FluTO: Graded multi-scale topology optimization of large contact area fluid-flow devices using neural networks
ENGINEERING WITH COMPUTERS(2023)
摘要
Fluid-flow devices with low dissipation, but large contact area, are of importance in many applications. A well-known strategy to design such devices is multi-scale topology optimization (MTO), where optimal microstructures are designed within each cell of a discretized domain. Unfortunately, MTO is computationally very expensive since one must perform homogenization of the evolving microstructures, during each step of the optimization process. Furthermore, methods to impose a desired contact area have not been pursued in MTO. Here, we propose a graded multiscale topology optimization for minimizing the dissipation in fluid-flow devices, subject to a desired contact area. Several pre-selected, but size-parameterized and orientable microstructures are chosen; their constitutive tensors and contact areas are pre-computed at a finite number of sizes. Then, during optimization, a simple interpolation is used to significantly reduce the computation while retaining many of the benefits of MTO. The algorithm allows for continuous switching between microstructures during optimization, but prevents mixing through penalization. The optimization is carried out using a neural network (NN) since: (1) the NN implicitly guarantees the partition of unity, i.e., ensures that the net volume fraction of microstructures in each cell is unity, (2) the number of design variables is only weakly dependent of the number of microstructure used, (3) it supports automatic differentiation, thereby eliminating manual sensitivity analysis, and (4) one can perform topology optimization at a coarser scale, and then extract a high-resolution design via a simple post-processing step. Several numerical results are presented to illustrate the proposed framework. Graphical abstract Given a set of candidate microstructures and a fluid topology optimization problem, a neural network (NN) selects appropriate microstructures, optimizes their size and orientation to produce a graded multi-scale design.
更多查看译文
关键词
optimization,neural networks,large contact area,multi-scale,fluid-flow
AI 理解论文
溯源树
样例
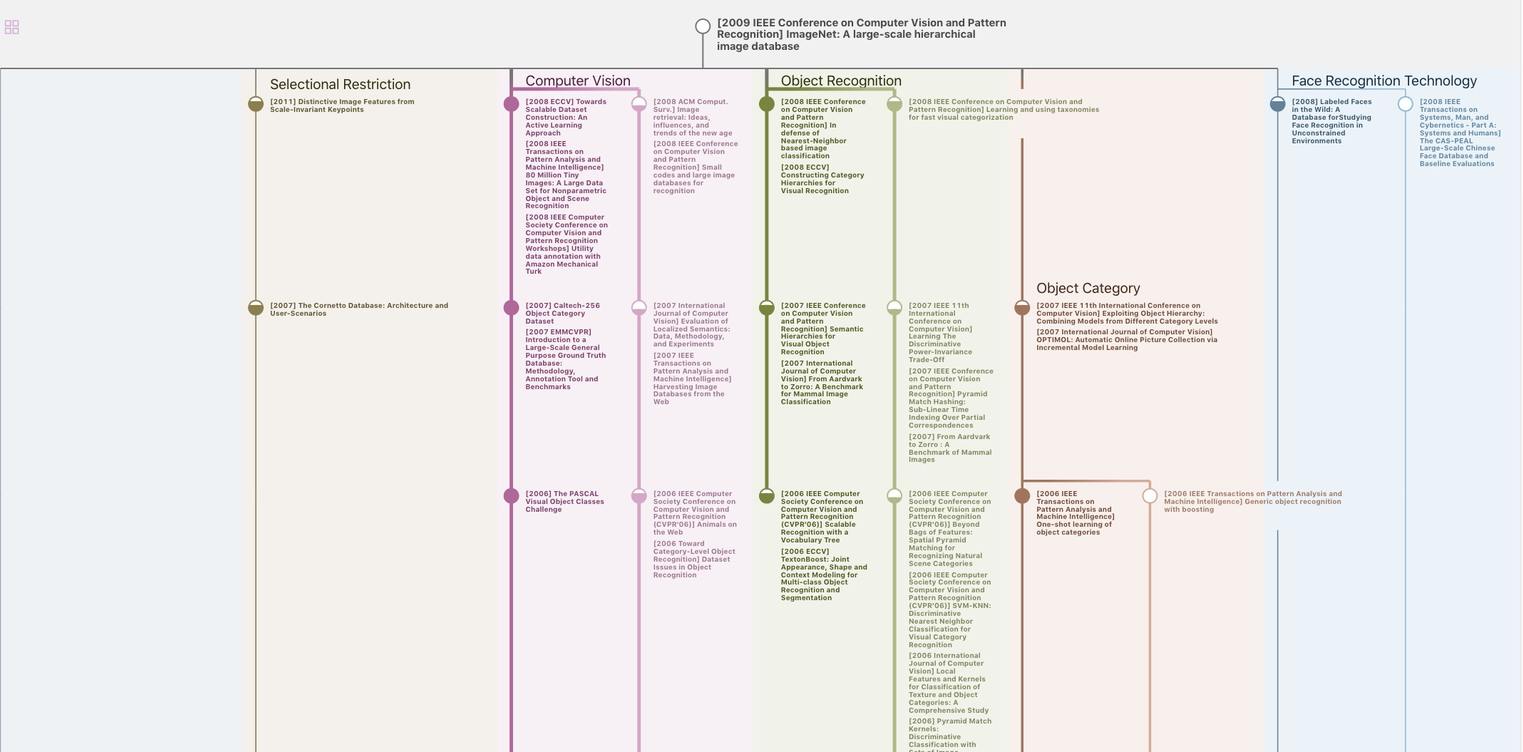
生成溯源树,研究论文发展脉络
Chat Paper
正在生成论文摘要