Cycle Consistency Driven Object Discovery
arXiv (Cornell University)(2023)
摘要
Developing deep learning models that effectively learn object-centric representations, akin to human cognition, remains a challenging task. Existing approaches have explored slot-based methods utilizing architectural priors or auxiliary information such as depth maps or flow maps to facilitate object discovery by representing objects as fixed-size vectors, called ``slots'' or ``object files''. However, reliance on architectural priors introduces unreliability and requires meticulous engineering to identify the correct objects. Likewise, methods relying on auxiliary information are suboptimal as such information is often unavailable for most natural scenes. To address these limitations, we propose a method that explicitly optimizes the constraint that each object in a scene should be mapped to a distinct slot. We formalize this constraint by introducing consistency objectives which are cyclic in nature. We refer to them as the \textit{cycle-consistency} objectives. By applying these consistency objectives to various existing slot-based object-centric methods, we demonstrate significant enhancements in object-discovery performance. These improvements are consistent across both synthetic and real-world scenes, highlighting the effectiveness and generalizability of the proposed approach. Furthermore, our experiments show that the learned slots from the proposed method exhibit superior suitability for downstream reinforcement learning (RL) tasks.
更多查看译文
关键词
discovery,consistency,object
AI 理解论文
溯源树
样例
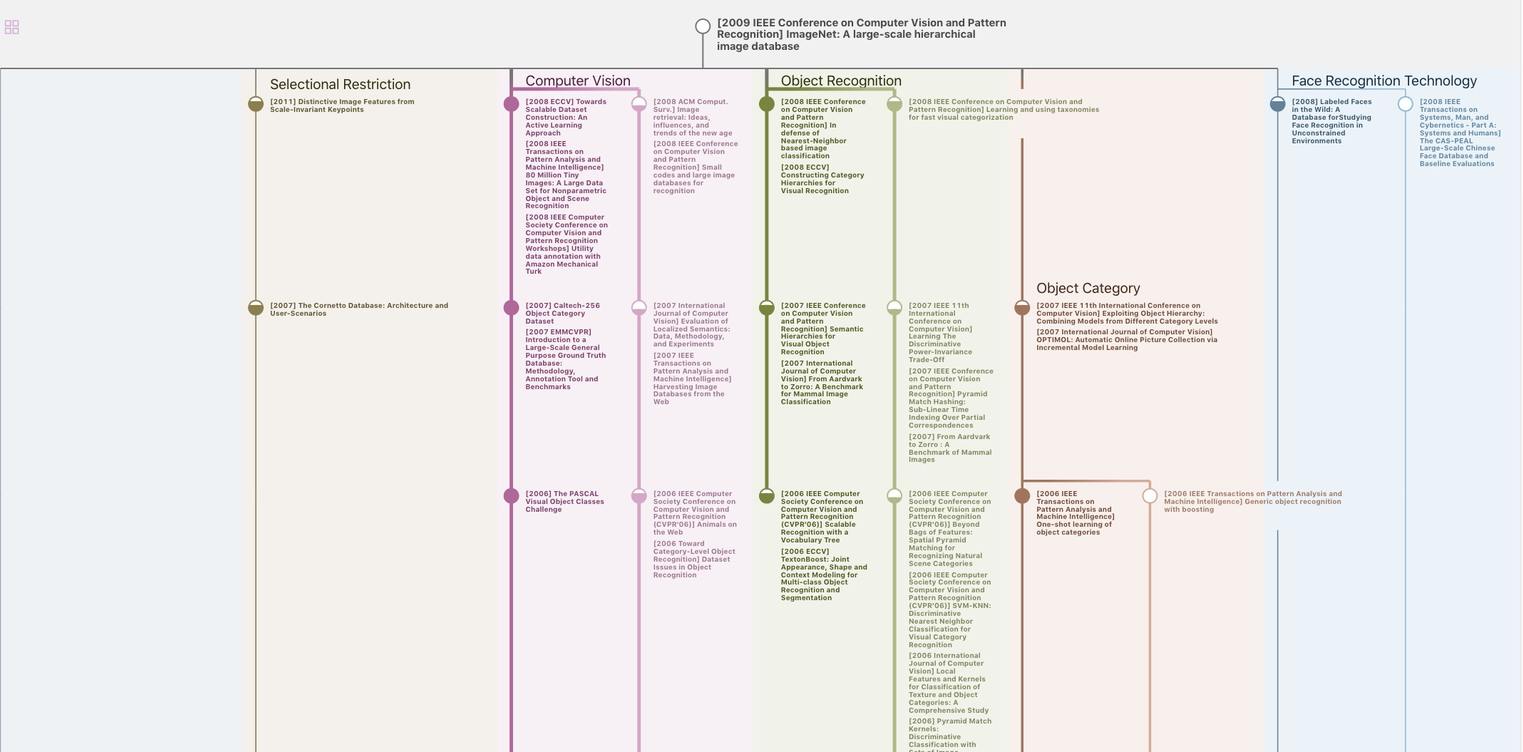
生成溯源树,研究论文发展脉络
Chat Paper
正在生成论文摘要