Preliminary evidence on machine learning approaches for clusterizing students? cognitive profile
HELIYON(2023)
摘要
Assessing the cognitive abilities of students in academic contexts can provide valuable insights for teachers to identify their cognitive profile and create personalized teaching strategies. While numerous studies have demonstrated promising outcomes in clustering students based on their cognitive profiles, effective comparisons between various clustering methods are lacking in the current literature. In this study, we aim to compare the effectiveness of two clustering techniques to group stu-dents based on their cognitive abilities including general intelligence, attention, visual percep-tion, working memory, and phonological awareness. 292 students, aged 11-15 years, participated in the study. A two-level approach based on the joint use of Kohonen's Self-Organizing Map (SOMs) and k -means clustering algorithm was compared with an approach based on the k-means clustering algorithm only. The resulting profiles were then predicted via AdaBoost and ANN supervised algorithms. The results showed that the two-level approach provides the best solution for this problem while the ANN algorithm was the winner in the classification problem. These results laying the foundations for developing a useful instrument for predicting the students' cognitive profile.
更多查看译文
关键词
Machine learning, Self -organizing maps, Cognitive profiling, Specific learning difficulties (SLD), K -means
AI 理解论文
溯源树
样例
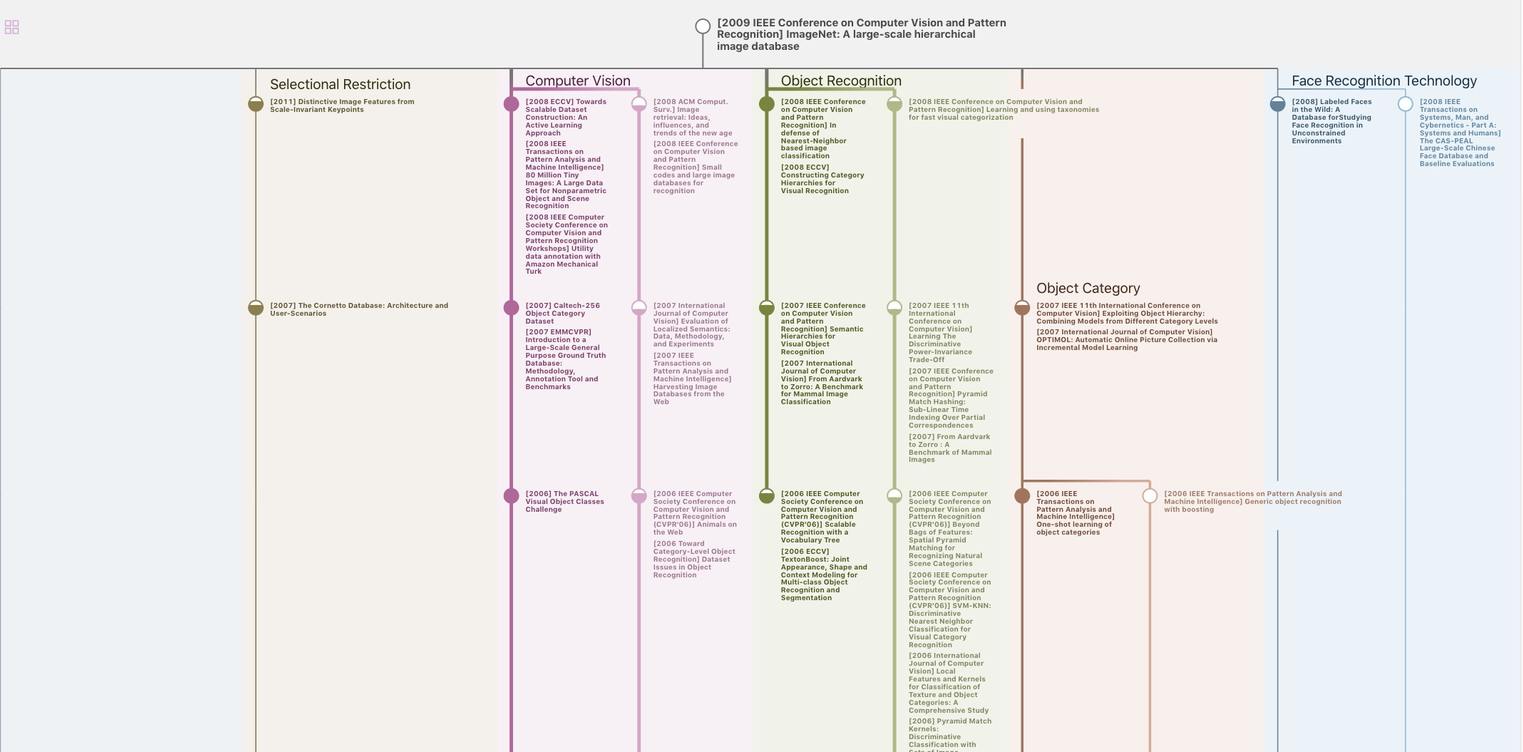
生成溯源树,研究论文发展脉络
Chat Paper
正在生成论文摘要