Prior-Guided Contrastive Image Compression for Underwater Machine Vision
IEEE Transactions on Circuits and Systems for Video Technology(2023)
摘要
Machine analysis of underwater images is essential to most underwater applications. However, both the limitation of communication bandwidth and underwater degradation bring much difficulty to accurate machine recognition at the end system. Few existing underwater compression methods consider unique underwater prior knowledge to better serve for machine vision under low bit-rates. To address this problem, we propose a novel underwater image compression framework for machines, which utilizes underwater priors to contrastively enhance degraded features by contrastive learning and efficiently compress machine-friendly features under low bit-rates. A dataset is built to provide positive and negative samples for contrastive learning based on machine analysis performance. At the encoder side, a feature extractor and a feature encoder are employed to extract machine-related features and compress them into compact representations. To alleviate the effect of underwater degradation on machine vision, a prior-guided contrastive feature enhancement module is proposed to learn more machine-friendly features based on positive and negative samples from our dataset. Then a feature refinement block is designed to remove channel-wise redundancy and focus spatial-wise importance based on high similarity of machine-related features and characteristics of underwater images. More compact representations are obtained without degrading analysis performance under low bit-rates. At the decoder side, both machine-friendly features and image are reconstructed to support different types of analysis tasks. Experimental results demonstrate the superiority of our framework in machine vision tasks compared with traditional compression methods and learned-based methods. Besides, our method still preserves basic capability of human perception.
更多查看译文
关键词
Underwater image compression,feature enhance,contrastive learning
AI 理解论文
溯源树
样例
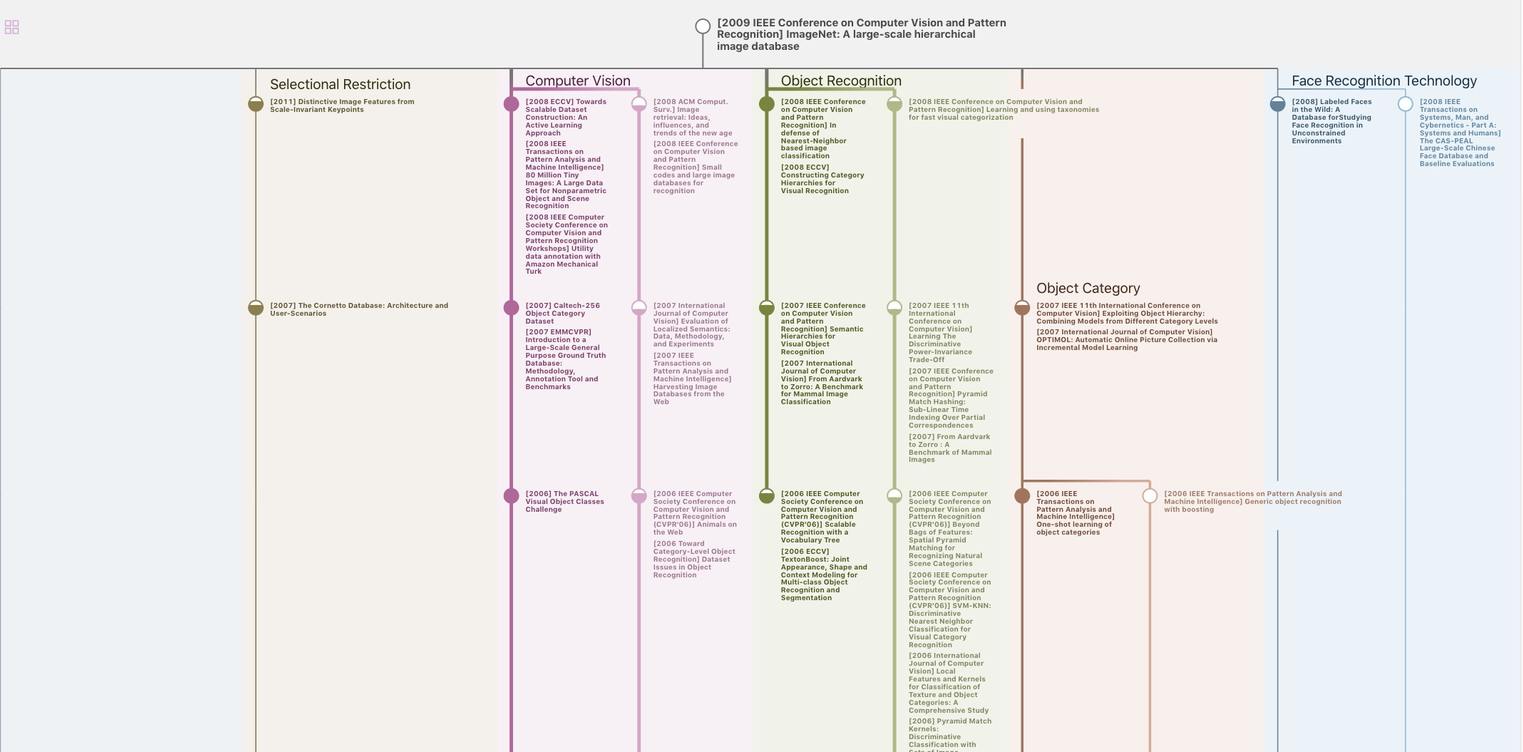
生成溯源树,研究论文发展脉络
Chat Paper
正在生成论文摘要