GAD-NR: Graph Anomaly Detection via Neighborhood Reconstruction
PROCEEDINGS OF THE 17TH ACM INTERNATIONAL CONFERENCE ON WEB SEARCH AND DATA MINING, WSDM 2024(2024)
摘要
Graph Anomaly Detection (GAD) is a technique used to identify abnormal nodes within graphs, finding applications in network security, fraud detection, social media spam detection, and various other domains. A common method for GAD is Graph AutoEncoders (GAEs), which encode graph data into node representations and identify anomalies by assessing the reconstruction quality of the graphs based on these representations. However, existing GAE models are primarily optimized for direct link reconstruction, resulting in nodes connected in the graph being clustered in the latent space. As a result, they excel at detecting cluster-type structural anomalies but struggle with more complex structural anomalies that do not conform to clusters. To address this limitation, we propose a novel solution called GAD-NR, a new variant of GAE that incorporates neighborhood reconstruction for graph anomaly detection. GAD-NR aims to reconstruct the entire neighborhood of a node, encompassing the local structure, self-attributes, and neighbor attributes, based on the corresponding node representation. By comparing the neighborhood reconstruction loss between anomalous nodes and normal nodes, GAD-NR can effectively detect any anomalies. Extensive experimentation conducted on six real-world datasets validates the effectiveness of GAD-NR, showcasing significant improvements (by up to 30%. in AUC) over state-of-the-art competitors. The source code for GAD-NR is openly available. Importantly, the comparative analysis reveals that the existing methods perform well only in detecting one or two types of anomalies out of the three types studied. In contrast, GAD-NR excels at detecting all three types of anomalies across the datasets, demonstrating its comprehensive anomaly detection capabilities.
更多查看译文
关键词
Anomaly Detection,Graph Neural Network,Auto-Encoder
AI 理解论文
溯源树
样例
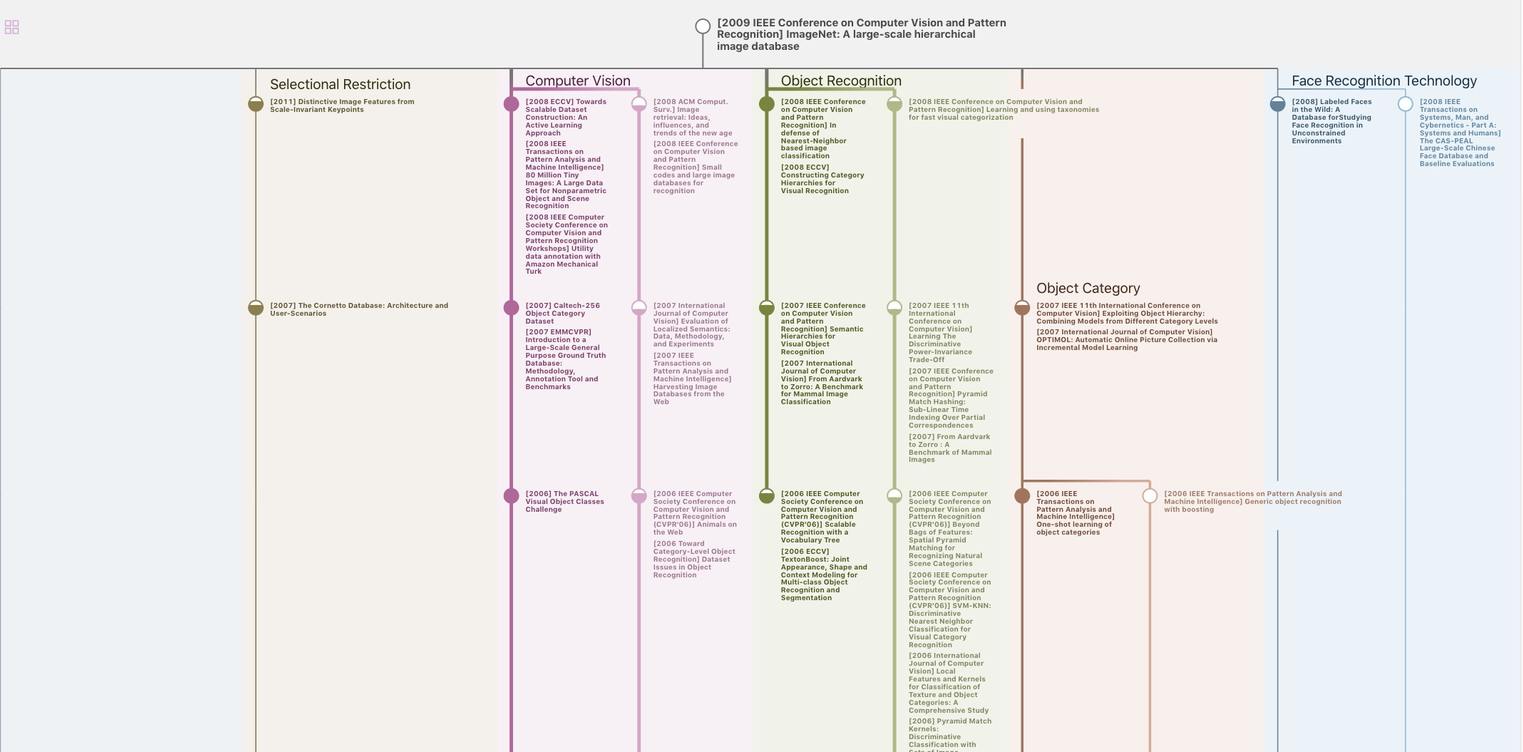
生成溯源树,研究论文发展脉络
Chat Paper
正在生成论文摘要