Damage detection with ultrasonic guided waves using machine learning and aggregated baselines
STRUCTURAL HEALTH MONITORING-AN INTERNATIONAL JOURNAL(2024)
摘要
In guided wave (GW)-based structural health monitoring (SHM), ultrasonic elastic waves are used to detect damages in structures by comparing the acquired signals with those acquired before defect formation. Making the SHM system automatic, especially for similar structures, such as turbine blades, is rather challenging. The high sensitivity of GWs to environmental and operational conditions, the variabilities due to sensor positioning, sensor coupling, and material variability in composites limit the baseline application. This work presents a machine learning (ML)-based damage detection method using aggregated baselines independent of their damaged states to enhance the generalization capability of ML algorithms by considering similar structures' variabilities. The methodology relies on feature extraction from raw GW signals and training classification algorithms (e.g., kernel machines, ensemble methods, and neural networks). Two experimental data sets on composite panels are used. The first experimental data set of 45 composite panels is used to validate the approach by considering the aforementioned inter-specimen variabilities. Half of the 45 panels provide pristine data, and the rest provide damaged data so that the same sample is present in the training or test set but never in both. High classification performance is obtained, demonstrating that the classifier has successfully learned to recognize defect signatures despite the influence of the variabilities linked to the multiple instrumented specimens. The second experimental data set of 1 composite panel with temperature variation is used. Good classification performance is obtained without using baseline correction methods.
更多查看译文
关键词
Ultrasonic guided wave SHM,kernel machines,ensemble methods,deep learning,temperature variation,composite materials
AI 理解论文
溯源树
样例
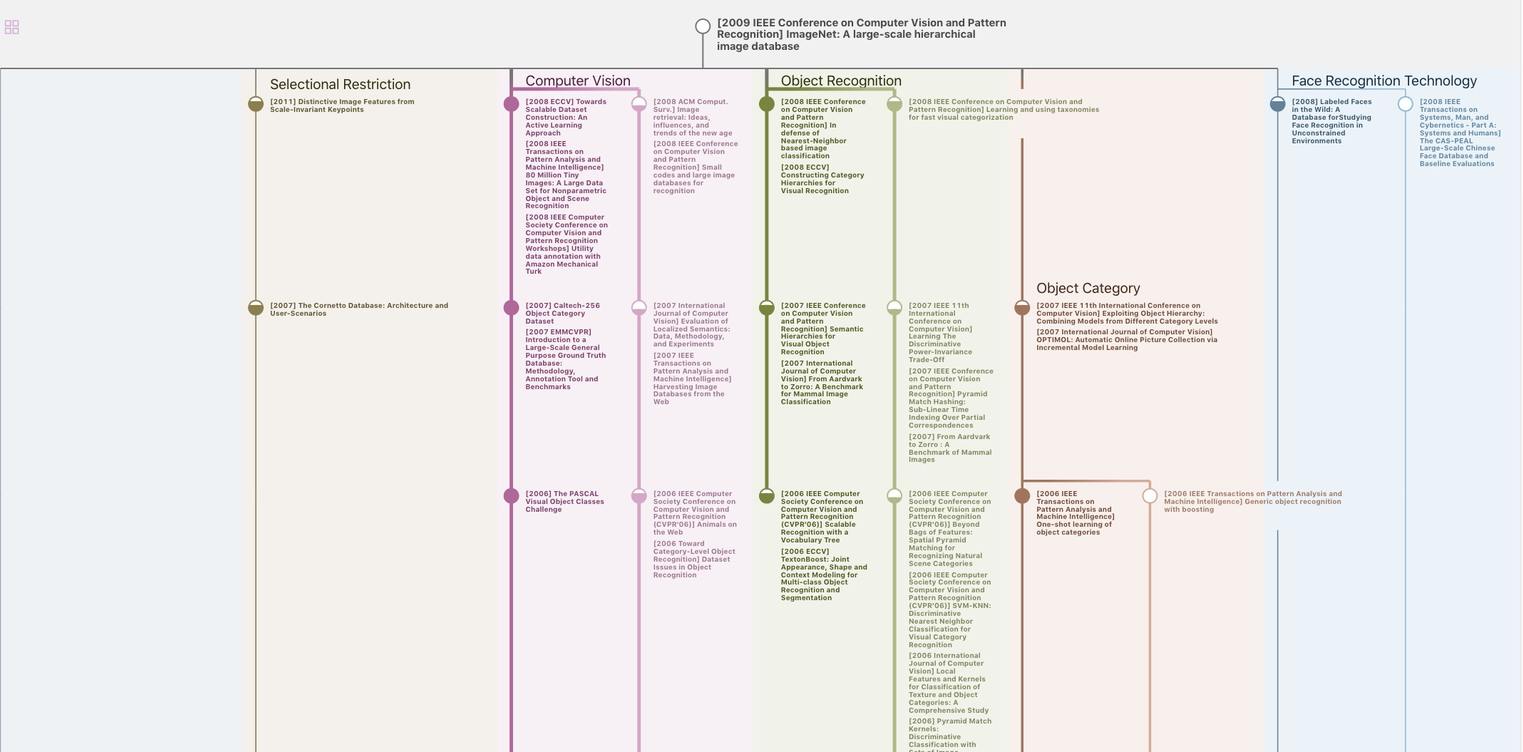
生成溯源树,研究论文发展脉络
Chat Paper
正在生成论文摘要