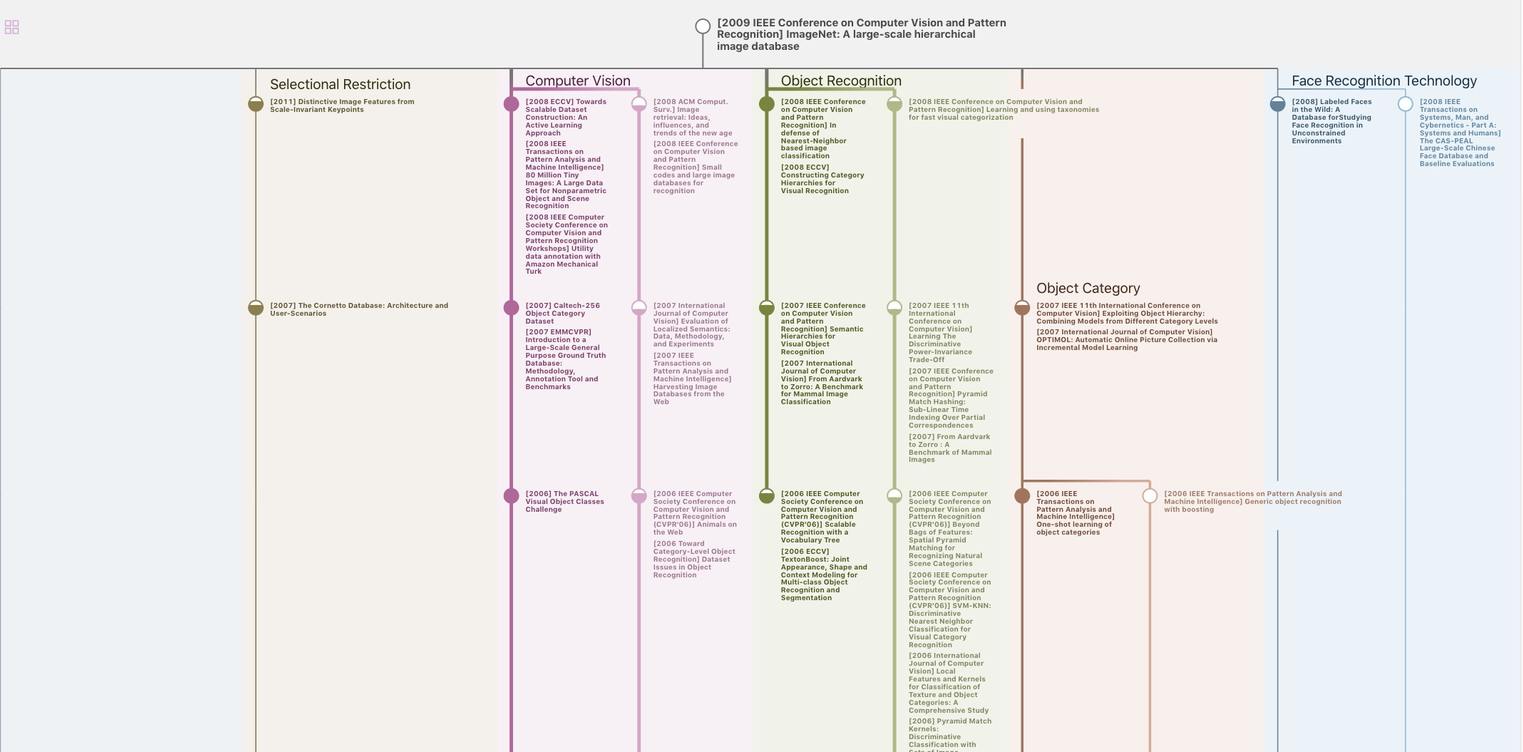
生成溯源树,研究论文发展脉络
Chat Paper
正在生成论文摘要