Molecular Identification from AFM Images Using the IUPAC Nomenclature and Attribute Multimodal Recurrent Neural Networks
ACS APPLIED MATERIALS & INTERFACES(2023)
摘要
Spectroscopic methods-like nuclear magnetic resonance, mass spectrometry, X-ray diffraction, and UV/visible spectroscopies-applied to molecular ensembles have so far been the workhorse for molecular identification. Here, we propose a radically different chemical characterization approach, based on the ability of noncontact atomic force microscopy with metal tips functionalized with a CO molecule at the tip apex (referred as HR-AFM) to resolve the internal structure of individual molecules. Our work demonstrates that a stack of constant-height HR-AFM images carries enough chemical information for a complete identification (structure and composition) of quasiplanar organic molecules, and that this information can be retrieved using machine learning techniques that are able to disentangle the contribution of chemical composition, bond topology, and internal torsion of the molecule to the HR-AFM contrast. In particular, we exploit multimodal recurrent neural networks (M-RNN) that combine convolutional neural networks for image analysis and recurrent neural networks to deal with language processing, to formulate the molecular identification as an imaging captioning problem. The algorithm is trained using a data set-which contains almost 700,000 molecules and 165 million theoretical AFM images-to produce as final output the IUPAC name of the imaged molecule. Our extensive test with theoretical images and a few experimental ones shows the potential of deep learning algorithms in the automatic identification of molecular compounds by AFM. This achievement supports the development of on-surface synthesis and overcomes some limitations of spectroscopic methods in traditional solution-based synthesis.
更多查看译文
关键词
atomic force microscopy, molecular identification, deep learning, neural network, image captioning, density functional theory
AI 理解论文
溯源树
样例
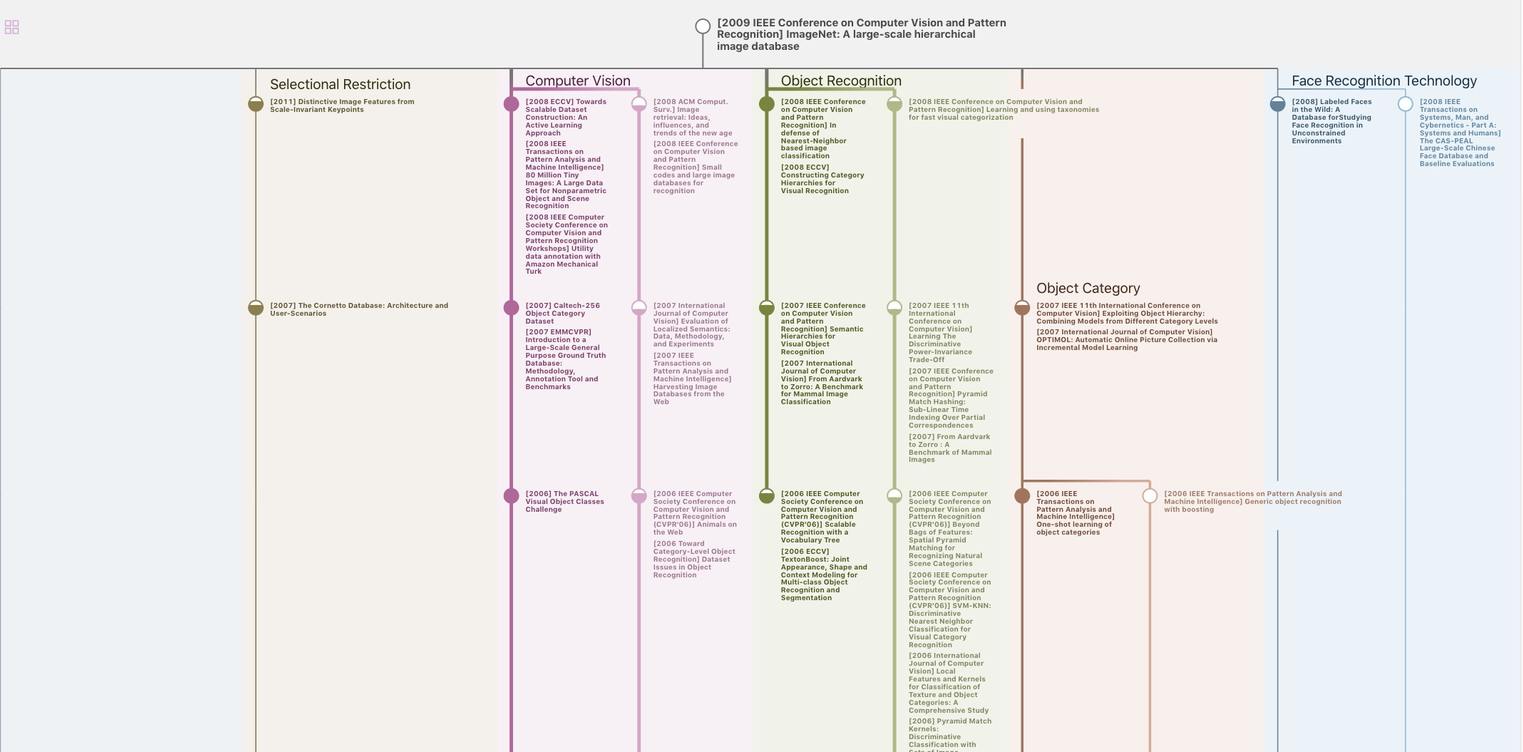
生成溯源树,研究论文发展脉络
Chat Paper
正在生成论文摘要