Self-supervised monocular depth estimation in fog
OPTICAL ENGINEERING(2023)
摘要
Self-supervised depth estimation has achieved remarkable results in sunny weather. However, in the foggy scenes, their performance is limited because of the low contrast and limited visibility caused by the fog. To address this problem, an end-to-end feature separation network for self-supervised depth estimation of fog images is proposed. We take paired clear and synthetic foggy images as input, separate the image information into interference information (illumination, fog, etc.) and invariant information (structure, texture, etc.) by a feature extractor with orthogonality loss. The invariant information is used to estimate depth. Meanwhile, similarity loss is introduced to constrain the fog image depth using the depth of the clear image as a pseudo-label, and an attention module and reconstruction loss are added to refine the output depth, so that better depth maps can be obtained. Then, real-world fog images are used for fine-tuning, which effectively reduces the domain gap between synthetic data and real data. Experiments show that our approach produces advanced results on both synthetic datasets and Cityscape datasets, demonstrating the superiority of our approach. (c) 2022 Society of Photo-Optical Instrumentation Engineers (SPIE)
更多查看译文
关键词
monocular depth estimation,fog,self-supervised
AI 理解论文
溯源树
样例
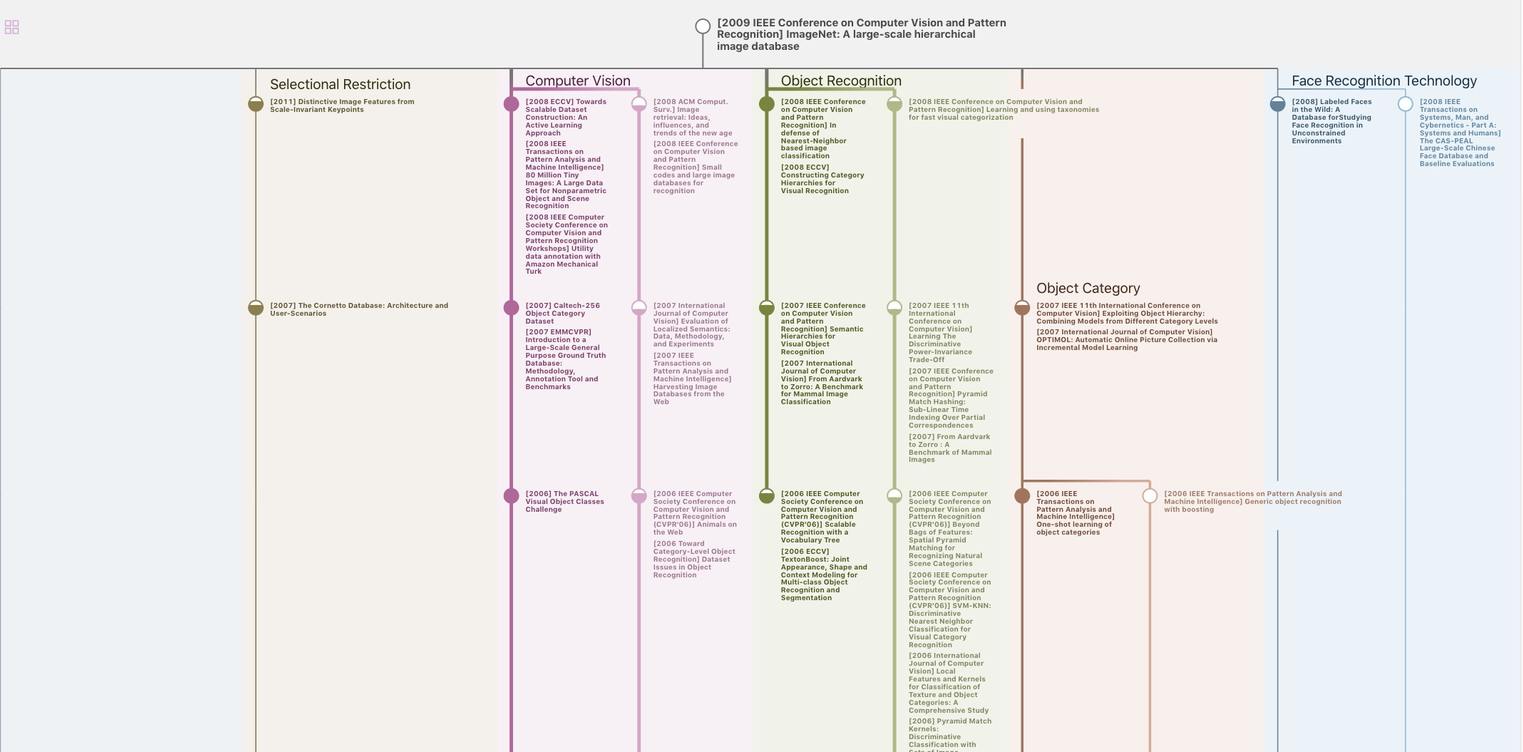
生成溯源树,研究论文发展脉络
Chat Paper
正在生成论文摘要