A new method for multispace analysis of multidimensional social exclusion
GEOJOURNAL(2023)
摘要
Social phenomena are multidimensional and dependent on geographic space. Numerous methods are capable of representing multidimensional social phenomena through a composite indicator. Among these methods, principal component analysis (PCA) is the most used when considering the geographical perspective. However, the composite indicators built by the method are sensitive to outliers and dependent on the input data, implying informational loss and specific eigenvectors that make multi-space–time comparisons impossible. This research proposes a new method to overcome these problems: the Robust Multispace PCA. The method incorporates the following innovations. The sub-indicators are weighted according to their conceptual importance in the multidimensional phenomenon. The non-compensatory aggregation of these sub-indicators guarantees the function of the weights as of relative importance. Aggregating indicators in dimensions balances the weight structure of dimensions in the composite indicator. A new scale transformation function that eliminates outliers and allows multispatial comparison reduces by 1.52 times the informational loss of the composite indicator of social exclusion in eight cities' urban areas. The Robust Multispace-PCA has a high potential for appropriation by researchers and policymakers, as it is easy to follow, offers more informative and accurate representations of multidimensional social phenomena, and favors the development of policies at multiple geographic scales.
更多查看译文
关键词
Social exclusion,Multidimensional analysis,Composite indicators,Spatial analysis,Principal component analysis
AI 理解论文
溯源树
样例
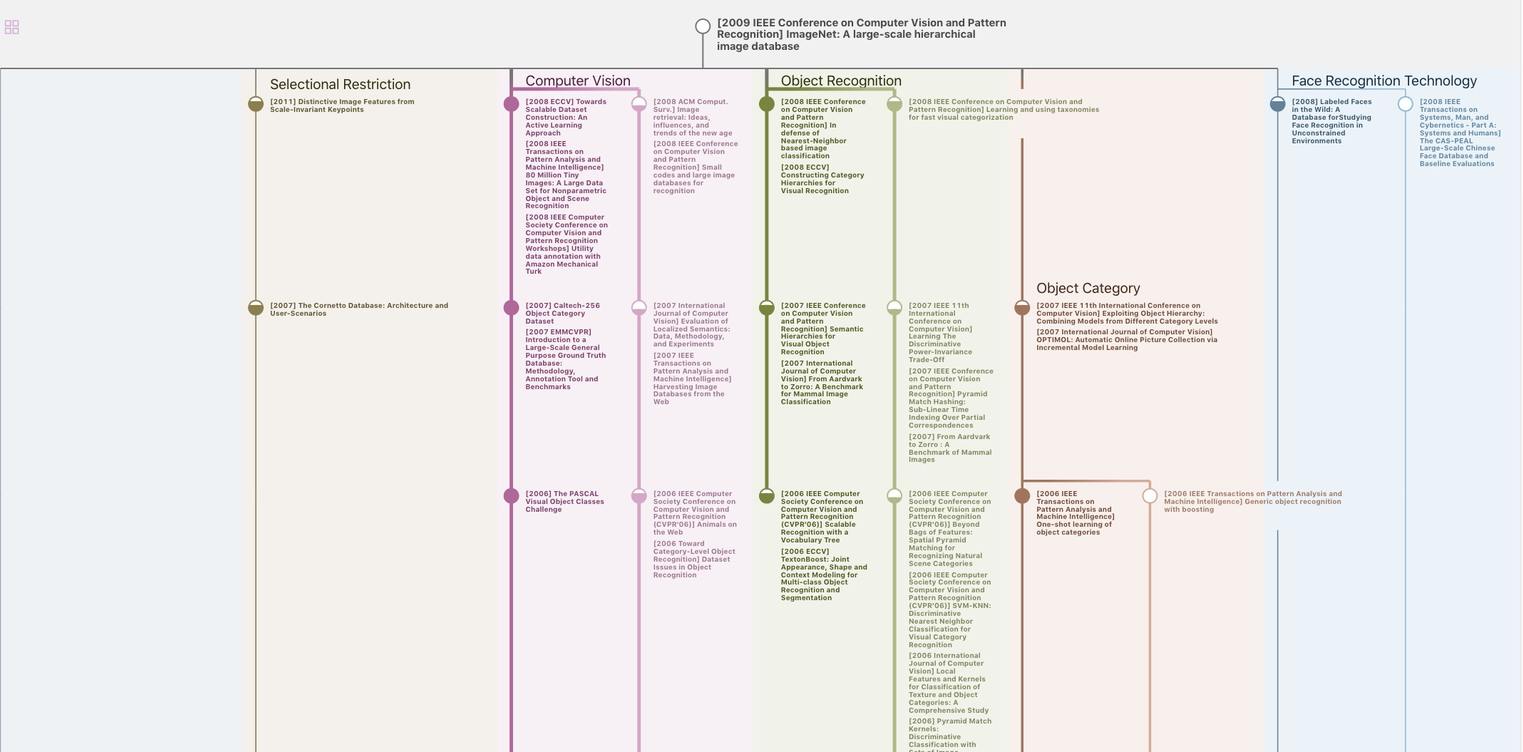
生成溯源树,研究论文发展脉络
Chat Paper
正在生成论文摘要