Deep learning-based cerebral aneurysm segmentation and morphological analysis with three-dimensional rotational angiography
JOURNAL OF NEUROINTERVENTIONAL SURGERY(2024)
摘要
BackgroundThe morphological assessment of cerebral aneurysms based on cerebral angiography is an essential step when planning strategy and device selection in endovascular treatment, but manual evaluation by human raters only has moderate interrater/intrarater reliability. MethodsWe collected data for 889 cerebral angiograms from consecutive patients with suspected cerebral aneurysms at our institution from January 2017 to October 2021. The automatic morphological analysis model was developed on the derivation cohort dataset consisting of 388 scans with 437 aneurysms, and the performance of the model was tested on the validation cohort dataset consisting of 96 scans with 124 aneurysms. Five clinically important parameters were automatically calculated by the model: aneurysm volume, maximum aneurysm size, neck size, aneurysm height, and aspect ratio. ResultsOn the validation cohort dataset the average aneurysm size was 7.9 +/- 4.6 mm. The proposed model displayed high segmentation accuracy with a mean Dice similarity index of 0.87 (median 0.93). All the morphological parameters were significantly correlated with the reference standard (all P<0.0001; Pearson correlation analysis). The difference in the maximum aneurysm size between the model prediction and reference standard was 0.5 +/- 0.7 mm (mean +/- SD). The difference in neck size between the model prediction and reference standard was 0.8 +/- 1.7 mm (mean +/- SD). ConclusionThe automatic aneurysm analysis model based on angiography data exhibited high accuracy for evaluating the morphological characteristics of cerebral aneurysms.
更多查看译文
关键词
aneurysm,angiography,intervention
AI 理解论文
溯源树
样例
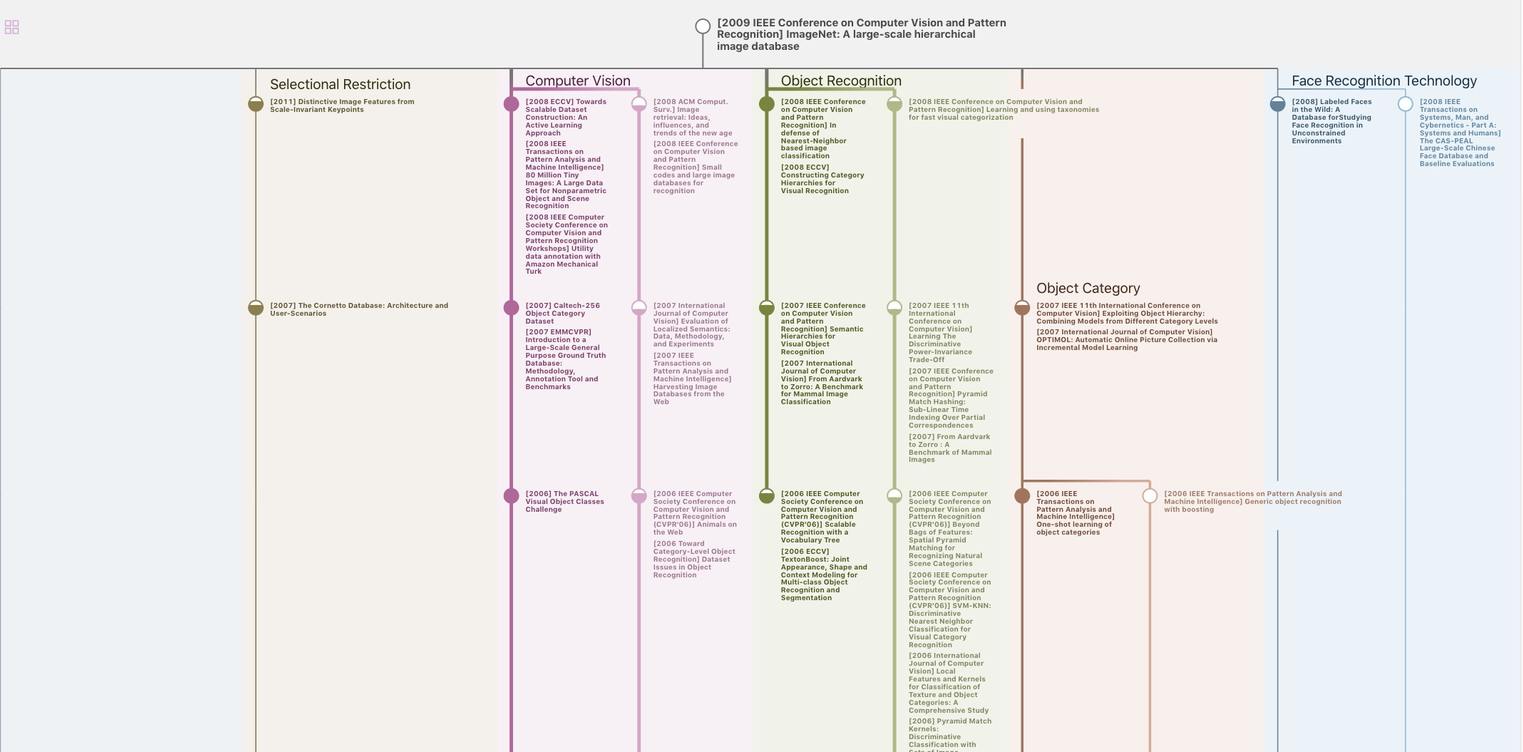
生成溯源树,研究论文发展脉络
Chat Paper
正在生成论文摘要