Iterative Translation Refinement with Large Language Models
CoRR(2023)
摘要
Large language models have shown surprising performances in understanding instructions and performing natural language tasks. In this paper, we propose iterative translation refinement to leverage the power of large language models for more natural translation and post-editing. We show that by simply involving a large language model in an iterative process, the output quality improves beyond mere translation. Extensive test scenarios with GPT-3.5 reveal that although iterations reduce string-based metric scores, neural metrics indicate comparable if not improved translation quality. Further, human evaluations demonstrate that our method effectively reduces translationese compared to initial GPT translations and even human references, especially for into-English directions. Ablation studies underscore the importance of anchoring the refinement process to the source input and a reasonable initial translation.
更多查看译文
关键词
iterative translation refinement,models
AI 理解论文
溯源树
样例
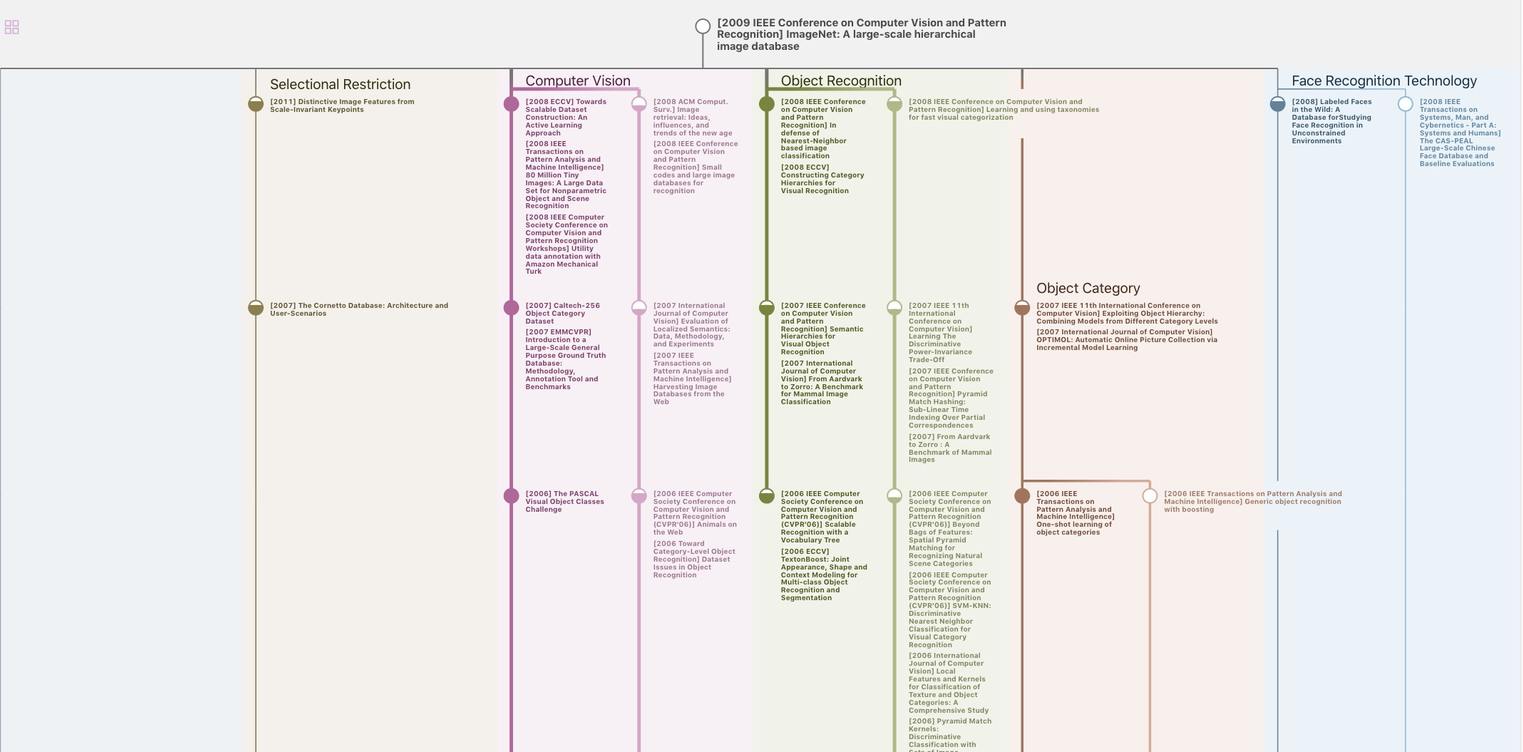
生成溯源树,研究论文发展脉络
Chat Paper
正在生成论文摘要