Self-Supervised Pretraining Based on Noise-Free Motion Reconstruction and Semantic-Aware Contrastive Learning for Human Motion Prediction
IEEE TRANSACTIONS ON EMERGING TOPICS IN COMPUTATIONAL INTELLIGENCE(2024)
摘要
Human motion prediction is to forecast future human motions based on the observed ones. Currently, a prediction model always includes an encoder to extract the features of the observed motions and a decoder for motion prediction with the extracted features. However, existing encoders have limited abilities of anti-interference and semantic attribute learning; thus, the prediction results are not fully satisfactory. To solve this problem, we propose two novel pretext tasks, i.e., noise-free motion reconstruction and semantic-aware contrastive learning, to implement self-supervised pretraining on the encoder. The former extracts the features of a noise-added motion to reconstruct a noise-free motion. This pretext task can improve the ability of anti-interference of the encoder. The latter shortens the distance between two motions with the same motion category while widening the distance between two motions from different motion categories. This pretext task enables the encoder to capture the semantic commonality of the motions with the same motion category, thereby enhancing the encoder's ability of semantic attribute learning. After implementing the self-supervised pretraining, the pretrained encoder is connected with a decoder to build a prediction model called ReLe-GCN, which is fine-tuned for human motion prediction. The results on public datasets show the high accuracies of ReLe-GCN in both short-term and long-term motion predictions.
更多查看译文
关键词
Human motion prediction,self-supervised pretraining,anti-interference,semantic attribute learning
AI 理解论文
溯源树
样例
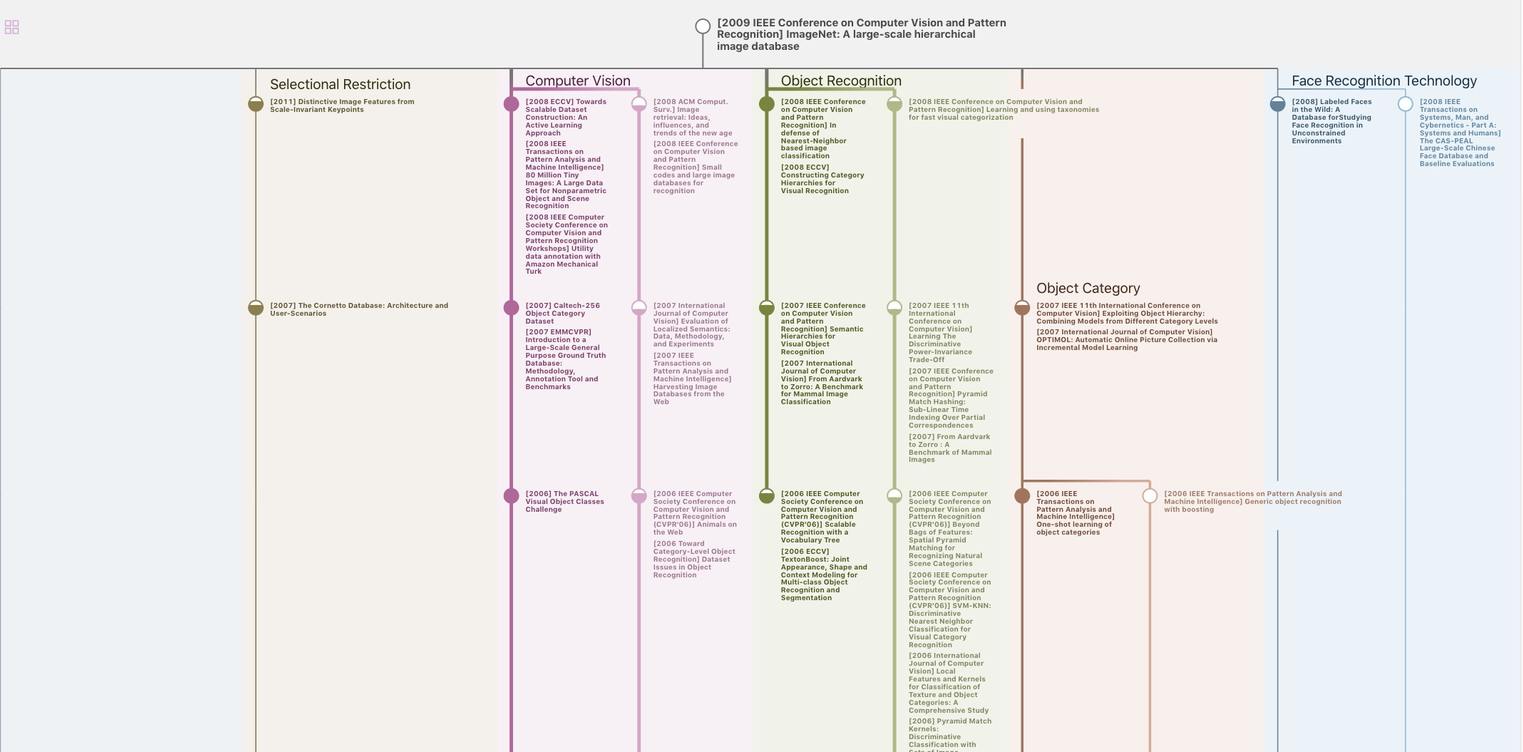
生成溯源树,研究论文发展脉络
Chat Paper
正在生成论文摘要