UAV Hyperspectral Characterization of Vegetation Using Entropy-Based Active Sampling for Partial Least Square Regression Models
APPLIED SCIENCES-BASEL(2023)
摘要
Optimization of agricultural practices is key for facing the challenges of modern agri-food systems, which are expected to satisfy a growing demand of food production in a landscape characterized by a reduction in cultivable lands and an increasing awareness of sustainability issues. In this work, an operational methodology for characterization of vegetation biomass and nitrogen content based on close-range hyperspectral remote sensing is introduced. It is based on an unsupervised active learning technique suitable for the calibration of a partial least square regression. The proposed technique relies on an innovative usage of Shannon's entropy and allows for the set-up of an incremental monitoring framework from scratch aiming at minimizing field sampling activities. Experimental results concerning the estimation of grassland biomass and nitrogen content returned RMSE values of 2.05 t/ha and 4.68 kg/ha, respectively. They are comparable with the literature, mostly relying on supervised frameworks and confirmed the suitability of the proposed methodology with operational environments.
更多查看译文
关键词
UAV,precision agriculture,hyperspectral imagery,active sampling,vegetation biomass,vegetation nitrogen content,partial least squares regression
AI 理解论文
溯源树
样例
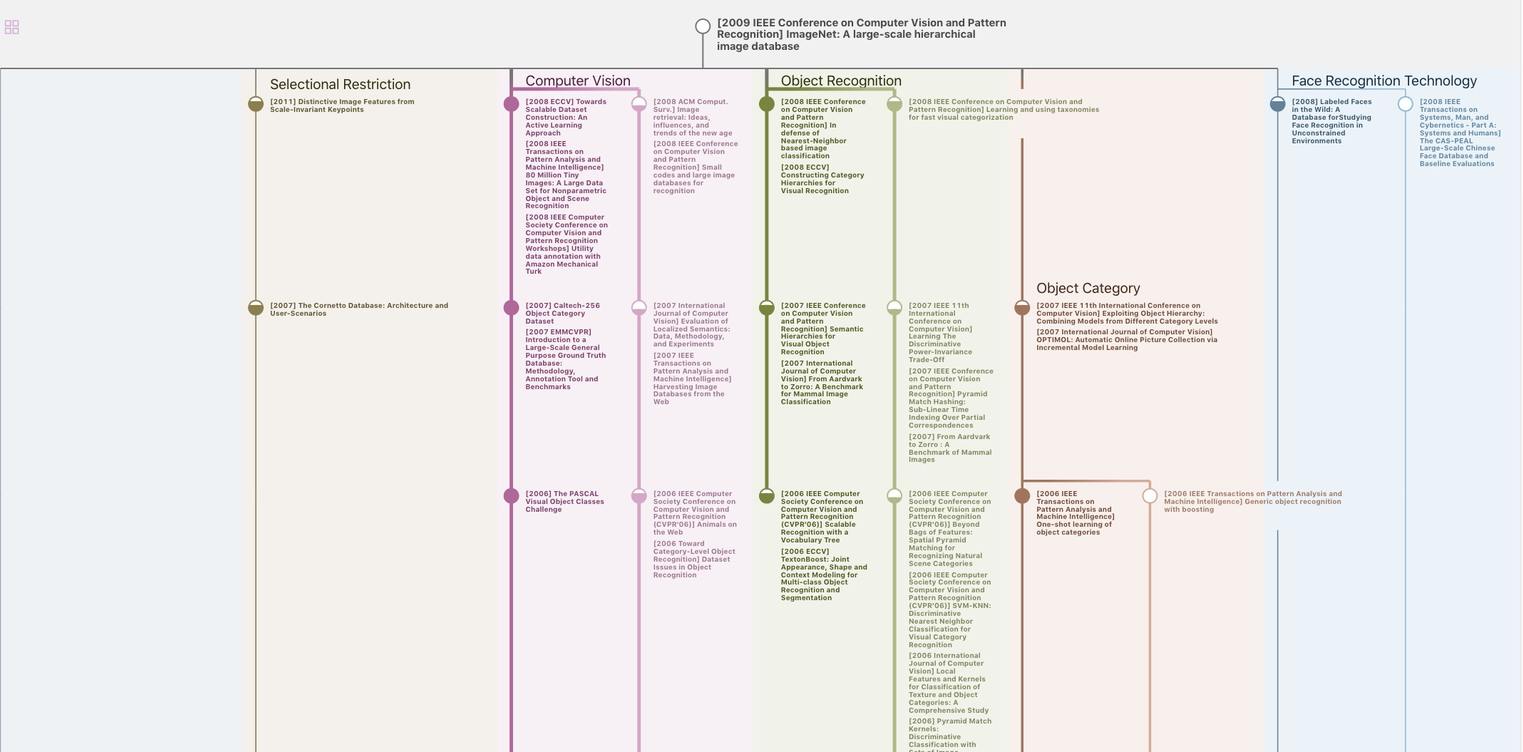
生成溯源树,研究论文发展脉络
Chat Paper
正在生成论文摘要