Multidisciplinary Topology Optimization Using Generative Adversarial Networks for Physics-Based Design Enhancement
JOURNAL OF MECHANICAL DESIGN(2023)
摘要
The computational cost of traditional gradient-based topology optimization is amplified for multidisciplinary design optimization (MDO) problems, most notably when coupling between physics disciplines is accounted for. To alleviate this, we investigate new methods and applications of generative adversarial networks (GANs) as a surrogate for MDO. Accepting physical fields from each physics discipline as input, the trained network produces an optimal design that closely resembles that of the iterative gradient-based approach. With this model as a baseline, we introduce a novel architecture that performs physics-based design enhancement of optimal single-physics designs to produce multiphysics designs. By providing the network with boundary conditions from a secondary physics discipline, we obtain multiphysics structures while avoiding the need for costly coupled multiphysics analysis, thereby generating significant savings in computational effort. We demonstrate our approach by designing a series of structures optimized for both thermal and elastic performance. With the physics-based design enhancement GAN, we obtain thermoelastic structures that outperform those produced by the baseline multiphysics GAN architecture.
更多查看译文
关键词
generative adversarial networks,design enhancement,topology,optimization,physics-based
AI 理解论文
溯源树
样例
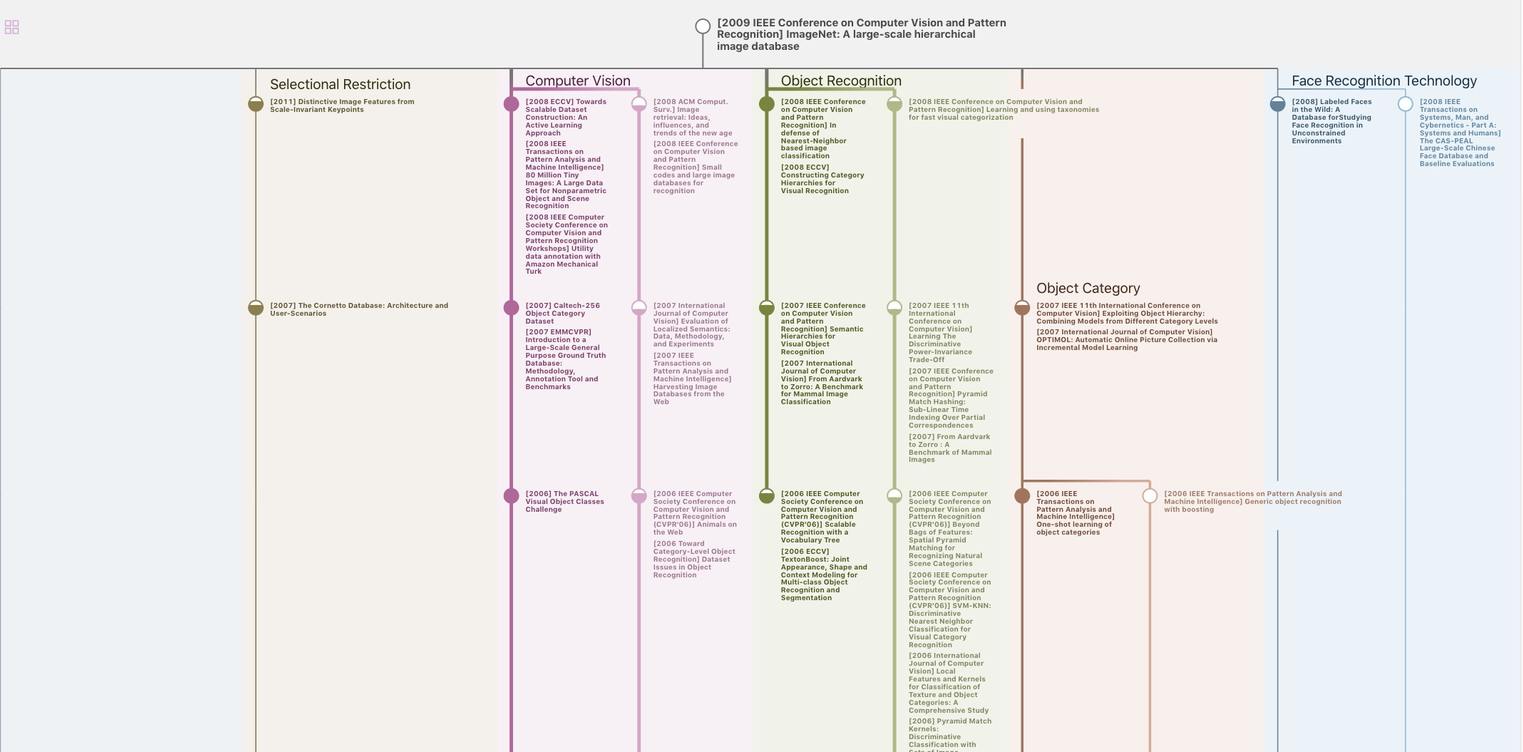
生成溯源树,研究论文发展脉络
Chat Paper
正在生成论文摘要