M3GAN: A masking strategy with a mutable filter for multidimensional anomaly detection
KNOWLEDGE-BASED SYSTEMS(2023)
摘要
With the advent of the big data era, the detection of anomalies in time series data, especially multidimensional time series data, has received a great deal of attention from researchers in many fields, e.g., industry, medicine, and aerospace. Multidimensional time series anomaly detection (MTSAD) methods aim to discover anomalies. They play an important early warning role in protecting people from possible property damage and personal injury. Many current GAN-based anomaly detection methods suffer from poor robustness, poor generalization, and low accuracy. The M2GAN (a GAN framework based on a masking strategy for multidimensional anomaly detection) proposed in this paper uses the masking method to diversify the data and improve the robustness of the model. This paper further proposes the M3GAN (M2GAN with a mutable filter), which can utilize the different characteristics of different filters to select a suitable model for the features of the data, thus enhancing the generalization ability and improving the accuracy. The experiments prove the effectiveness of the proposed method.(c) 2023 Elsevier B.V. All rights reserved.
更多查看译文
关键词
Anomaly detection,Multidimensional time series analysis,Masking strategy,Generative adversarial network,Mutable filter
AI 理解论文
溯源树
样例
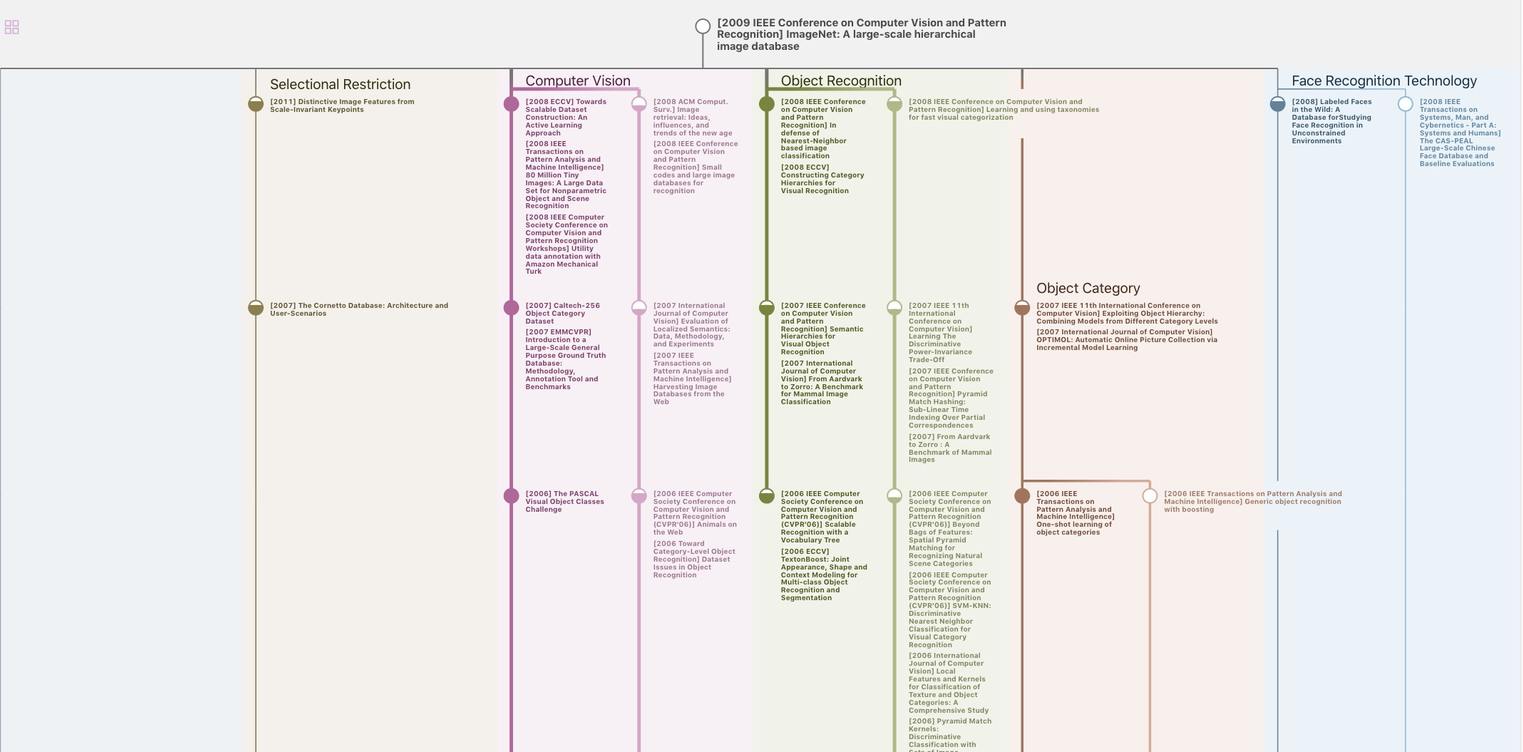
生成溯源树,研究论文发展脉络
Chat Paper
正在生成论文摘要