Active Sparse Conversations for Improved Audio-Visual Embodied Navigation
CoRR(2023)
摘要
Efficient navigation towards an audio-goal necessitates an embodied agent to not only possess the ability to use audio-visual cues effectively, but also be equipped to actively (but occasionally) seek human/oracle assistance without sacrificing autonomy, e.g., when it is uncertain of where to navigate towards locating a noisy or sporadic audio goal. To this end, we present CAVEN -- a conversational audio-visual embodied navigation agent that is capable of posing navigation questions to a human/oracle and processing the oracle responses; both in free-form natural language. At the core of CAVEN is a multimodal hierarchical reinforcement learning (RL) setup that is equipped with a high-level policy that is trained to choose from one of three low-level policies (at every step), namely: (i) to navigate using audio-visual cues, or (ii) to frame a question to the oracle and receive a short or detailed response, or (iii) ask generic questions (when unsure of what to ask) and receive instructions. Key to generating the agent's questions is our novel TrajectoryNet that forecasts the most likely next steps to the goal and a QuestionNet that uses these steps to produce a question. All the policies are learned end-to-end via the RL setup, with penalties to enforce sparsity in receiving navigation instructions from the oracle. To evaluate the performance of CAVEN, we present extensive experiments on the SoundSpaces framework for the task of semantic audio-visual navigation. Our results show that CAVEN achieves upto 12% gain in performance over competing methods, especially in localizing new sound sources, even in the presence of auditory distractions.
更多查看译文
关键词
active sparse conversations,navigation,audio-visual
AI 理解论文
溯源树
样例
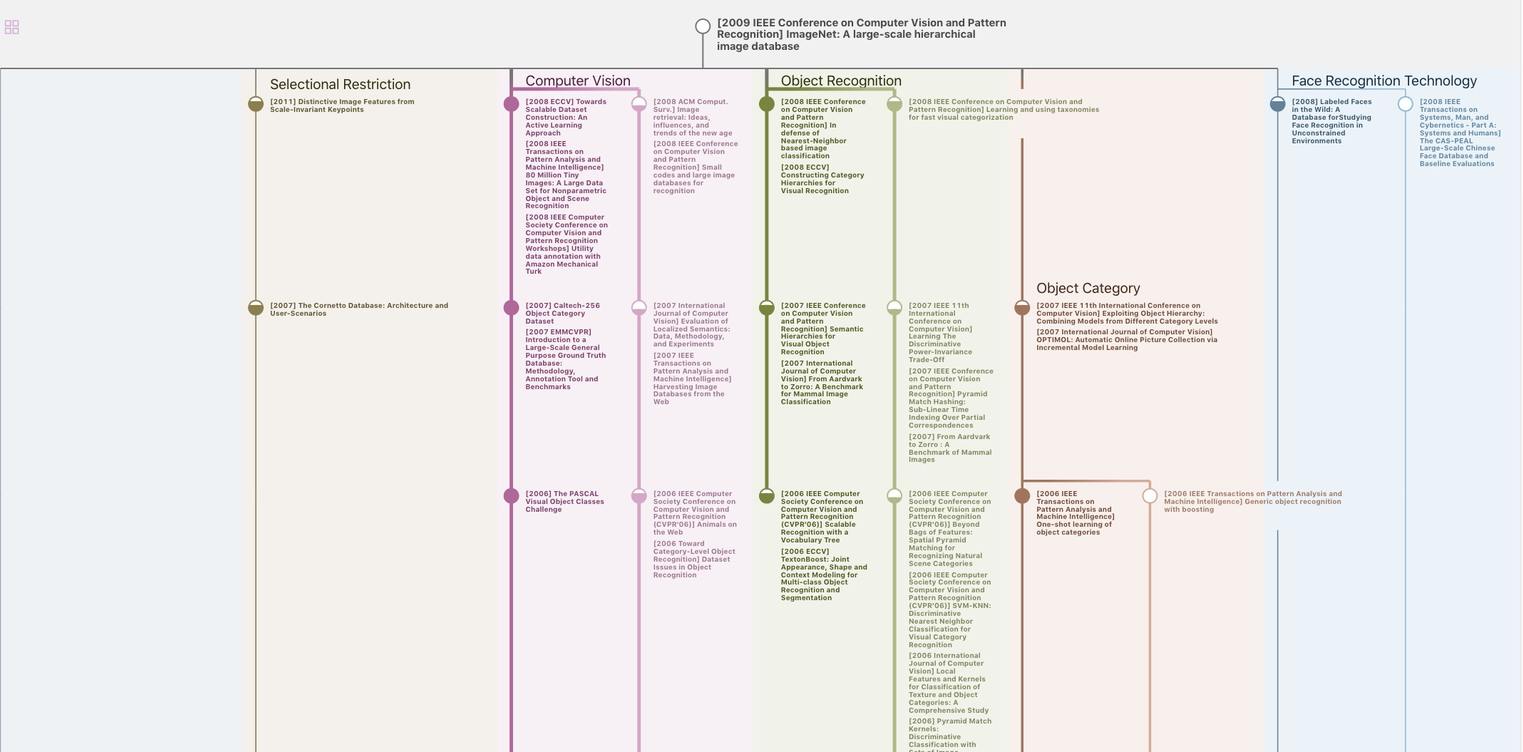
生成溯源树,研究论文发展脉络
Chat Paper
正在生成论文摘要