A Nano-QSTR model to predict nano-cytotoxicity: an approach using human lung cells data
PARTICLE AND FIBRE TOXICOLOGY(2023)
摘要
Background The widespread use of new engineered nanomaterials (ENMs) in industries such as cosmetics, electronics, and diagnostic nanodevices, has been revolutionizing our society. However, emerging studies suggest that ENMs present potentially toxic effects on the human lung. In this regard, we developed a machine learning (ML) nano-quantitative-structure-toxicity relationship (QSTR) model to predict the potential human lung nano-cytotoxicity induced by exposure to ENMs based on metal oxide nanoparticles. Results Tree-based learning algorithms (e.g., decision tree (DT), random forest (RF), and extra-trees (ET)) were able to predict ENMs’ cytotoxic risk in an efficient, robust, and interpretable way. The best-ranked ET nano-QSTR model showed excellent statistical performance with R 2 and Q 2 -based metrics of 0.95, 0.80, and 0.79 for training, internal validation, and external validation subsets, respectively. Several nano-descriptors linked to the core-type and surface coating reactivity properties were identified as the most relevant characteristics to predict human lung nano-cytotoxicity. Conclusions The proposed model suggests that a decrease in the ENMs diameter could significantly increase their potential ability to access lung subcellular compartments (e.g., mitochondria and nuclei), promoting strong nano-cytotoxicity and epithelial barrier dysfunction. Additionally, the presence of polyethylene glycol (PEG) as a surface coating could prevent the potential release of cytotoxic metal ions, promoting lung cytoprotection. Overall, the current work could pave the way for efficient decision-making, prediction, and mitigation of the potential occupational and environmental ENMs risks.
更多查看译文
关键词
Engineered nanomaterials,Computational nanotoxicology,Machine learning,Nano-QSTR,Lung nano-cytotoxicity,A549 cell line
AI 理解论文
溯源树
样例
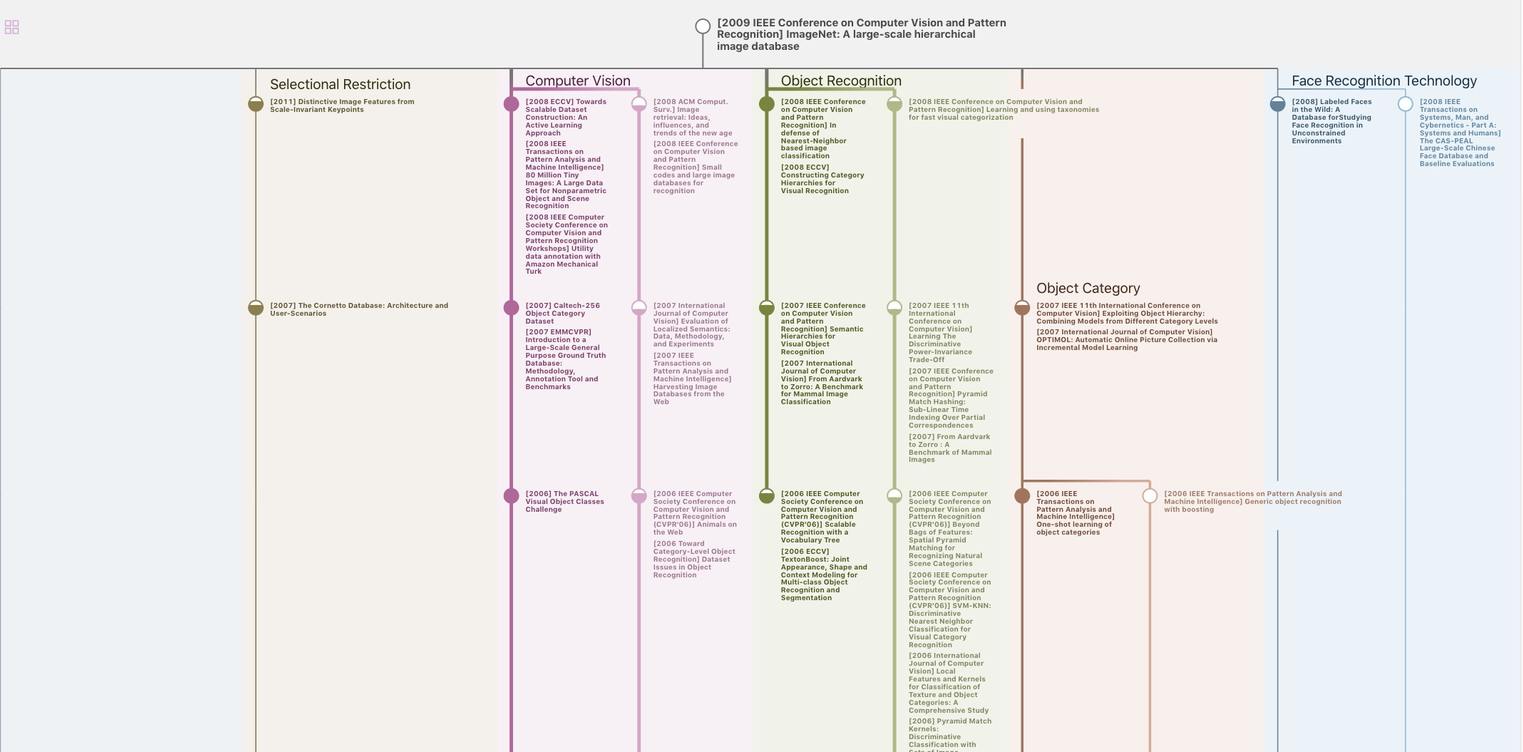
生成溯源树,研究论文发展脉络
Chat Paper
正在生成论文摘要