Unlocking Potential of 3D-aware GAN for More Expressive Face Generation
ICMR '23: Proceedings of the 2023 ACM International Conference on Multimedia Retrieval(2023)
摘要
As style-based image generators have achieved disentanglement in features by converting latent vector space to style vector space, numerous efforts have been made to enhance the controllability of the latent. However, existing methods for controllable models have limitations in precisely creating high-resolution faces with large expressions. The degradation is due to the dependence on the training dataset, as the high-resolution face datasets do not have sufficient expressive images. To tackle this challenge, we propose a robust training framework for 3D-aware generative adversarial networks to learn the high-quality generation of more expressive faces through a signed distance field. First, we propose a novel 3D enforcement loss to generate more expressive images in an unsupervised manner. Second, we introduce a partial training method to fine-tune the network on multiple datasets without loss of image resolution. Finally, we propose a ray-scaling scheme for the volume renderer to represent a face at arbitrary scales. Through the proposed framework, the network learns 3D face priors, such as expressional shapes of the parametric facial model, to generate detailed faces. The experimental results outperform the methods of the state of the art, showing strong benefits in the generation of high-resolution facial expressions.
更多查看译文
关键词
facial generation, 3D-aware GAN, controllable generative model, inversion, style-based generator
AI 理解论文
溯源树
样例
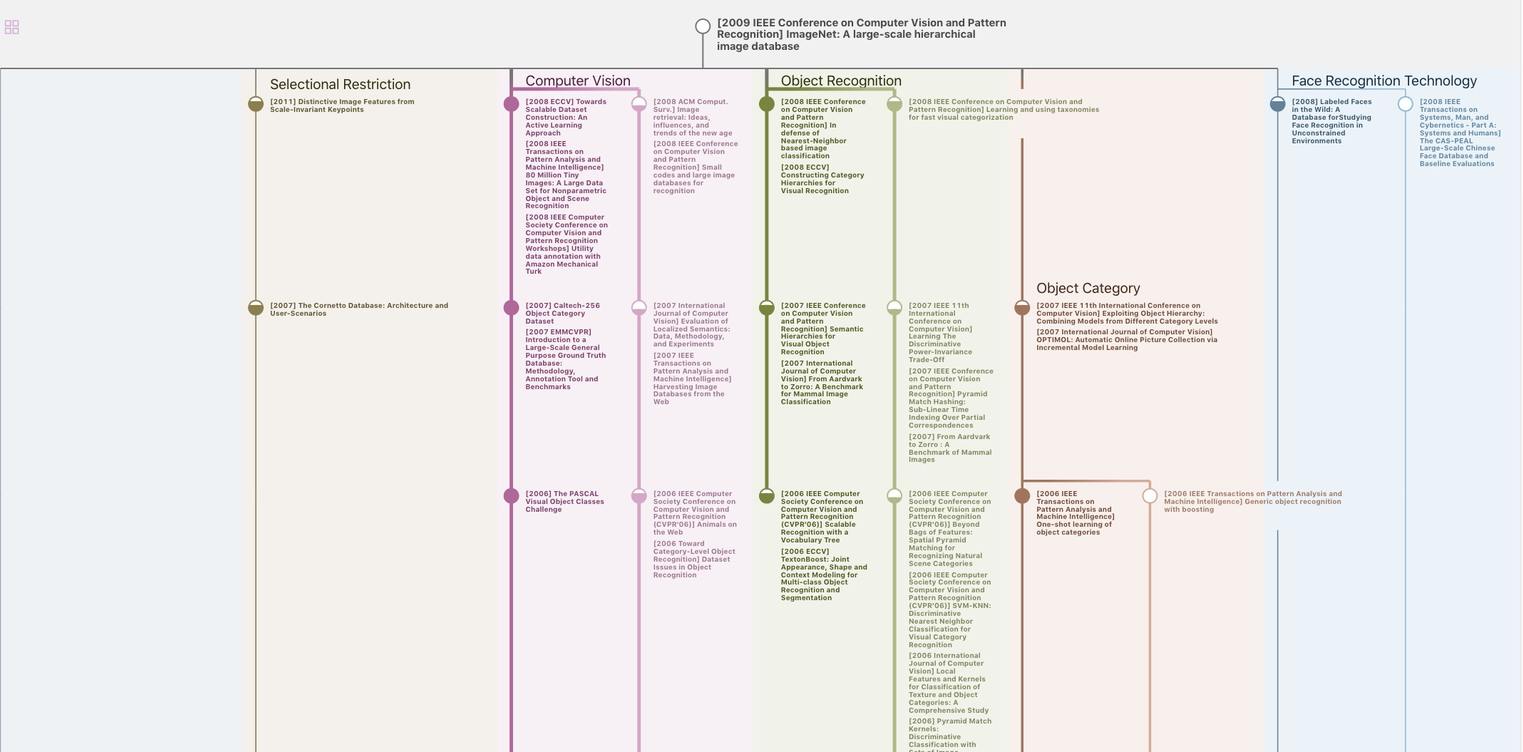
生成溯源树,研究论文发展脉络
Chat Paper
正在生成论文摘要