A Transformer-based multimodal-learning framework using sky images for ultra-short-term solar irradiance forecasting
APPLIED ENERGY(2023)
摘要
The development of solar energy is crucial to combat the global climate change and fossil energy crisis. However, the inherent uncertainty of solar power prevents its large-scale integration into power grids. Although various sky-image-derived modeling methods exist to forecast the variations of solar irradiance, few focus on fully uti-lizing the coupling correlations between sky images and historical data to improve the forecasting performance. Therefore, a novel multimodal-learning framework is proposed for forecasting global horizontal irradiance (GHI) in the ultra-short-term. First, the historical and empirically estimated clear-sky GHI are encoded by Informer. Then, the ground-based sky images are transformed into optical flow maps, which can be handled by Vision Transformer. Subsequently, a cross-modality attention method is proposed to explore the coupling correlations between the two modalities. Last, a generative decoder is used to implement multi-step forecasting. The experimental results show that the proposed method achieves a normalized root mean square error (NRMSE) of 4.28% in 10-min-ahead forecasting. Several state-of-the-art methods are also used for comparisons. The exper-imental results show that the proposed method outperforms the benchmark methods and exhibits higher ac-curacy and robustness in ultra-short-term GHI forecasting.
更多查看译文
关键词
Solar irradiance forecasting, Multimodal-learning, Transformer, Ground-based sky image
AI 理解论文
溯源树
样例
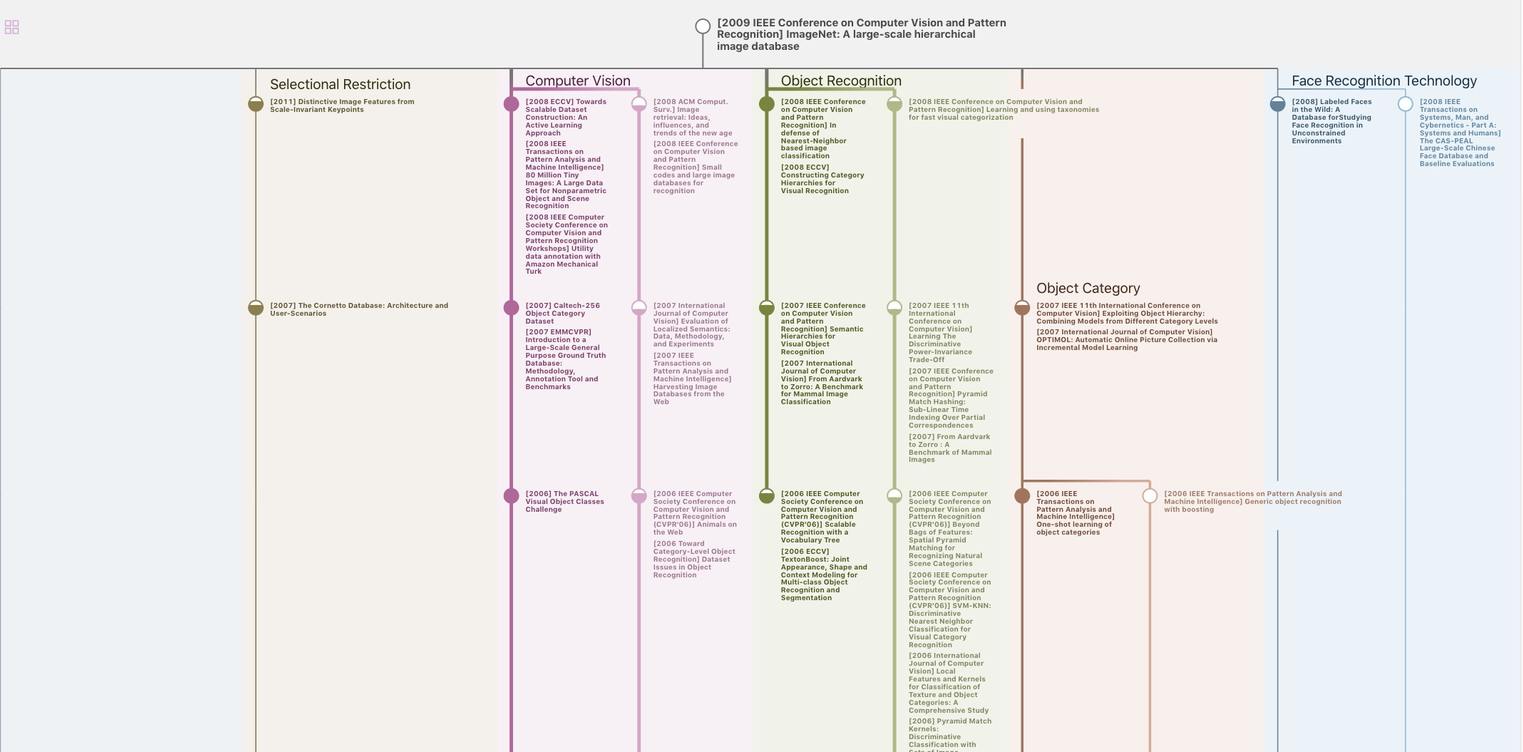
生成溯源树,研究论文发展脉络
Chat Paper
正在生成论文摘要