A Simple Yet High-Performing On-disk Learned Index: Can We Have Our Cake and Eat it Too?
CoRR(2023)
摘要
While in-memory learned indexes have shown promising performance as compared to B+-tree, most widely used databases in real applications still rely on disk-based operations. Based on our experiments, we observe that directly applying the existing learned indexes on disk suffers from several drawbacks and cannot outperform a standard B+-tree in most cases. Therefore, in this work we make the first attempt to show how the idea of learned index can benefit the on-disk index by proposing AULID, a fully on-disk updatable learned index that can achieve state-of-the-art performance across multiple workload types. The AULID approach combines the benefits from both traditional indexing techniques and the learned indexes to reduce the I/O cost, the main overhead under disk setting. Specifically, three aspects are taken into consideration in reducing I/O costs: (1) reduce the overhead in updating the index structure; (2) induce shorter paths from root to leaf node; (3) achieve better locality to minimize the number of block reads required to complete a scan. Five principles are proposed to guide the design of AULID which shows remarkable performance gains and meanwhile is easy to implement. Our evaluation shows that AULID has comparable storage costs to a B+-tree and is much smaller than other learned indexes, and AULID is up to 2.11x, 8.63x, 1.72x, 5.51x, and 8.02x more efficient than FITing-tree, PGM, B+-tree, ALEX, and LIPP.
更多查看译文
关键词
index,high-performing,on-disk
AI 理解论文
溯源树
样例
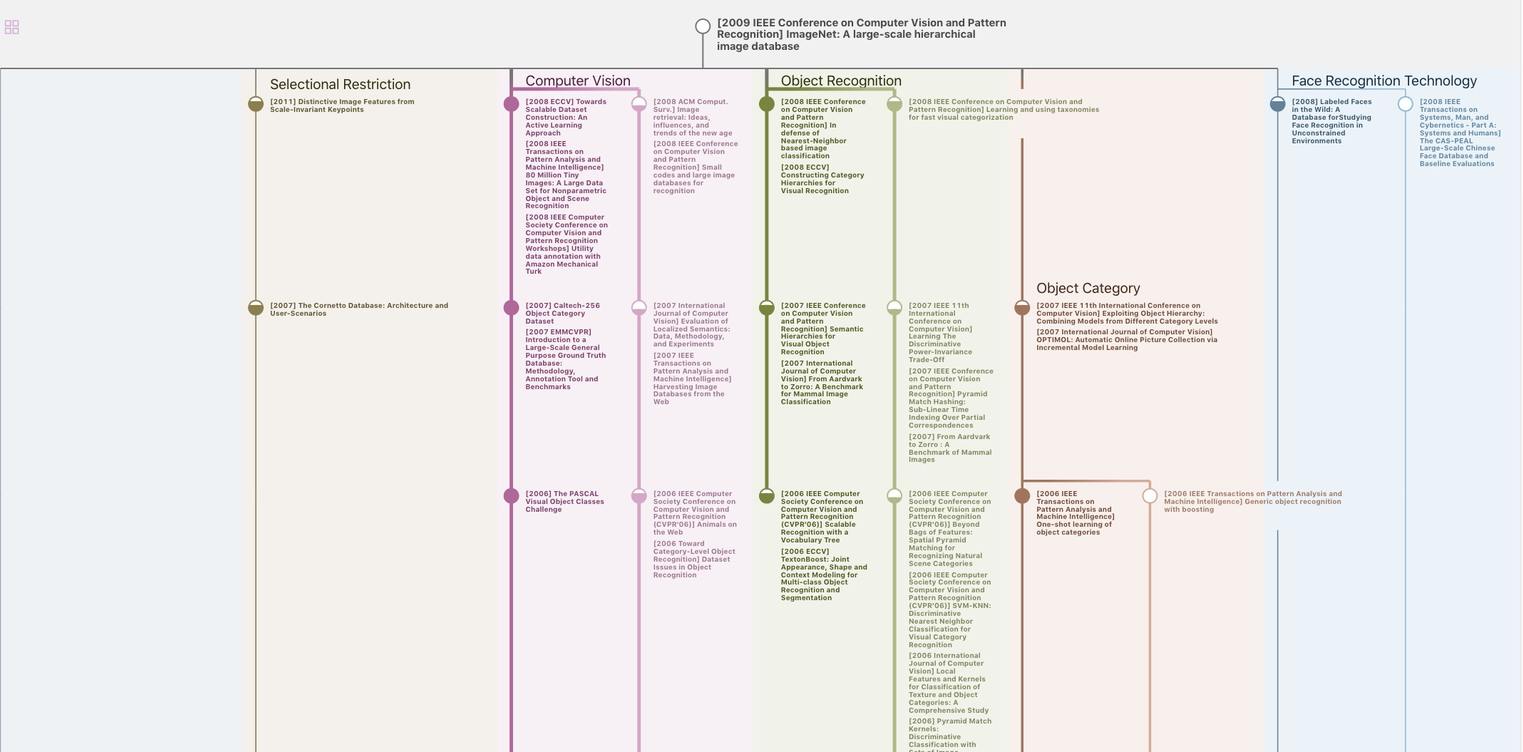
生成溯源树,研究论文发展脉络
Chat Paper
正在生成论文摘要