Bloom’s Taxonomy-based exam question classification: The outcome of CNN and optimal pre-trained word embedding technique
EDUCATION AND INFORMATION TECHNOLOGIES(2023)
摘要
The automated classification of examination questions based on Bloom’s Taxonomy (BT) aims to assist the question setters so that high-quality question papers are produced. Most studies to automate this process adopted the machine learning approach, and only a few utilised the deep learning approach. The pre-trained contextual and non-contextual word embedding techniques effectively solved various natural language processing tasks. This study aims to identify the optimal pre-trained word embedding technique and propose a Convolutional Neural Network (CNN) model with the optimal word embedding technique. Therefore, non-contextual word embedding techniques: Word2vec, GloVe, and FastText, whereas contextualised embedding techniques: BERT, RoBERTa, and ELECTRA, were analysed in this study with two datasets. The experiment results showed that FastText is the most optimal technique in the first dataset, whereas RoBERTa is in the second dataset. This outcome of the first dataset differs from the text classification since contextual embedding generally outperforms non-contextual embedding. It could be due to the comparatively smaller size of the first dataset and the shorter length of the examination questions. Since RoBERTa is the most optimal word embedding technique in the second dataset, hence used along with CNN to build the model. This study used CNN instead of Recurrent Neural Networks (RNNs) since extracting relevant features is more important than the learning sequence from data in the context of examination question classification. The proposed CNN model achieved approximately 86% in both weighted F1-score and accuracy and outperformed all the models proposed by past studies, including RNNs. The proposed model’s robustness could be assessed in the future using a more comprehensive dataset.
更多查看译文
关键词
exam question classification,taxonomy-based,pre-trained
AI 理解论文
溯源树
样例
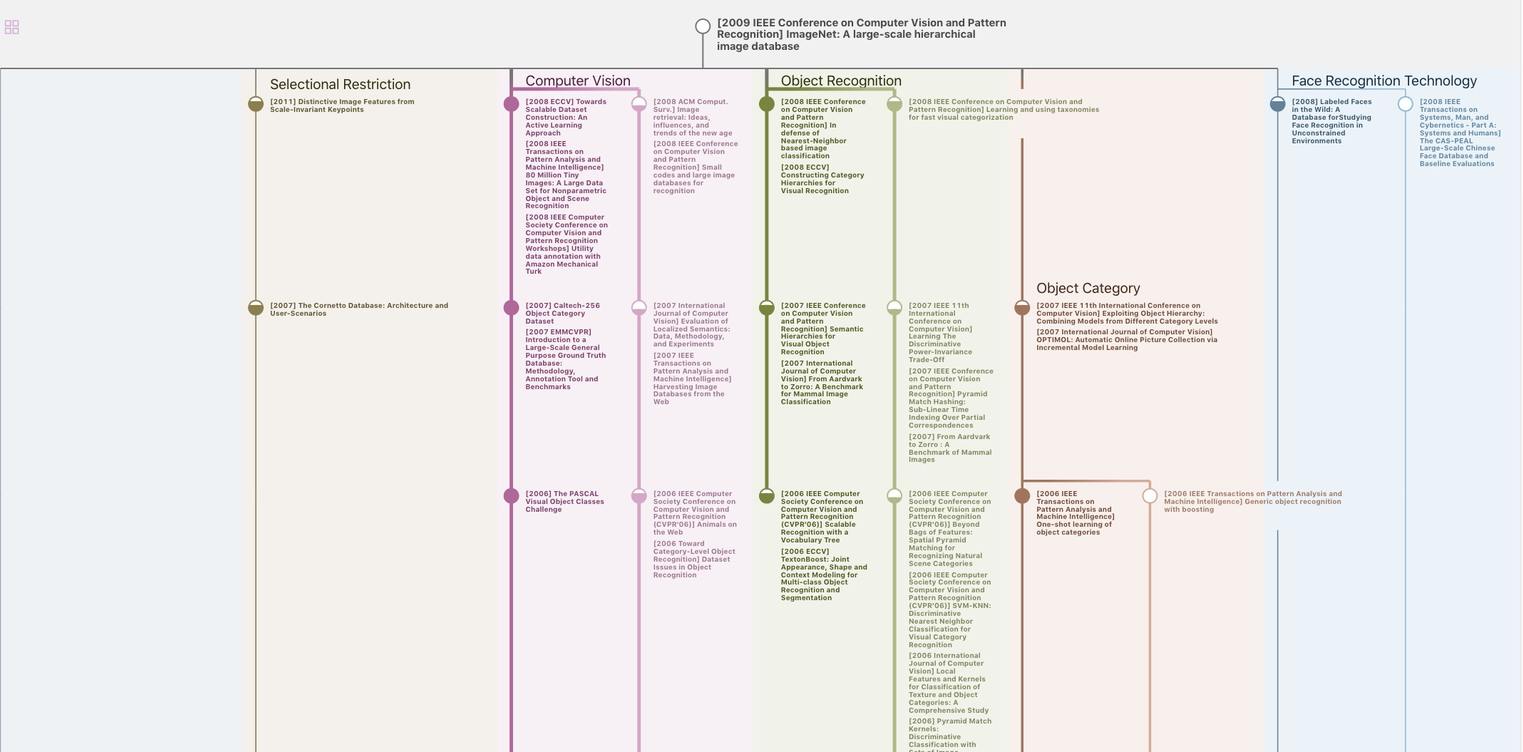
生成溯源树,研究论文发展脉络
Chat Paper
正在生成论文摘要