A machine learning-based data augmentation strategy for structural damage classification in civil infrastructure system
JOURNAL OF CIVIL STRUCTURAL HEALTH MONITORING(2023)
摘要
With the explosive growth of available data collected from various sensors and increasingly powerful computing resources, recent advanced in machine learning (ML) and other data-driven techniques have yielded transformative results in structural health monitoring (SHM). A common challenge in applying ML algorithms to SHM problems in a supervised learning manner is that the ML models need a comprehensive and systematic training process, typically requiring large datasets representing structures under different damage conditions, a requirement that is usually not met when dealing with real-life civil structures. In this study, we propose a novel data augmentation strategy within a vibration-based SHM framework, based on a conditional variational autoencoder (CVAE) to generate additional data samples of the power cepstral coefficients of the structural acceleration response in various damage conditions. The developed CVAE architecture can be trained to model various statistical distributions of the power cepstral coefficients simultaneously by incorporating newly defined conditional variables, where the decoder of the trained CVAE is subsequently separated and used to generate new samples for the data augmentation. A new type of the power cepstral coefficients is used to boost the performance and robustness of the data augmentation and consequently of the subsequent damage classification task. The augmented training datasets can be used to better train a probabilistic linear discriminant analysis (PLDA) model that can be employed for structural damage identification and classification in both supervised and unsupervised learning strategies. The proposed method has been validated by numerical simulations and experimental data.
更多查看译文
关键词
Variational autoencoders,Data augmentation,Power cepstral coefficients,Structural health monitoring
AI 理解论文
溯源树
样例
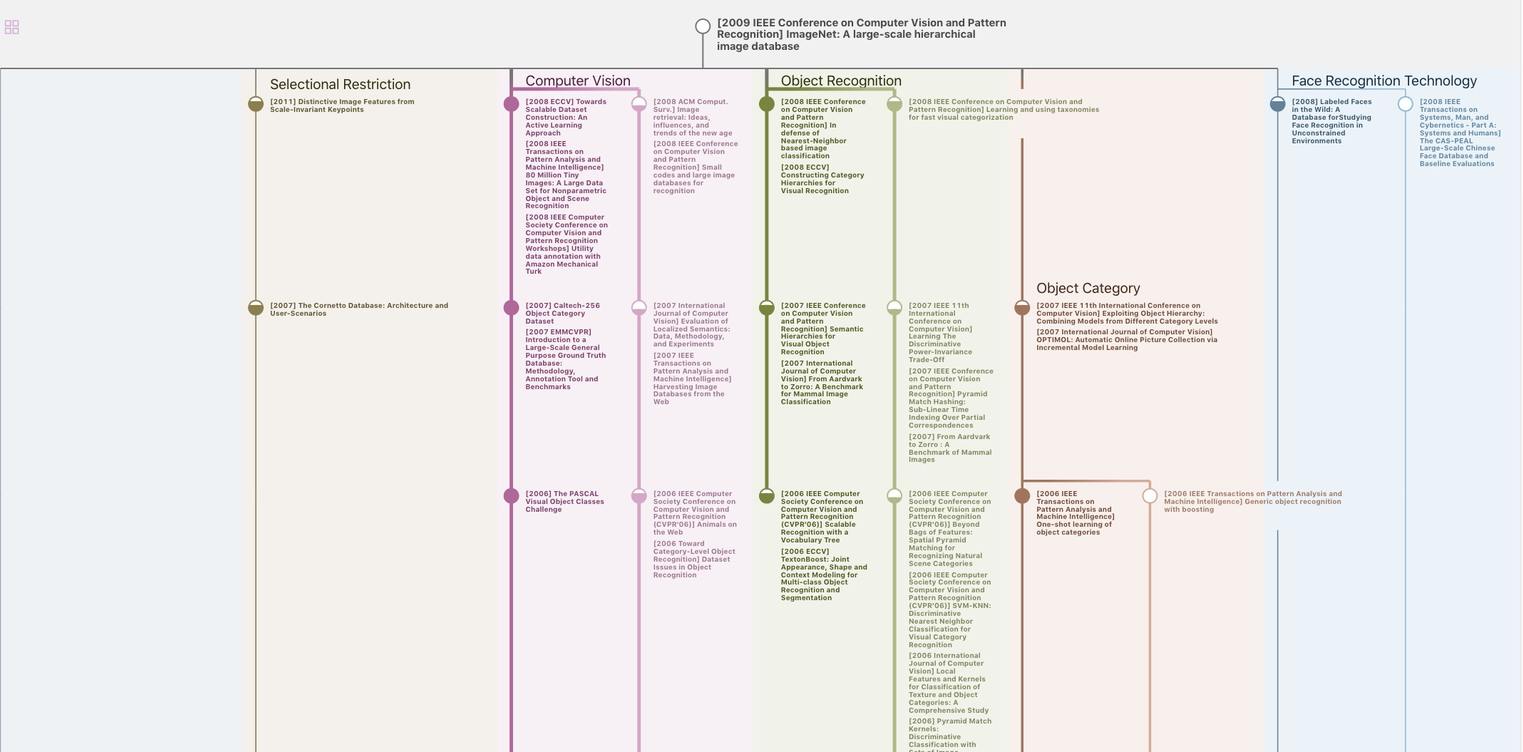
生成溯源树,研究论文发展脉络
Chat Paper
正在生成论文摘要