A hybrid deep convolutional neural network-based electronic nose for pollution detection purposes
CHEMOMETRICS AND INTELLIGENT LABORATORY SYSTEMS(2023)
摘要
- in the electronic nose fields, many types of research have been focused on deep learning for gas classification. Compared to traditional machine learning algorithms such as K-Nearest Neighbors (KNN) and Support Vector Machines (SVM), convolutional neural network (CNN) architectures can classify gases, resulting in higher classification accuracy. In this study, a hybrid convolutional neural network with linear discriminant analysis (CNN-LDA) was proposed for the classification of pollutant gases. One open-source gas dataset applied the proposed model. CNN and LDA models were both used for feature extraction and classification. Results showed the reliability of the hybrid CNN-LDA model, which achieved the highest test accuracy with a score of 93%, compared to the individual CNN and LDA models with classification accuracies of 90% and 83%, respectively. Metrics such as accuracy, recall, F1 score, and precision, allowed us to combine the results of the/experiments used.
更多查看译文
关键词
Linear discriminant analysis, Convolutional neural network, Electronic nose, Air pollution, Hybrid method
AI 理解论文
溯源树
样例
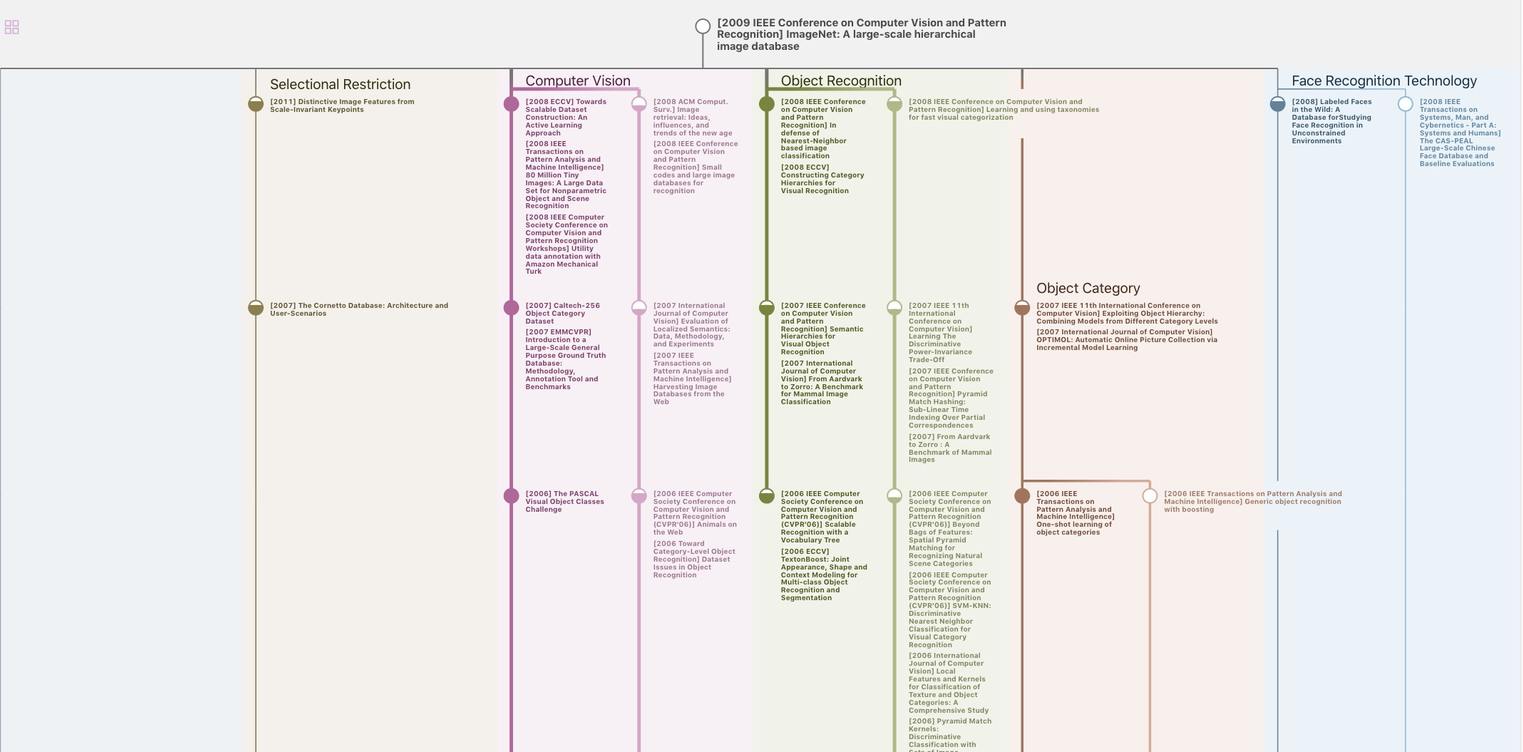
生成溯源树,研究论文发展脉络
Chat Paper
正在生成论文摘要