Asymmetric Loss Functions for Noise-Tolerant Learning: Theory and Applications
IEEE Transactions on Pattern Analysis and Machine Intelligence(2023)
摘要
Supervised deep learning has achieved tremendous success in many computer vision tasks, which however is prone to overfit noisy labels. To mitigate the undesirable influence of noisy labels, robust loss functions offer a feasible approach to achieve noise-tolerant learning. In this work, we systematically study the problem of noise-tolerant learning with respect to both classification and regression. Specifically, we propose a new class of loss function, namely
asymmetric loss functions
(ALFs), which are tailored to satisfy the Bayes-optimal condition and thus are robust to noisy labels. For classification, we investigate general theoretical properties of ALFs on categorical noisy labels, and introduce the asymmetry ratio to measure the asymmetry of a loss function. We extend several commonly-used loss functions, and establish the necessary and sufficient conditions to make them asymmetric and thus noise-tolerant. For regression, we extend the concept of noise-tolerant learning for image restoration with continuous noisy labels. We theoretically prove that
$\ell _{p}$
loss (
$p>0$
) is noise-tolerant for targets with the additive white Gaussian noise. For targets with general noise, we introduce two losses as surrogates of
$\ell _{0}$
loss that seeks the mode when clean pixels keep dominant. Experimental results demonstrate that ALFs can achieve better or comparative performance compared with the state-of-the-arts. The source code of our method is available at:
https://github.com/hitcszx/ALFs
.
更多查看译文
关键词
learning,loss,noise-tolerant
AI 理解论文
溯源树
样例
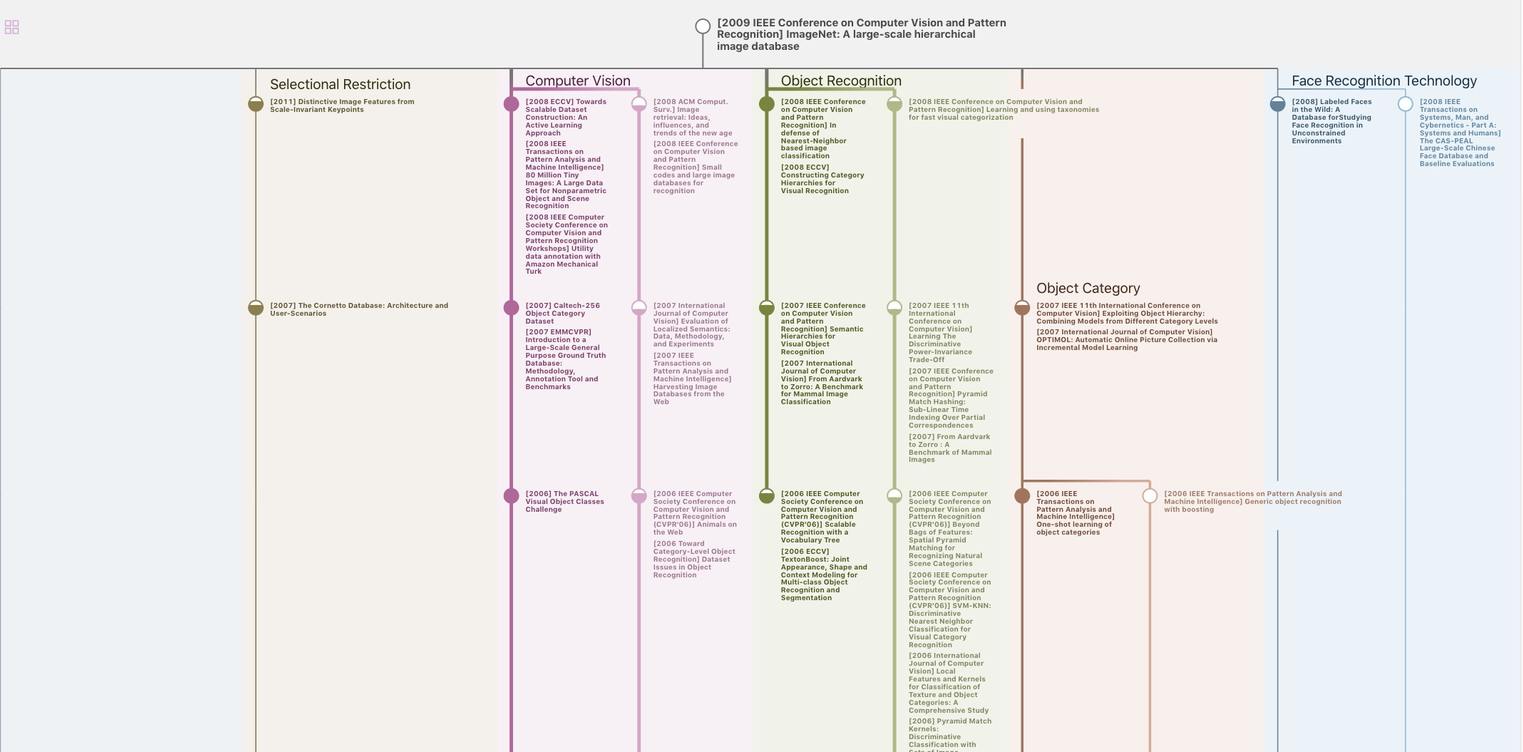
生成溯源树,研究论文发展脉络
Chat Paper
正在生成论文摘要