An enhanced monthly runoff forecasting using least squares support vector machine based on Harris hawks optimization and secondary decomposition
EARTH SCIENCE INFORMATICS(2023)
摘要
Accurate and reliable monthly runoff predictions are crucial for dispatching, allocation, and planning management of water resources. This research provides a hybrid forecasting model to increase the precision of monthly runoff predictions. Firstly, a series of intrinsic mode functions (IMF) and residual values are obtained from raw monthly runoff time series by applying complete ensemble empirical mode decomposition with adaptive noise (CEEMDAN). Secondly, variational mode decomposition (VMD) is used to perform a secondary decomposition of high-frequency IMFs. Thirdly, to determine the input–output relationships for all IMFs, Harris Hawks Optimization (HHO) algorithm is used to optimize least squares support vector machine (LSSVM) model. Finally, each IMF output is superimposed and reconstructed to obtain the final result. Five evaluation indicators are utilized to evaluate the effectiveness of the proposed hybrid model on monthly runoff data from Manwan and Hongjiadu Hydropowers in China. MAE, RMSE, MAPE, NSEC, and R of CEEMDAN-VMD-HHO-LSSVM model are 103.25, 137.29, 10.84, 0.98, and 0.99 in Manwan Hydropower and 18.28, 23.58, 28.49, 0.97 and 0.98 in Hongjiadu Hydropower, respectively. The five performance evaluation indicators of the proposed model exhibit excellent results when compared to those of other benchmarking models, demonstrating that the secondary decomposition can successfully extract the complex runoff sequence information so as to significantly increase the hybrid model's prediction accuracy.
更多查看译文
关键词
Monthly runoff forecast,Complete ensemble empirical mode decomposition with adaptive noise,Variational modal decomposition,Secondary decomposition,Harris hawks optimization algorithm,Least squares support vector machine
AI 理解论文
溯源树
样例
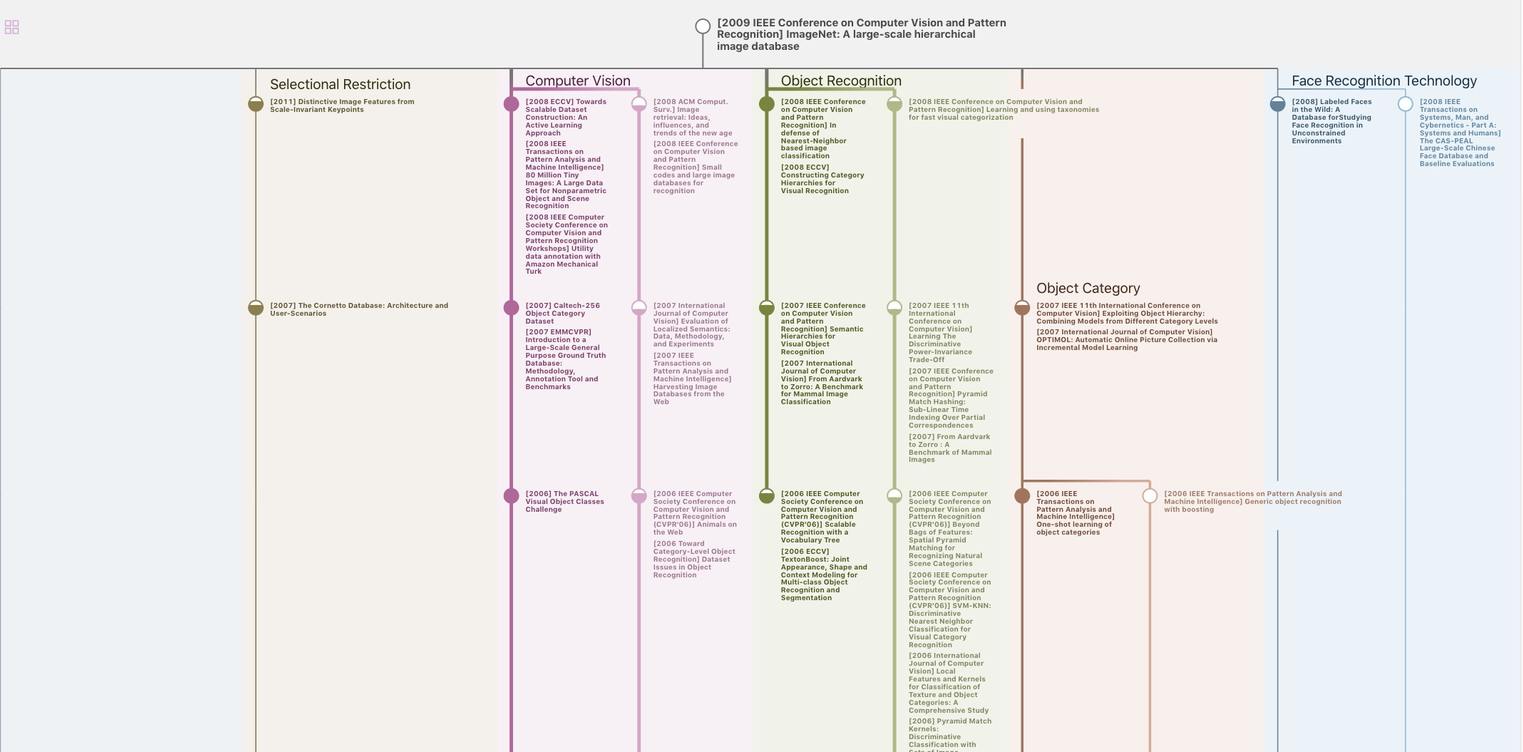
生成溯源树,研究论文发展脉络
Chat Paper
正在生成论文摘要