Web-Informed-Augmented Fake News Detection Model Using Stacked Layers of Convolutional Neural Network and Deep Autoencoder
MATHEMATICS(2023)
摘要
Today, fake news is a growing concern due to its devastating impacts on communities. The rise of social media, which many users consider the main source of news, has exacerbated this issue because individuals can easily disseminate fake news more quickly and inexpensive with fewer checks and filters than traditional news media. Numerous approaches have been explored to automate the detection and prevent the spread of fake news. However, achieving accurate detection requires addressing two crucial aspects: obtaining the representative features of effective news and designing an appropriate model. Most of the existing solutions rely solely on content-based features that are insufficient and overlapping. Moreover, most of the models used for classification are constructed with the concept of a dense features vector unsuitable for short news sentences. To address this problem, this study proposed a Web-Informed-Augmented Fake News Detection Model using Stacked Layers of Convolutional Neural Network and Deep Autoencoder called ICNN-AEN-DM. The augmented information is gathered from web searches from trusted sources to either support or reject the claims in the news content. Then staked layers of CNN with a deep autoencoder were constructed to train a probabilistic deep learning-base classifier. The probabilistic outputs of the stacked layers were used to train decision-making by staking multilayer perceptron (MLP) layers to the probabilistic deep learning layers. The results based on extensive experiments challenging datasets show that the proposed model performs better than the related work models. It achieves 26.6% and 8% improvement in detection accuracy and overall detection performance, respectively. Such achievements are promising for reducing the negative impacts of fake news on communities.
更多查看译文
关键词
fake news detection,web-informed,augmented information,misinformation,two-stage classification,deep learning,stacked learning,CNN,deep autoencoder
AI 理解论文
溯源树
样例
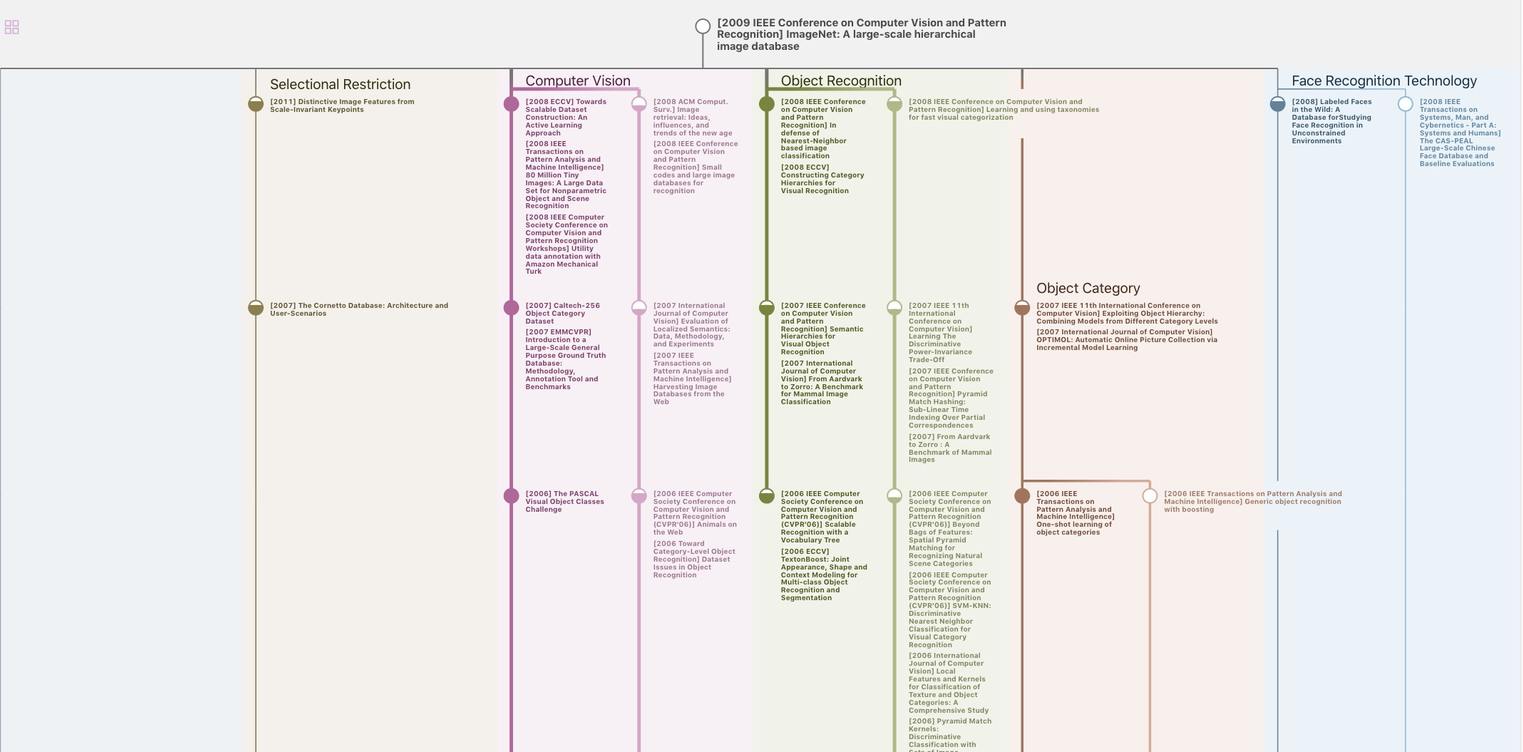
生成溯源树,研究论文发展脉络
Chat Paper
正在生成论文摘要