Machine learning-based approach for fatigue crack growth prediction using acoustic emission technique
FATIGUE & FRACTURE OF ENGINEERING MATERIALS & STRUCTURES(2023)
摘要
In this study, a general machine learning-based approach is proposed for fatigue crack growth rate (FCGR) prediction using multivariate acoustic emission (AE) online monitoring data. To improve the prediction accuracy, a backpropagation neural network optimized by genetic algorithm (GA-BPNN) is developed to describe the intricate link between the FCGR and multivariate input data. Several conventional machine learning models and the traditional FCGR prediction method based on the linear relationship between AE energy rate and FCGR are also used to examine the effectiveness of the proposed GA-BPNN model. The results indicate that the developed GA-BPNN exhibits higher accuracy and superior adaptability in predicting FCGR from unseen data than other methods. The findings of this study will provide a strategy for developing and optimizing a machine learning solution for FCGR prediction based on AE monitoring data and also aid in determining the most suitable feature for AE monitoring studies.
更多查看译文
关键词
acoustic emission, fatigue crack growth, fatigue life prediction, genetic algorithm, machine learning, neural network
AI 理解论文
溯源树
样例
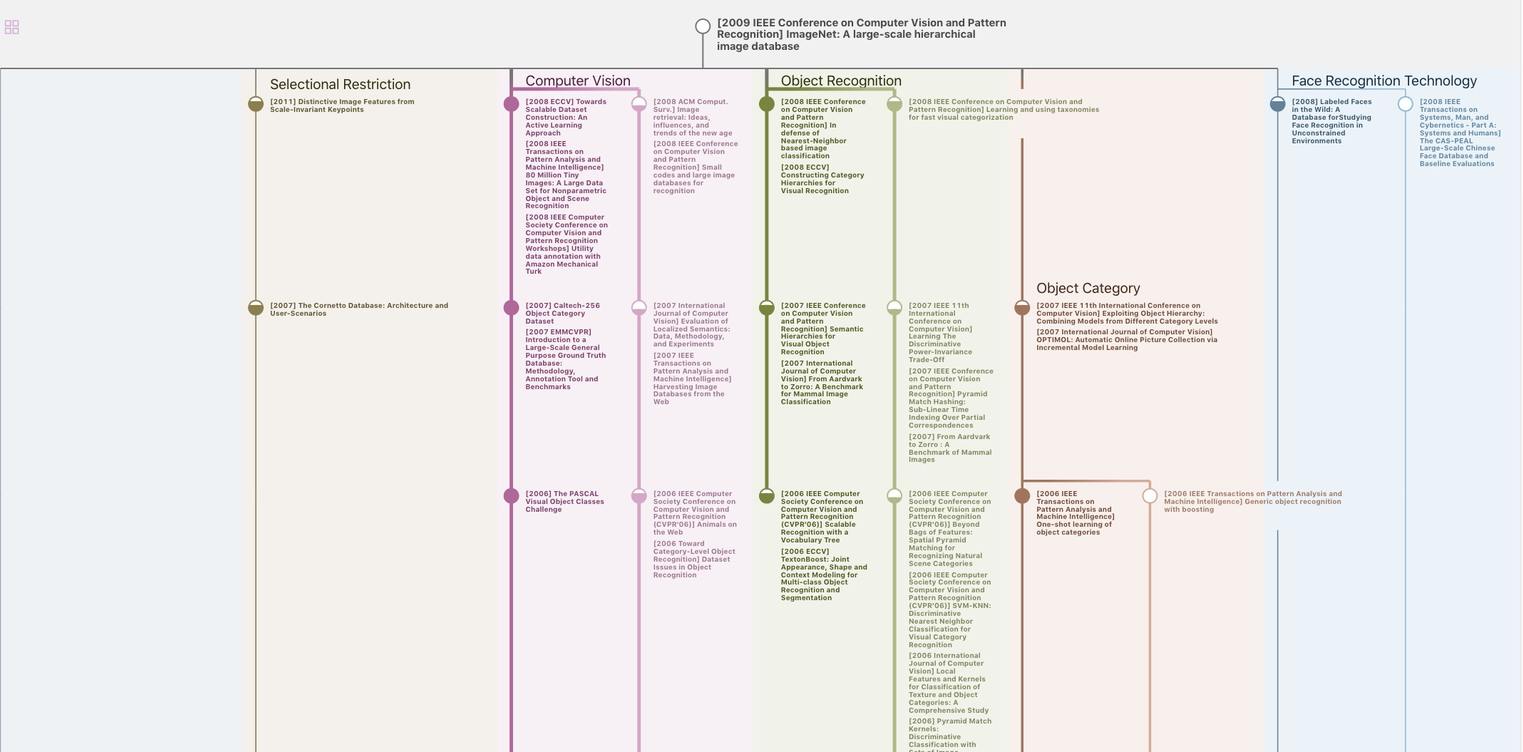
生成溯源树,研究论文发展脉络
Chat Paper
正在生成论文摘要