Uncertainty quantification and propagation across a multi-model computational framework for the tailored design of additively manufactured shape memory alloys
ADDITIVE MANUFACTURING(2023)
摘要
Integrated computational materials engineering (ICME) combines the utility and efficiency of simulations with experimentation to drive forward materials design and discovery. These physics-based and data-driven frame-works have enabled material advancement by querying the complex process-structure-property-performance relationships to inform and guide experiments for the cost-effective design of alloy systems. In this study, a proven computational framework is presented and applied towards the tailored design of additively manufactured (AM) high-temperature NiTiHf shape-memory alloy (SMA) parts. Specifically, the effort deploys a design tool to attain specific transformation temperatures by composition control through differential evaporation, which in turn depends on processing conditions. This framework consists of a fast-acting discrete source model to simulate thermal history, a multi-layer model to account for composition evolution across melt pools, and a differential evaporation model to evaluate Nickel loss throughout the fabrication process. Besides the development of this multi-model chain, proper quantification of model uncertainties is critical to an ICME approach for materials design. Addressing these concerns, the parameter calibration and uncertainty quantification (UQ) of hierarchical model components is conducted through a Markov Chain Monte Carlo (MCMC) Bayesian approach over either the model itself or a representative Gaussian process-based surrogate model. These uncertainties are propagated across the models to the final response, i.e., martensitic start temperature. Subsequently, the hierarchical model framework is validated by comparing the experimental results with the most plausible values and uncertainty bounds obtained for the multi-model predictions at different processing conditions. From this calibrated and validated framework, process maps to streamline and illustrate the tailored design of AM high-temperature NiTiHf SMAs are developed, which demonstrates a promising path towards efficient design under uncertainty in additive manufacturing processes.
更多查看译文
关键词
alloys,uncertainty,multi-model
AI 理解论文
溯源树
样例
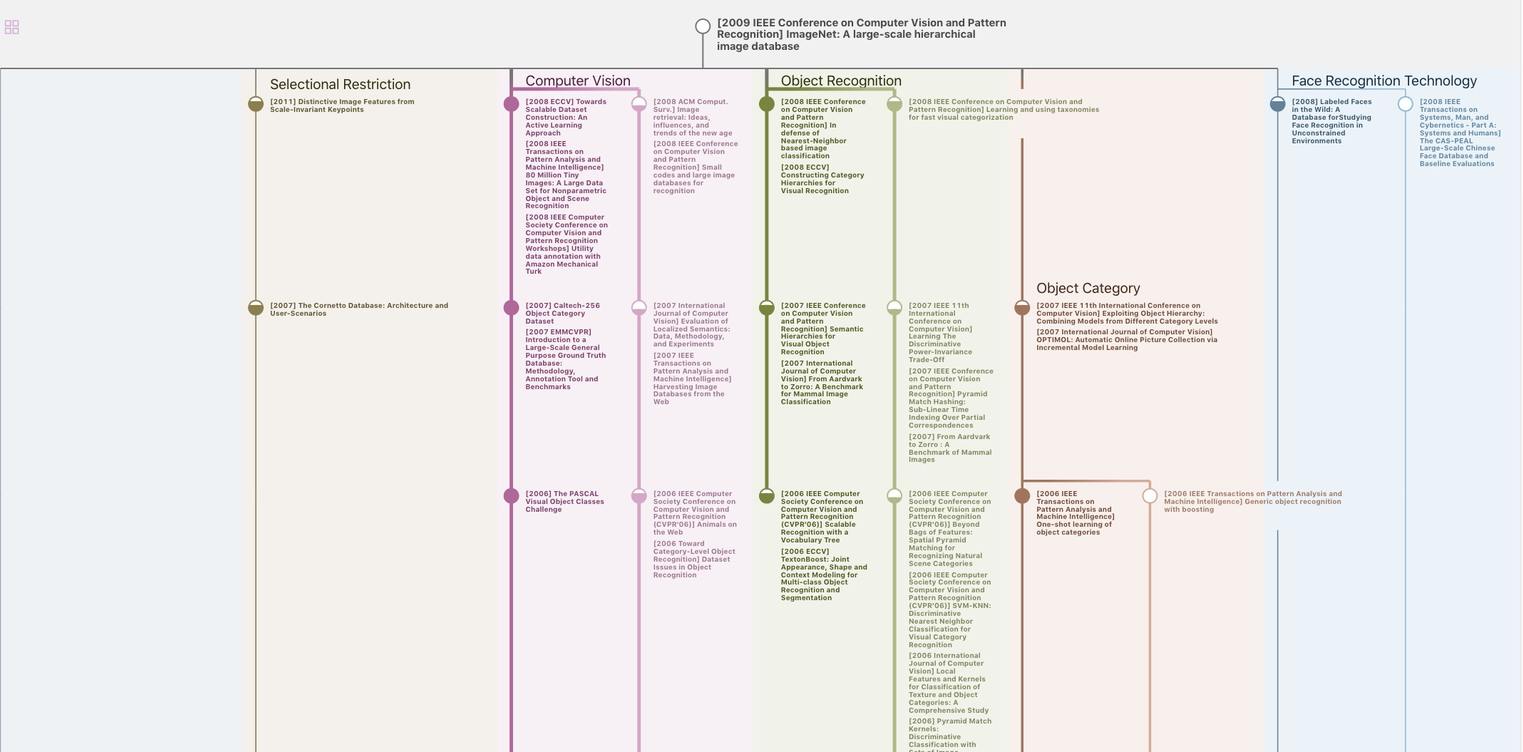
生成溯源树,研究论文发展脉络
Chat Paper
正在生成论文摘要