No Bidding, No Regret: Pairwise-Feedback Mechanisms for Digital Goods and Data Auctions
CoRR(2023)
摘要
The growing demand for data and AI-generated digital goods, such as personalized written content and artwork, necessitates effective pricing and feedback mechanisms that account for uncertain utility and costly production. Motivated by these developments, this study presents a novel mechanism design addressing a general repeated-auction setting where the utility derived from a sold good is revealed post-sale. The mechanism's novelty lies in using pairwise comparisons for eliciting information from the bidder, arguably easier for humans than assigning a numerical value. Our mechanism chooses allocations using an epsilon-greedy strategy and relies on pairwise comparisons between realized utility from allocated goods and an arbitrary value, avoiding the learning-to-bid problem explored in previous work. We prove this mechanism to be asymptotically truthful, individually rational, and welfare and revenue maximizing. The mechanism's relevance is broad, applying to any setting with made-to-order goods of variable quality. Experimental results on multi-label toxicity annotation data, an example of negative utilities, highlight how our proposed mechanism could enhance social welfare in data auctions. Overall, our focus on human factors contributes to the development of more human-aware and efficient mechanism design.
更多查看译文
关键词
bidding,digital goods
AI 理解论文
溯源树
样例
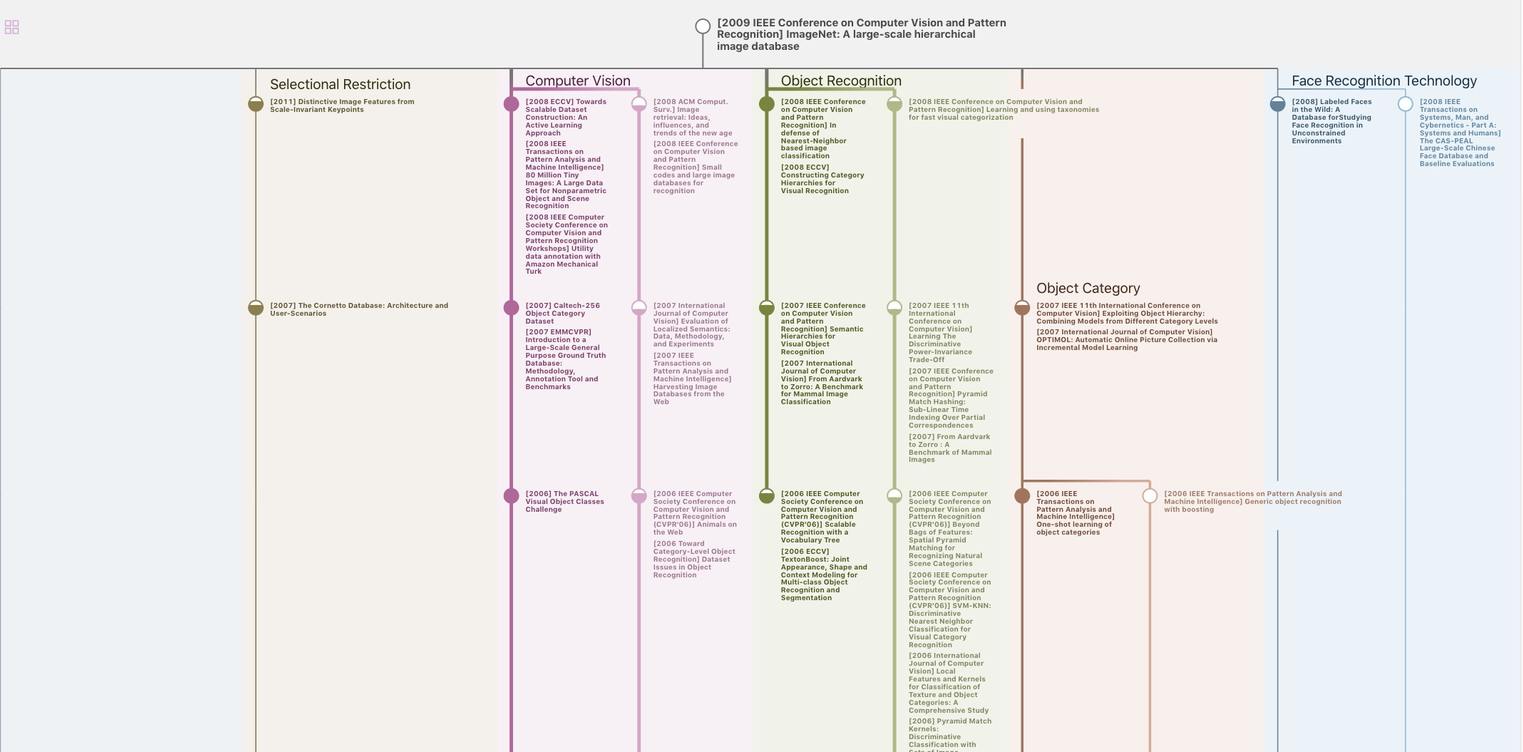
生成溯源树,研究论文发展脉络
Chat Paper
正在生成论文摘要