AVForensics: Audio-driven Deepfake Video Detection with Masking Strategy in Self-supervision
ICMR '23: Proceedings of the 2023 ACM International Conference on Multimedia Retrieval(2023)
摘要
Existing cross-dataset deepfake detection approaches exploit mouth-related mismatches between the auditory and visual modalities in fake videos to enhance generalisation to unseen forgeries. However, such methods inevitably suffer performance degradation with limited or unaltered mouth motions, we argue that face forgery detection consistently benefits from using high-level cues across the whole face region. In this paper, we propose a two-phase audio-driven multi-modal transformer-based framework, termed AVForensics, to perform deepfake video content detection from an audio-visual matching view related to full face. In the first pre-training phase, we apply the novel uniform masking strategy to model global facial features and learn temporally dense video representations in a self-supervised cross-modal manner, by capturing the natural correspondence between the visual and auditory modalities regardless of large-scaled labelled data and heavy memory usage. Then we use these learned representations to fine-tune for the down-stream deepfake detection task in the second phase, which encourages the model to offer accurate predictions based on captured global facial movement features. Extensive experiments and visualizations on various public datasets demonstrate the superiority of our self-supervised pre-trained method for achieving generalisable and robust deepfake video detection.
更多查看译文
关键词
Deepfake detection, audio-visual, masking strategy, self-supervision
AI 理解论文
溯源树
样例
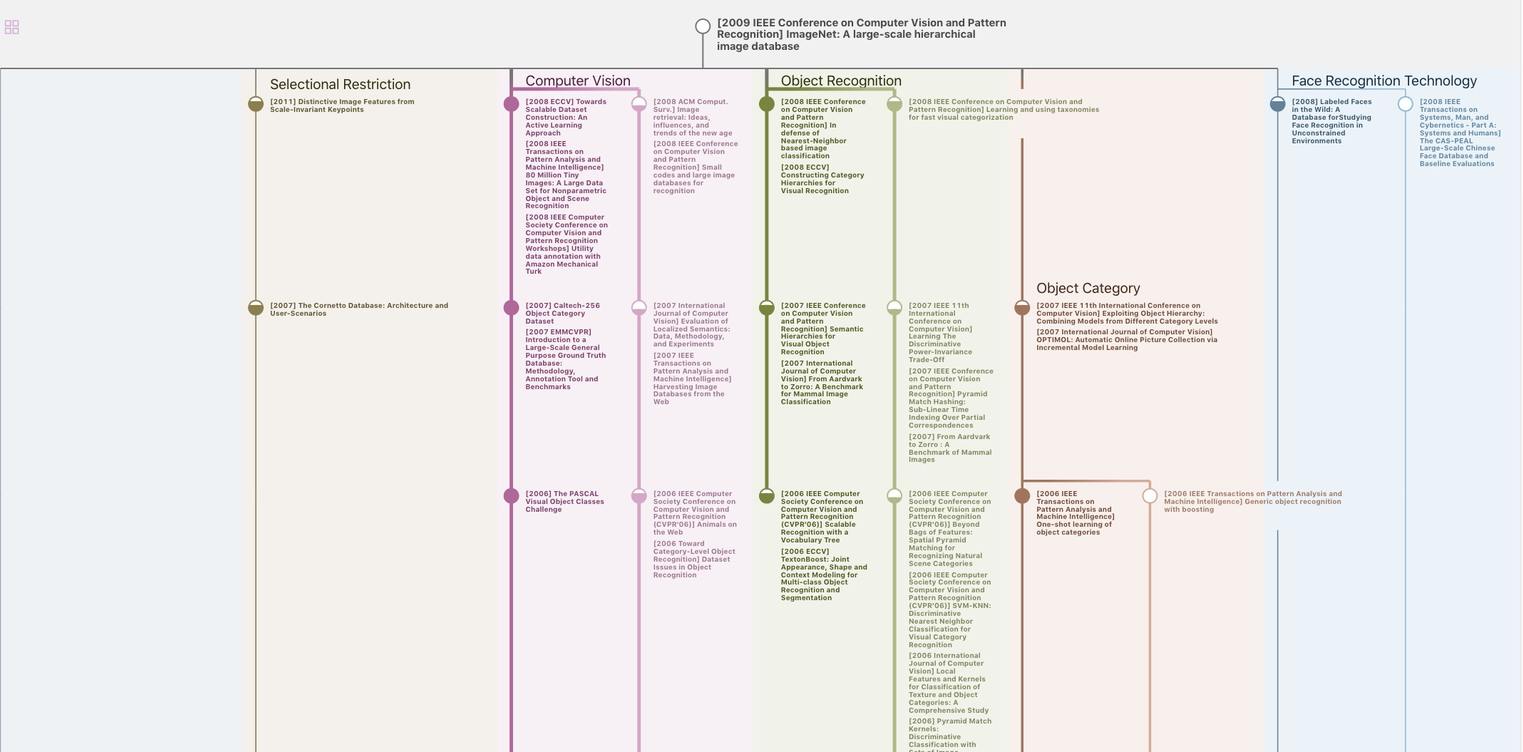
生成溯源树,研究论文发展脉络
Chat Paper
正在生成论文摘要