Spherical Fourier Neural Operators: Learning Stable Dynamics on the Sphere
CoRR(2023)
摘要
Fourier Neural Operators (FNOs) have proven to be an efficient and effective method for resolution-independent operator learning in a broad variety of application areas across scientific machine learning. A key reason for their success is their ability to accurately model long-range dependencies in spatio-temporal data by learning global convolutions in a computationally efficient manner. To this end, FNOs rely on the discrete Fourier transform (DFT), however, DFTs cause visual and spectral artifacts as well as pronounced dissipation when learning operators in spherical coordinates since they incorrectly assume a flat geometry. To overcome this limitation, we generalize FNOs on the sphere, introducing Spherical FNOs (SFNOs) for learning operators on spherical geometries. We apply SFNOs to forecasting atmospheric dynamics, and demonstrate stable auto\-regressive rollouts for a year of simulated time (1,460 steps), while retaining physically plausible dynamics. The SFNO has important implications for machine learning-based simulation of climate dynamics that could eventually help accelerate our response to climate change.
更多查看译文
关键词
sphere,stable dynamics,operators,learning
AI 理解论文
溯源树
样例
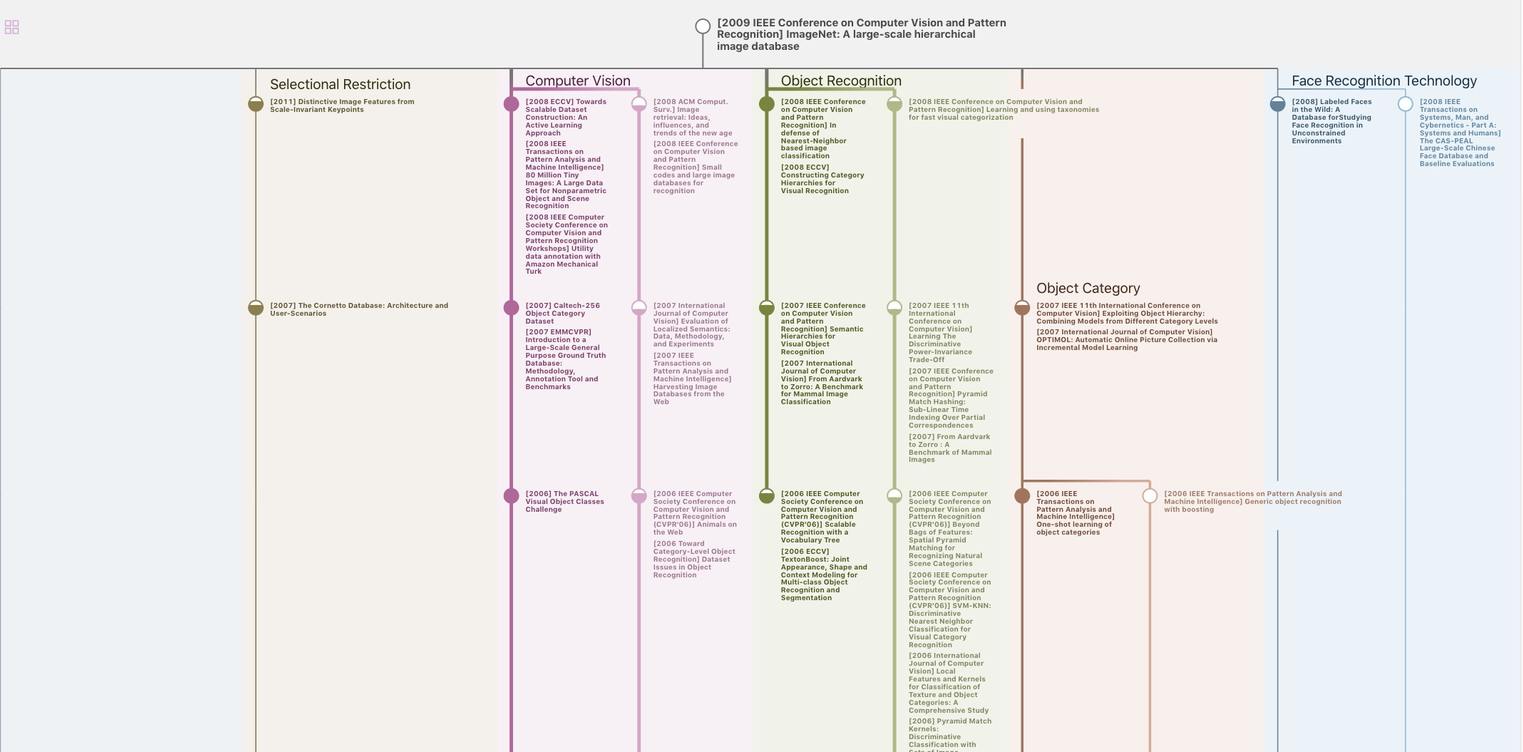
生成溯源树,研究论文发展脉络
Chat Paper
正在生成论文摘要