Harnessing Data Augmentation and Normalization Preprocessing to Improve the Performance of Chemical Reaction Predictions of Data-Driven Model
POLYMERS(2023)
摘要
As a template-free, data-driven methodology, the molecular transformer model provides an alternative by which to predict the outcome of chemical reactions and design the route of the retrosynthetic plane in the field of organic synthesis and polymer chemistry. However, in consideration of the small datasets of chemical reactions, the data-driven model suffers from the difficulty of low accuracy in the prediction tasks of chemical reactions. In this contribution, we integrate the molecular transformer model with the strategies of data augmentation and normalization preprocessing to accomplish the three tasks of chemical reactions, including the forward predictions of chemical reactions, and single-step retrosynthetic predictions with and without the reaction classes. It is clearly demonstrated that the prediction accuracy of the molecular transformer model can be significantly raised by the use of proposed strategies for the three tasks of chemical reactions. Notably, after the introduction of the 40-level data augmentation and normalization preprocessing, the top-1 accuracy of the forward prediction increases markedly from 71.6% to 84.2% and the top-1 accuracy of the single-step retrosynthetic prediction with additional reaction class increases from 53.2% to 63.4%. Furthermore, it is found that the superior performance of the data-driven model originates from the correction of the grammatical errors of the SMILES strings, especially for the case of the reaction classes with small datasets.
更多查看译文
关键词
chemical reaction,retrosynthesis,data augmentation,machine learning,molecular transformer model
AI 理解论文
溯源树
样例
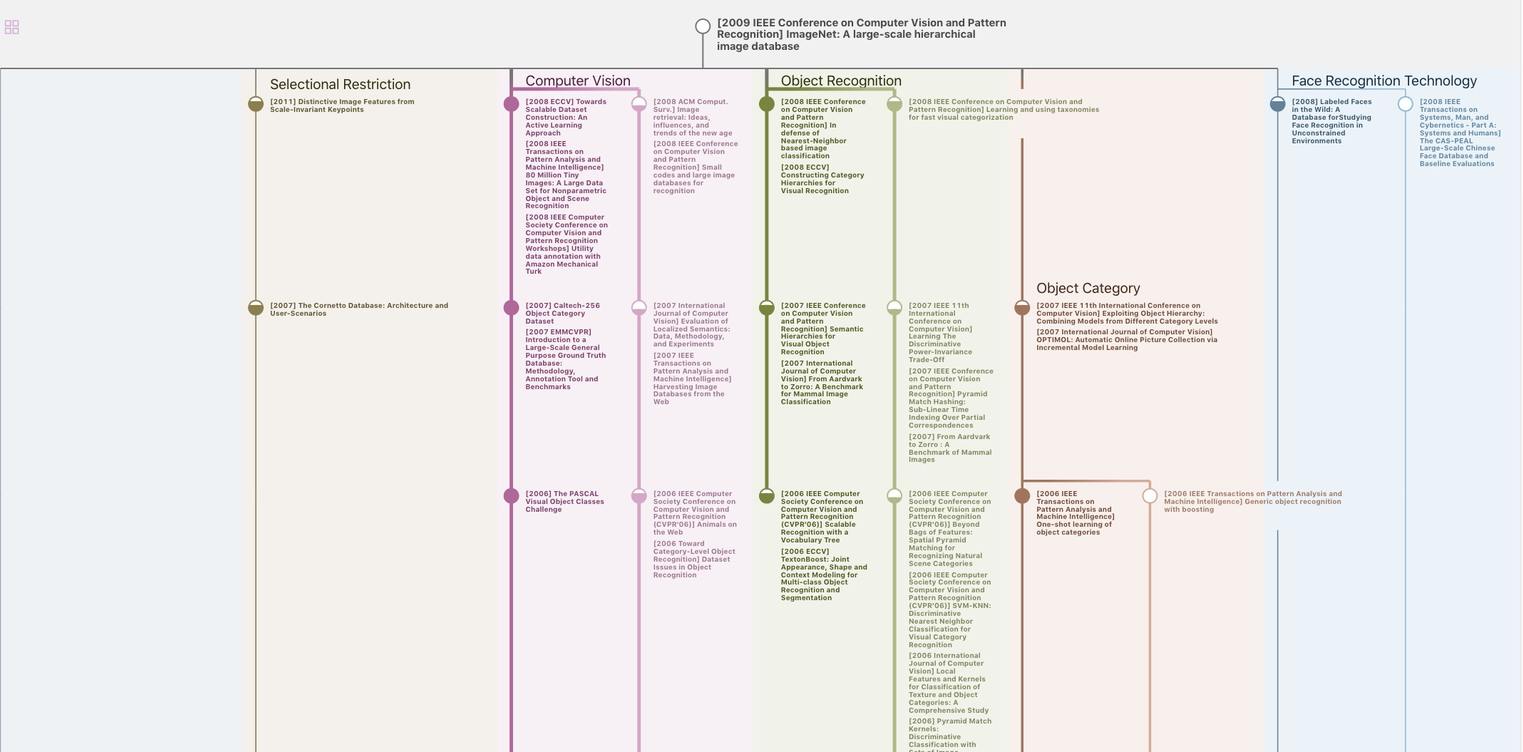
生成溯源树,研究论文发展脉络
Chat Paper
正在生成论文摘要