Forecasting residential electricity consumption using the novel hybrid model
ENERGY AND BUILDINGS(2023)
摘要
The accuracy of power load forecasting plays an important role in the development of the economy and the promotion of energy consumption and transformation. Aiming at the strong fluctuation and variability mecha-nism of electricity consumption, this paper proposes a novel hybrid method with the Long Short-term Memory (LSTM) on the dual optimization of particle swarm algorithm and butterfly algorithm, namely the EWT-MOLSTM-SVR model. Adaptive signal decomposition Empirical Wavelet Transform (EWT) decomposes the fluctuating electricity data into different modes, and uses the frequency domain analysis of signal analysis to classify and forecast. LSTM alone reveals that the advantage of the data variability mechanism is not significant. Particle Swarm Optimization (PSO) is used for law control, and Butterfly Optimization Algorithm (BOA) is used for parameter promotion to jointly optimize the expression effect. The PSO optimizes the LSTM by improving the convergence speed and global search ability of the BOA, and the optimal hyperparameters could be searched for forecast so that the accuracy of the forecast was improved. This paper adopts the data of American residential electricity data for empirical analysis. The experimental results show that the model is significantly better than Recurrent Neural Network (RNN), General Regression Neural Network (GRNN), PSO-SVM, and other models, which has a good effect on improving the forecast accuracy. The hybrid model reveals the fluctuation mechanism and variability of electricity data, deeply analyzes the economic behavior of electricity, and provides a new method for exploring residential energy consumption and optimizing residential buildings.
更多查看译文
关键词
Short-term load forecasting,Long Short-Term Memory (LSTM),Particle Swarm Optimization (PSO),Butterfly Optimization Algorithm (BOA),Empirical Wavelet Transform (EWT)
AI 理解论文
溯源树
样例
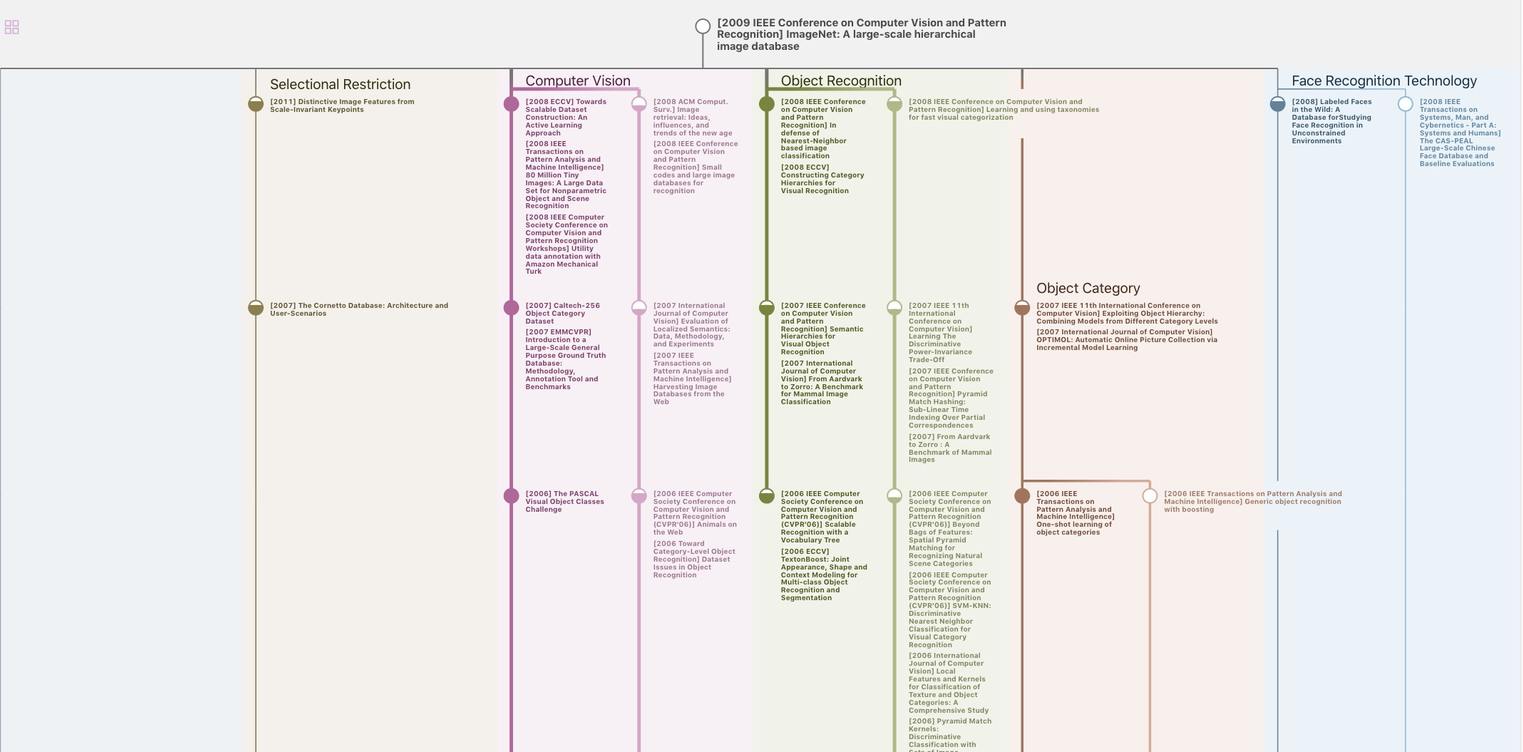
生成溯源树,研究论文发展脉络
Chat Paper
正在生成论文摘要