Data Augmentation for Improving Tail-traffic Robustness in Skill-routing for Dialogue Systems
CoRR(2023)
摘要
Large-scale conversational systems typically rely on a skill-routing component to route a user request to an appropriate skill and interpretation to serve the request. In such system, the agent is responsible for serving thousands of skills and interpretations which create a long-tail distribution due to the natural frequency of requests. For example, the samples related to play music might be a thousand times more frequent than those asking for theatre show times. Moreover, inputs used for ML-based skill routing are often a heterogeneous mix of strings, embedding vectors, categorical and scalar features which makes employing augmentation-based long-tail learning approaches challenging. To improve the skill-routing robustness, we propose an augmentation of heterogeneous skill-routing data and training targeted for robust operation in long-tail data regimes. We explore a variety of conditional encoder-decoder generative frameworks to perturb original data fields and create synthetic training data. To demonstrate the effectiveness of the proposed method, we conduct extensive experiments using real-world data from a commercial conversational system. Based on the experiment results, the proposed approach improves more than 80% (51 out of 63) of intents with less than 10K of traffic instances in the skill-routing replication task.
更多查看译文
关键词
tail-traffic,skill-routing
AI 理解论文
溯源树
样例
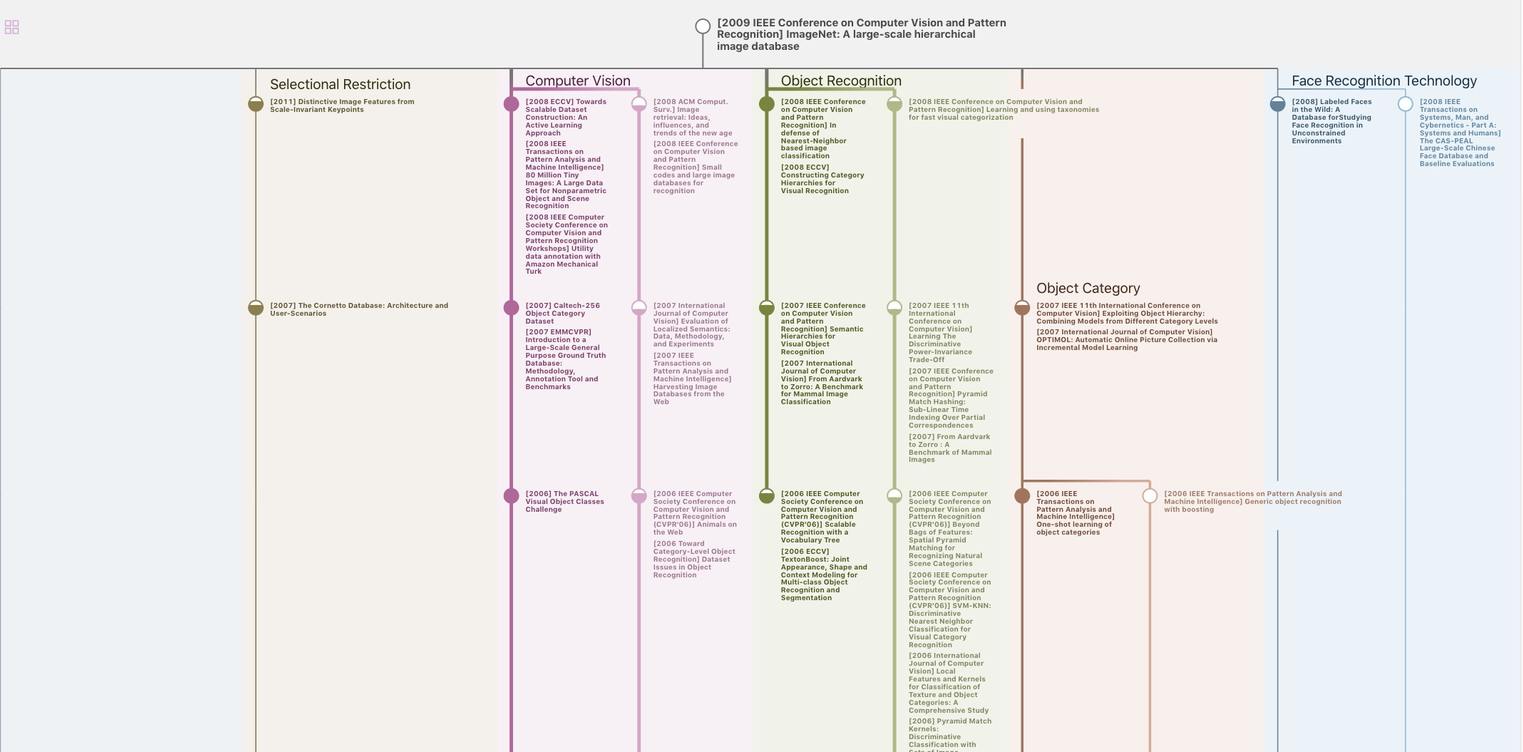
生成溯源树,研究论文发展脉络
Chat Paper
正在生成论文摘要