DAGrid: Directed Accumulator Grid
CoRR(2023)
摘要
Recent research highlights that the Directed Accumulator (DA), through its parametrization of geometric priors into neural networks, has notably improved the performance of medical image recognition, particularly with small and imbalanced datasets. However, DA's potential in pixel-wise dense predictions is unexplored. To bridge this gap, we present the Directed Accumulator Grid (DAGrid), which allows geometric-preserving filtering in neural networks, thus broadening the scope of DA's applications to include pixel-level dense prediction tasks. DAGrid utilizes homogeneous data types in conjunction with designed sampling grids to construct geometrically transformed representations, retaining intricate geometric information and promoting long-range information propagation within the neural networks. Contrary to its symmetric counterpart, grid sampling, which might lose information in the sampling process, DAGrid aggregates all pixels, ensuring a comprehensive representation in the transformed space. The parallelization of DAGrid on modern GPUs is facilitated using CUDA programming, and also back propagation is enabled for deep neural network training. Empirical results show DAGrid-enhanced neural networks excel in supervised skin lesion segmentation and unsupervised cardiac image registration. Specifically, the network incorporating DAGrid has realized a 70.8% reduction in network parameter size and a 96.8% decrease in FLOPs, while concurrently improving the Dice score for skin lesion segmentation by 1.0% compared to state-of-the-art transformers. Furthermore, it has achieved improvements of 4.4% and 8.2% in the average Dice score and Dice score of the left ventricular mass, respectively, indicating an increase in registration accuracy for cardiac images. The source code is available at https://github.com/tinymilky/DeDA.
更多查看译文
关键词
dagrid
AI 理解论文
溯源树
样例
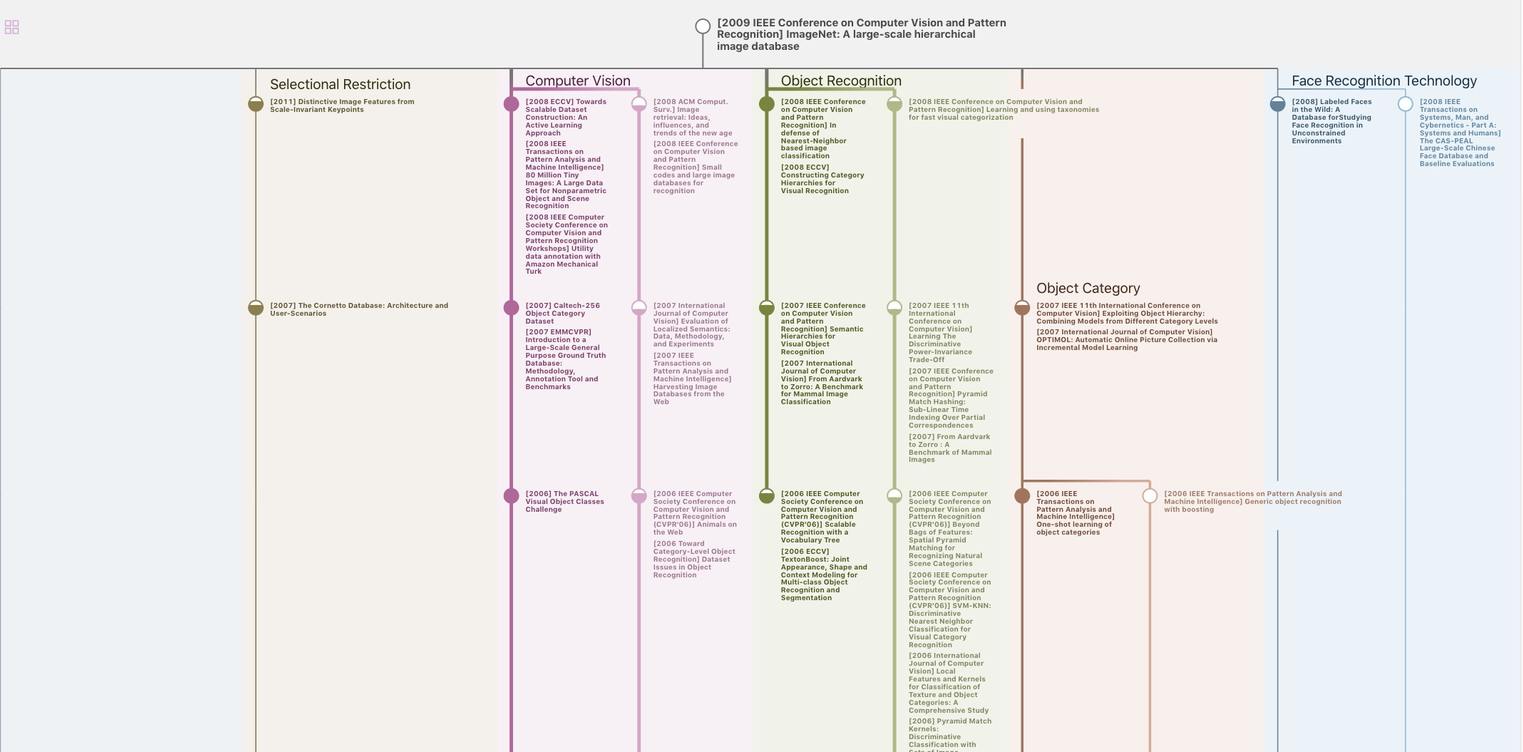
生成溯源树,研究论文发展脉络
Chat Paper
正在生成论文摘要