BeMap: Balanced Message Passing for Fair Graph Neural Network
CoRR(2023)
摘要
Graph Neural Network (GNN) has shown strong empirical performance in many downstream tasks by iteratively aggregating information from the local neighborhood of each node, i.e., message passing. However, concrete evidence has revealed that a graph neural network could be biased against certain demographic groups, which calls for the consideration of algorithmic fairness. Despite the increasing efforts in ensuring algorithmic fairness on graph neural networks, they often do not explicitly consider the induced bias caused by message passing in GNN during training. In this paper, we first investigate the problem of bias amplification in message passing. We empirically and theoretically demonstrate that message passing could amplify the bias when the 1-hop neighbors from different demographic groups are unbalanced. Guided by such analyses, we propose BeMap, a fair message passing method, that leverages a balance-aware sampling strategy to balance the number of the 1-hop neighbors of each node among different demographic groups. Extensive experiments on node classification demonstrate the efficacy of our proposed BeMap method in mitigating bias while maintaining classification accuracy.
更多查看译文
关键词
fair graph neural network,balanced message passing,neural network
AI 理解论文
溯源树
样例
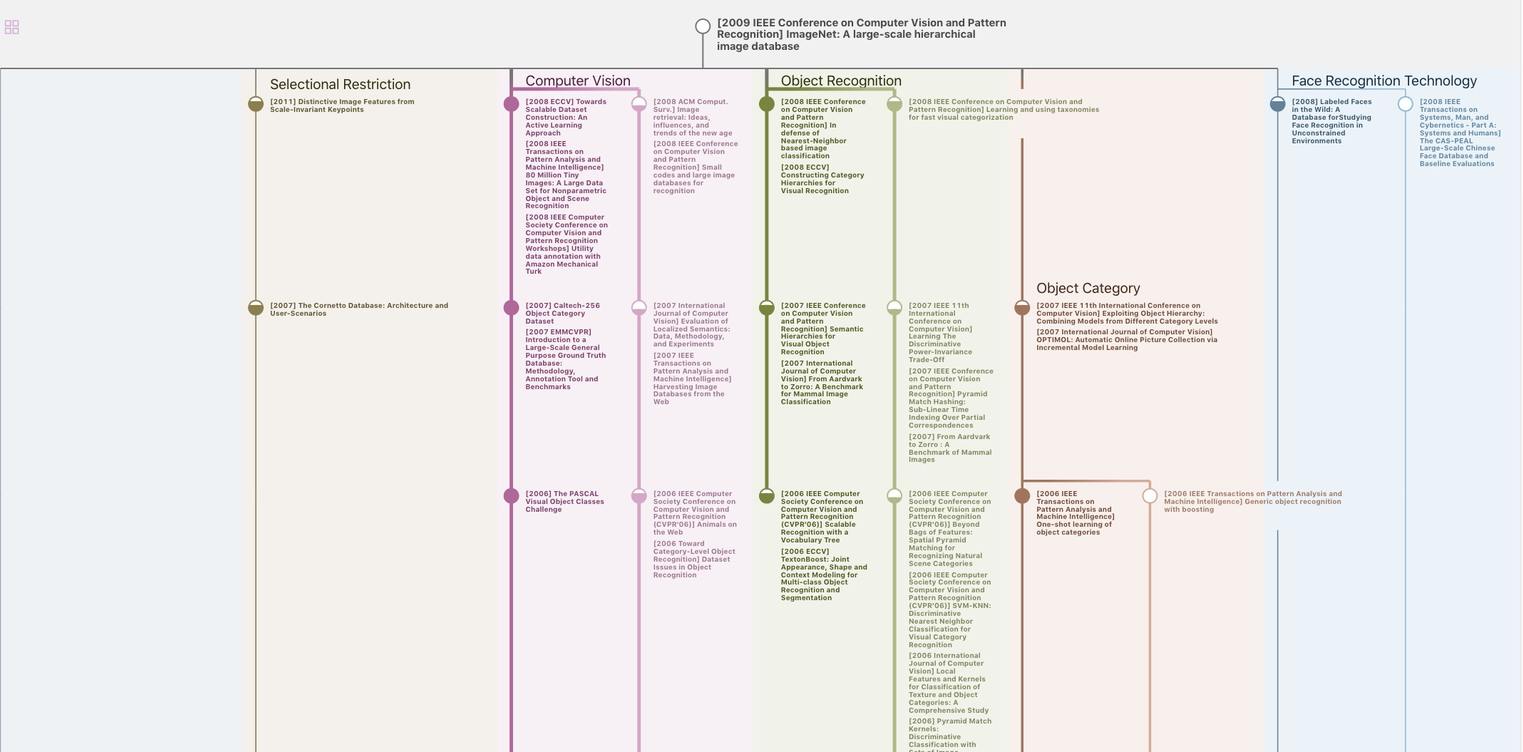
生成溯源树,研究论文发展脉络
Chat Paper
正在生成论文摘要