Information Flow Control in Machine Learning through Modular Model Architecture
CoRR(2023)
摘要
In today's machine learning (ML) models, any part of the training data can affect its output. This lack of control for information flow from training data to model output is a major obstacle in training models on sensitive data when access control only allows individual users to access a subset of data. To enable secure machine learning for access controlled data, we propose the notion of information flow control for machine learning, and develop a secure Transformer-based language model based on the Mixture-of-Experts (MoE) architecture. The secure MoE architecture controls information flow by limiting the influence of training data from each security domain to a single expert module, and only enabling a subset of experts at inference time based on an access control policy. The evaluation using a large corpus of text data shows that the proposed MoE architecture has minimal (1.9%) performance overhead and can significantly improve model accuracy (up to 37%) by enabling training on access-controlled data.
更多查看译文
关键词
modular model
AI 理解论文
溯源树
样例
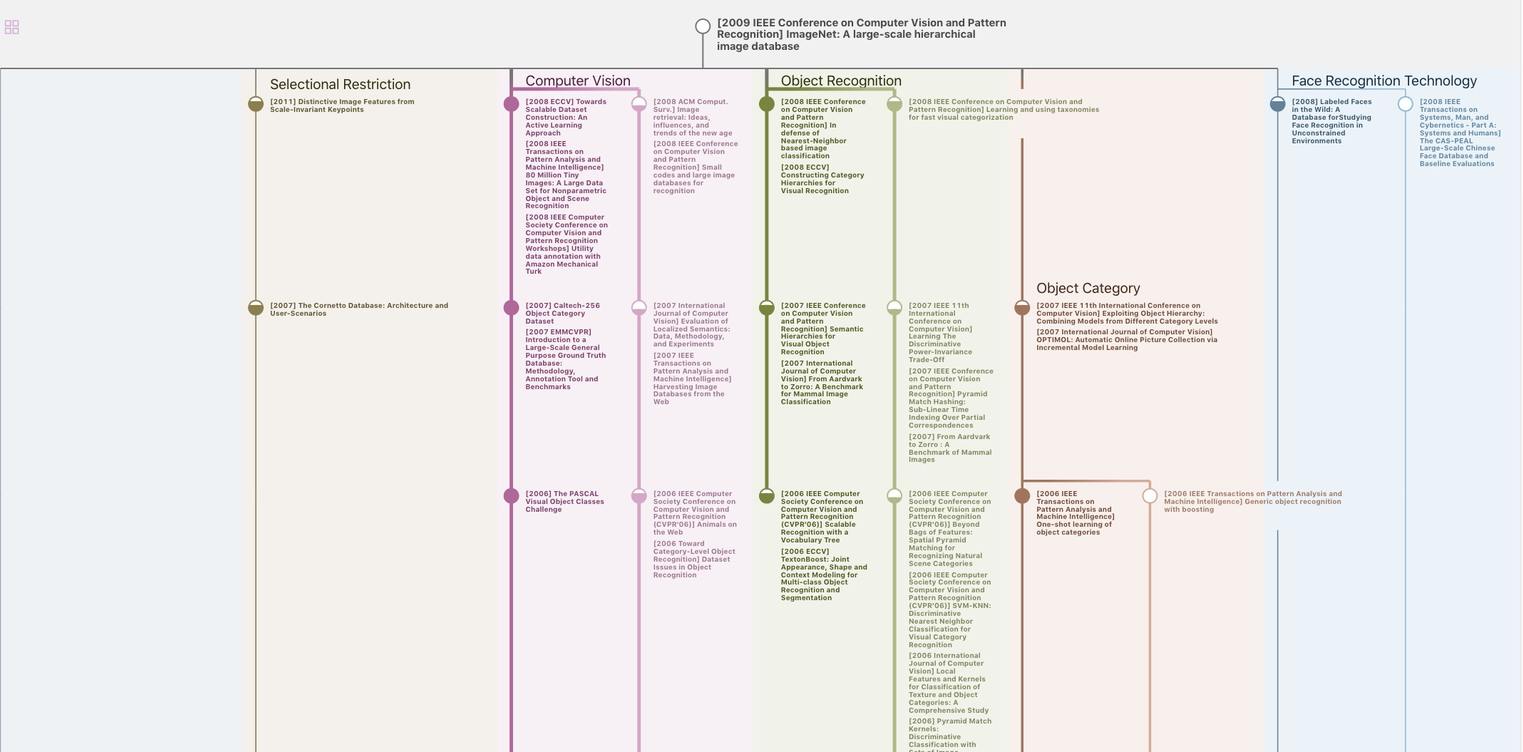
生成溯源树,研究论文发展脉络
Chat Paper
正在生成论文摘要