Self-Supervised Audio Teacher-Student Transformer for Both Clip-Level and Frame-Level Tasks
IEEE-ACM TRANSACTIONS ON AUDIO SPEECH AND LANGUAGE PROCESSING(2024)
摘要
Self-supervised learning (SSL) has emerged as a popular approach for learning audio representations. One goal of audio self-supervised pre-training is to transfer knowledge to downstream audio tasks, generally including clip-level and frame-level tasks. While frame-level tasks are important for fine-grained acoustic scene/event understanding, prior studies primarily evaluate on clip-level downstream tasks. In order to tackle both clip-level and frame-level tasks, this paper proposes Audio Teacher-Student Transformer (ATST), with a clip-level version (named ATST-Clip) and a frame-level version (named ATST-Frame), responsible for learning clip-level and frame-level representations, respectively. Both methods use a Transformer encoder and a teacher-student training scheme. We have carefully designed a view creation strategy for ATST-Clip and ATST-Frame. Specifically, ATST-Clip uses segment-wise data augmentations, and ATST-Frame integrates frame-wise data augmentations and masking. Experimental results show that our ATST-Frame model obtains state-of-the-art (SOTA) performances on most of the clip-level and frame-level downstream tasks. Especially, it outperforms other models by a large margin on the frame-level sound event detection task. In addition, the performance can be further improved by combining the two models through knowledge distillation.
更多查看译文
关键词
Task analysis,Transformers,Training,Self-supervised learning,Predictive models,Data augmentation,Speech processing,Audio representation learning,audio self-supervised learning
AI 理解论文
溯源树
样例
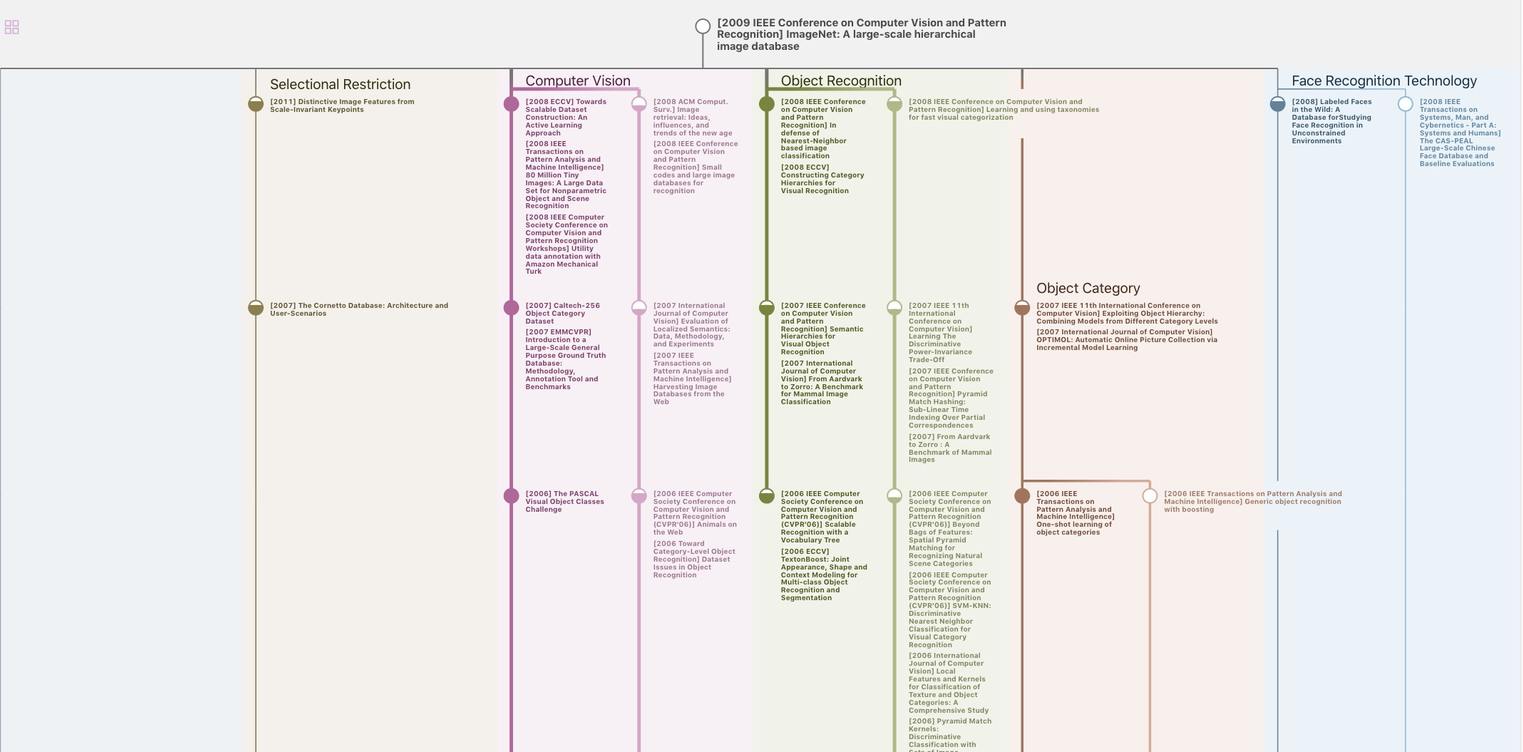
生成溯源树,研究论文发展脉络
Chat Paper
正在生成论文摘要