Gibbs sampling the posterior of neural networks
JOURNAL OF PHYSICS A-MATHEMATICAL AND THEORETICAL(2024)
摘要
In this paper, we study sampling from a posterior derived from a neural network. We propose a new probabilistic model consisting of adding noise at every pre- and post-activation in the network, arguing that the resulting posterior can be sampled using an efficient Gibbs sampler. For small models, the Gibbs sampler attains similar performances as the state-of-the-art Markov chain Monte Carlo methods, such as the Hamiltonian Monte Carlo or the Metropolis adjusted Langevin algorithm, both on real and synthetic data. By framing our analysis in the teacher-student setting, we introduce a thermalization criterion that allows us to detect when an algorithm, when run on data with synthetic labels, fails to sample from the posterior. The criterion is based on the fact that in the teacher-student setting we can initialize an algorithm directly at equilibrium.
更多查看译文
关键词
MCMC,Bayesian learning,neural networks,sampling algorithms,MCMC thermalization,statistical physics
AI 理解论文
溯源树
样例
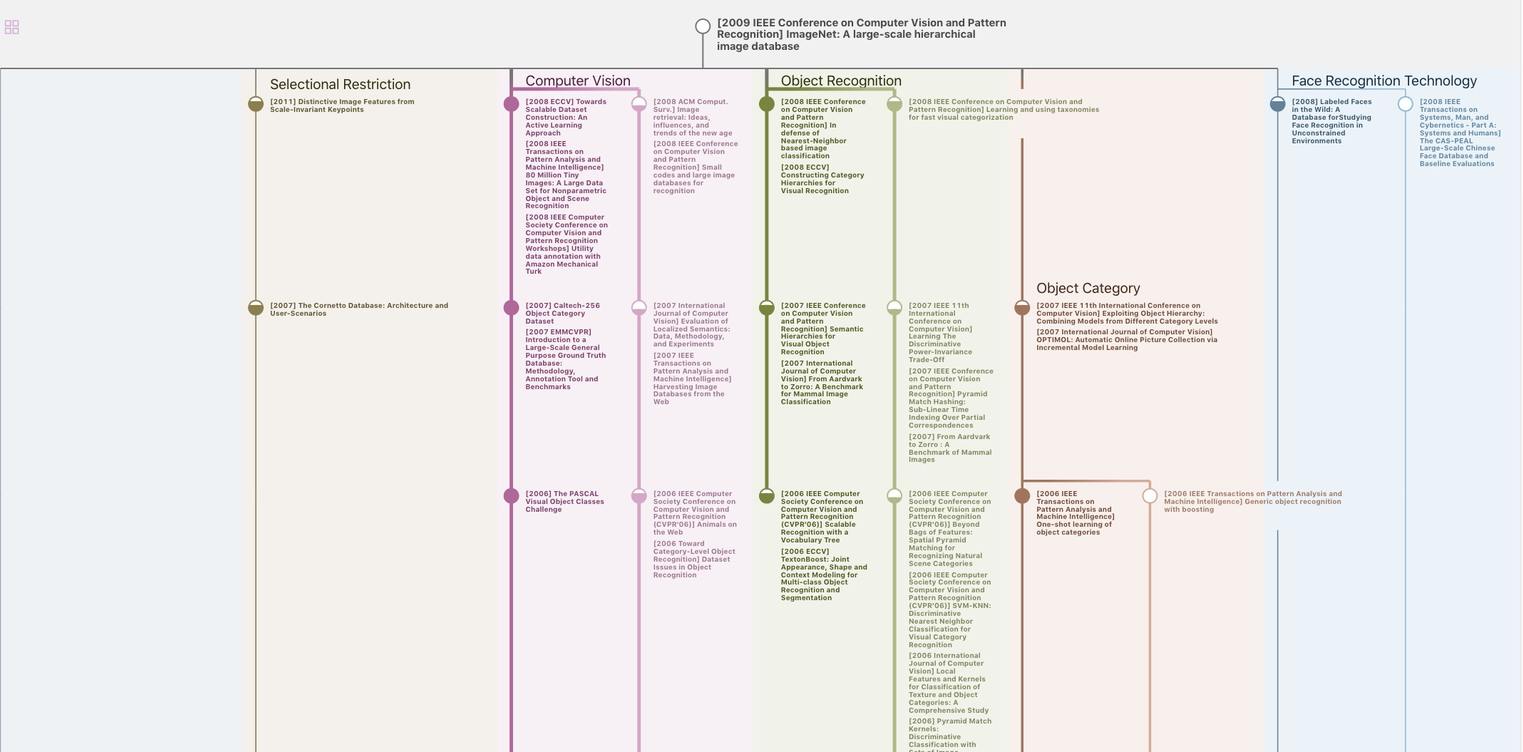
生成溯源树,研究论文发展脉络
Chat Paper
正在生成论文摘要