Latent low-rank representation sparse regression model with symmetric constraint for unsupervised feature selection
IET IMAGE PROCESSING(2023)
摘要
Unsupervised feature selection is a dimensionality reduction method and has been widely used as an important and indispensable preprocessing step in many tasks. However, real-world data are not only high-dimensional, but also have intrinsic correlations between data points, which are not fully utilized in feature selection. Furthermore, real-world data usually inevitably contain noise or outliers. In order to select features from data more effectively, a sparse regression model based on latent low-rank representation with the symmetric constraint for unsupervised feature selection is proposed. With the coefficient matrix of non-negative symmetric low-rank representation, an affinity matrix characterized by the correlation relationship between data points is adaptively obtained, which reveals the intrinsic geometric relationship, global structure, and discrimination of data points. A latent representation of all data points obtained from this affinity matrix is employed as a pseudo-label, and feature selection is carried out by sparse linear regression. This method performs feature selection in the learned latent space instead of the original data space. An alternating iteration algorithm is designed to solve the proposed model, and its effectiveness and efficiency are verified on several benchmark data sets.
更多查看译文
关键词
face recognition,feature selection,image representation,pattern clustering,regression analysis
AI 理解论文
溯源树
样例
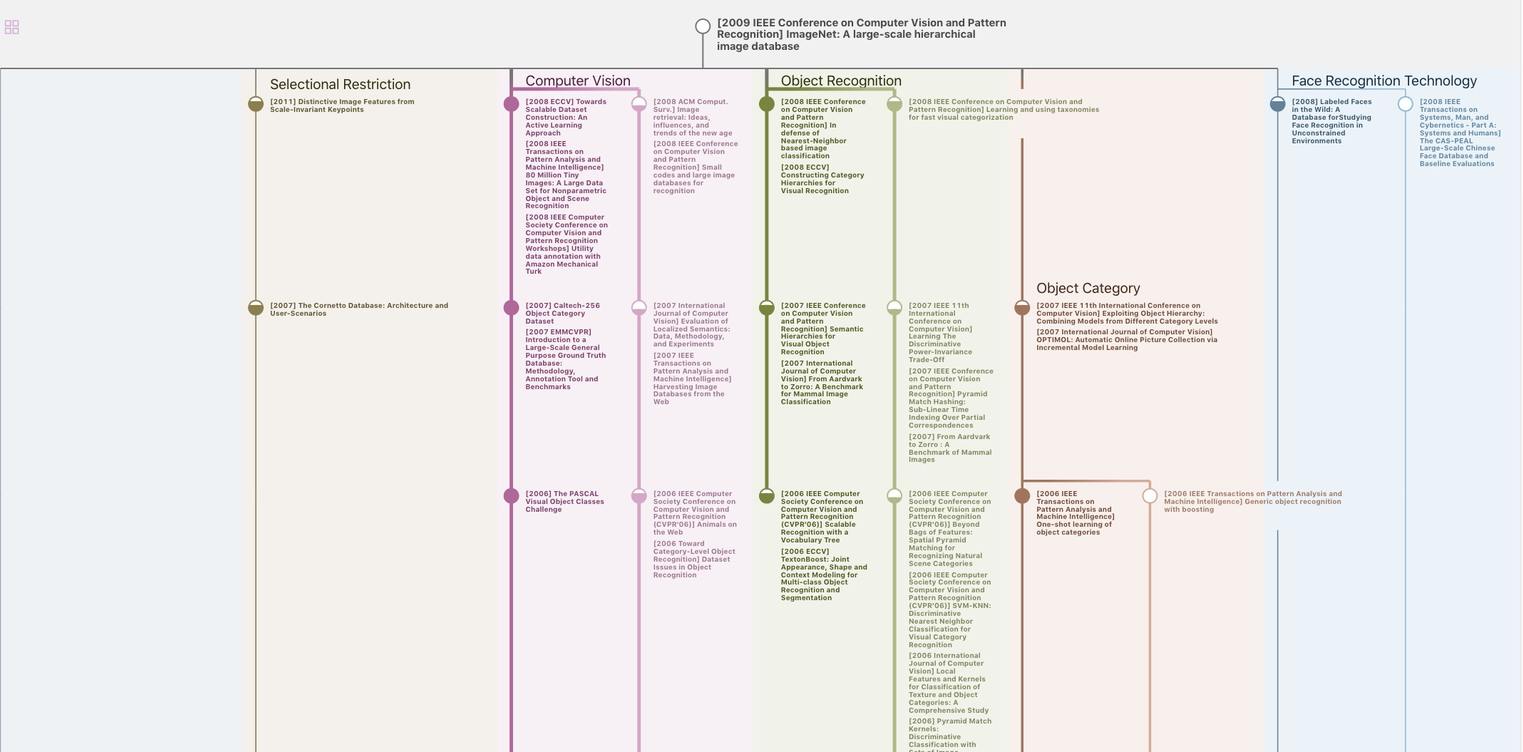
生成溯源树,研究论文发展脉络
Chat Paper
正在生成论文摘要